Classification-Aided High-Quality PET Image Synthesis via Bidirectional Contrastive GAN with Shared Information Maximization
Medical Image Computing and Computer Assisted Intervention – MICCAI 2022(2022)
摘要
Positron emission tomography (PET) is a pervasively adopted nuclear imaging technique, however, its inherent tracer radiation inevitably causes potential health hazards to patients. To obtain high-quality PET image while reducing radiation exposure, this paper proposes an algorithm for high-quality standard-dose PET (SPET) synthesis from low-dose PET (LPET) image. Specifically, considering that LPET images and SPET images come from the same subjects, we argue that there is abundant shared content and structural information between LPET and SPET domains, which is helpful for improving synthesis performance. To this end, we innovatively propose a bi-directional contrastive generative adversarial network (BiC-GAN), containing a master network and an auxiliary network. Both networks implement intra-domain reconstruction and inter-domain synthesis tasks, aiming to extract shared information from LPET and SPET domains, respectively. Meanwhile, the contrastive learning strategy is also introduced to two networks for enhancing feature representation capability and acquiring more domain-independent information. To maximize the shared information extracted from two domains, we further design a domain alignment module to constrain the consistency of the shared information extracted from the two domains. On the other hand, since synthesized PET images can be used to assist disease diagnosis, such as mild cognitive impairment (MCI) identification, the MCI classification task is incorporated into PET image synthesis to further improve clinical applicability of the synthesized PET image through direct feedback from the classification task. Evaluated on a Real Human Brain dataset, our proposed method is demonstrated to achieve state-of-the-art performance quantitatively and qualitatively.
更多查看译文
关键词
Positron emission tomography (PET), Contrastive learning, Shared information, Generative adversarial network (GAN), Image synthesis
AI 理解论文
溯源树
样例
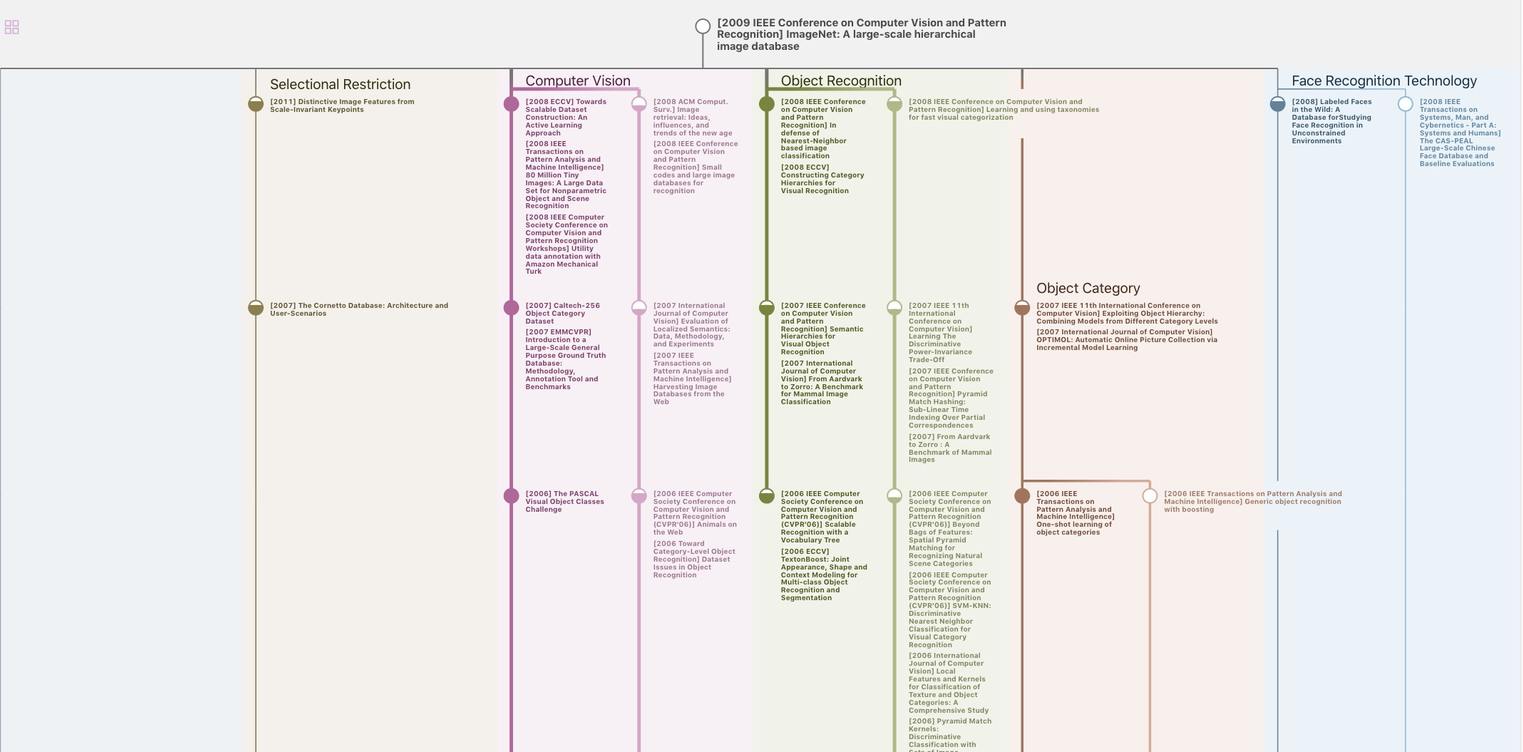
生成溯源树,研究论文发展脉络
Chat Paper
正在生成论文摘要