An Ensemble Model for Network Intrusion Detection Using AdaBoost, Random Forest and Logistic Regression
Applications of Artificial Intelligence and Machine Learning(2022)
摘要
Due to the extension of network-based services, the requirement of network security has increased than ever. Although, various technologies like cryptography, steganography, firewalls, and intrusion systems have been introduced to apply security on the information and network services. The Intrusion detection system has become a prevalent technique to sense the network environment, but sometimes these systems are unable to detect novel attacks. So, to achieve higher accuracy and lower false alarm various techniques and models have proposed by researchers, but the crucial part of existing models is detection time, low accuracy, unable to detect a new attack and higher detection time. To address these issues, a novel intrusion detection framework with ensemble machine learning technique has proposed to provide the best accuracy for attack detection. Three base learning techniques AdaBoost Classifier, Random Forest Classifier and Logistic Regression, have used to integrate voting ensemble model. Experiments of the proposed model have been conducted on KDDCUP99 dataset for identifying the attacks by the intrusion detection system. Empirical results present refinement in detection accuracy for all the classes (Normal, Probe, DoS, U2R and R2L) of network traffic. Efficiency of this model has measured by Accuracy Score = 99.86%. As results shows, the proposed model works efficiently and effectively for a network intrusion detection system.
更多查看译文
关键词
Intrusion detection, Ensemble learning, Machine learning, Random forest classifier, AdaBoost classifier, Logistic regressor, Network analysis
AI 理解论文
溯源树
样例
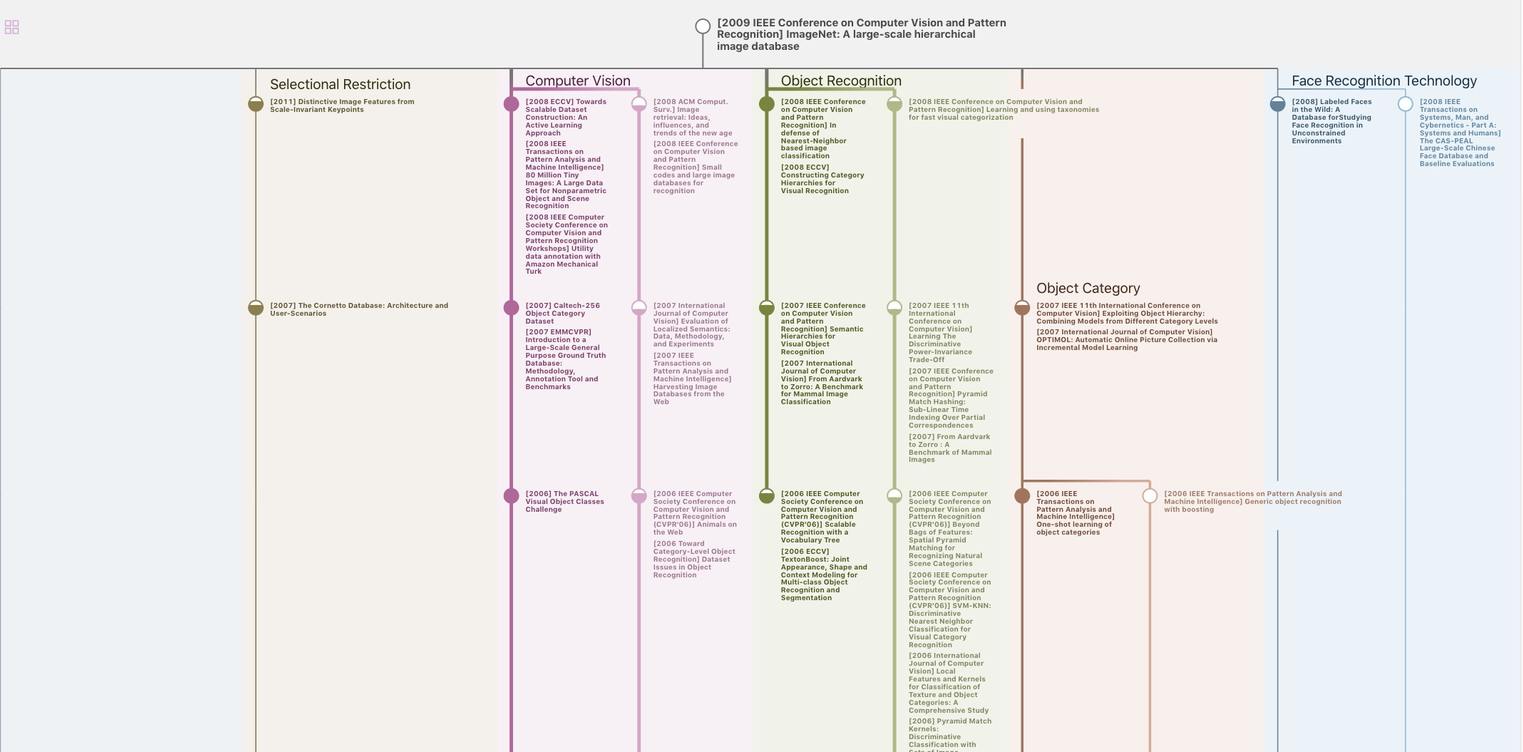
生成溯源树,研究论文发展脉络
Chat Paper
正在生成论文摘要