Wrist Ultrasound Segmentation by Deep Learning
Artificial Intelligence in Medicine(2022)
摘要
Ultrasound (US) is an increasingly popular medical imaging modality in clinical practice due to its low cost, portability, and real-time dynamic display. It is ideally suited for wrist and elbow fracture detection in children as it does not involve any ionizing radiation. Automatic assessment of wrist images requires delineation of relevant bony structures seen in the image including the radial epiphysis, radial metaphysis and carpal bones. With the advent of artificial intelligence, researchers are using deep learning models for segmentation in US scans including these to help with automatic diagnosis and disease progression. However, certain specific characteristics of US such as poor signal to noise ratio, presence of imaging artifacts and blurred boundaries around anatomical structures make segmentation challenging. In this research, we applied deep learning models including UNet and Generative Adversarial Network (GAN) to segment bony structures from a wrist US scan. Our ensemble models were trained on wrist 3D US datasets containing 10,500 images in 47 patients acquired from the University of Alberta Hospital (UAH) pediatric emergency department using a Philips iU22 ultrasound scanner. In general, although UNet gave the highest DICE score, precision and Jaccard Index, GAN achieved the highest recall. Our study shows the feasibility of using deep learning techniques for automatically segmenting bony regions from a wrist US image which could lead to automatic detection of fractures in pediatric emergencies.
Github
.
更多查看译文
关键词
Wrist ultrasound, Image segmentation, Deep learning, UNet, GAN, Pix2pix
AI 理解论文
溯源树
样例
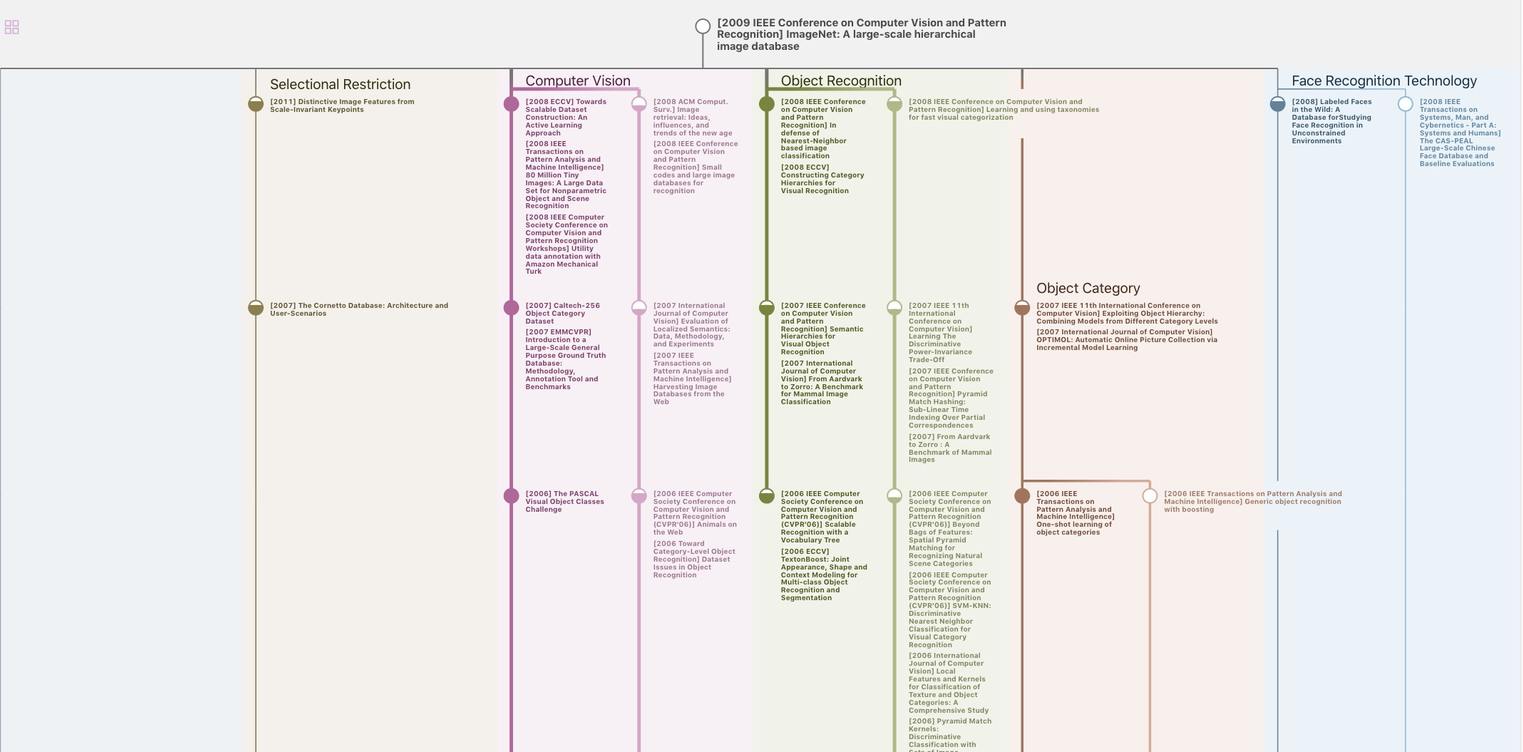
生成溯源树,研究论文发展脉络
Chat Paper
正在生成论文摘要