Comparing Time and Frequency Domain Heart Rate Variability for Deep Learning-Based Glucose Detection
ICT Innovations 2021. Digital Transformation(2022)
摘要
Many researchers have been challenged by the usage of devices related to the Internet of Things in conjunction with machine learning to anticipate and diagnose health issues. Diabetes has always been a problem that society has struggled with. Due to the simplicity with which electrocardiograms can be captured and analysed, deep learning can be used to forecast a patient’s instantaneous glucose levels. Our solution is based on a unique method for calculating heart rate variability that involves segment identification, averaging, and concatenating the data to reveal better feature engineering results. Immediate plasma glucose levels are detected using short-term heart rate variability and applied a deep learning method based on Autokeras. In this paper. we address a research question to compare the predictive capability of time and frequency domain features for instantaneous glucose values. The neural architectural search for the time domain approach provided the best results for the 15-min electrocardiogram measurements. Similarly, the Frequency Domain approach showed better results on the same time frame. Regarding the time domain the best results are as follows: RMSE (0.368), MSE (0.193), R
$$^2$$
(0.513), and R
$$^2$$
loss (0.541). The best results for the Frequency Domain approach the best results are as follows: RMSE (0.301), MSE (0.346), R
$$^2$$
(0.45578), and R
$$^2$$
loss (0.482).
更多查看译文
关键词
ECG, HRV, Deep learning, Glucose, Diabetes
AI 理解论文
溯源树
样例
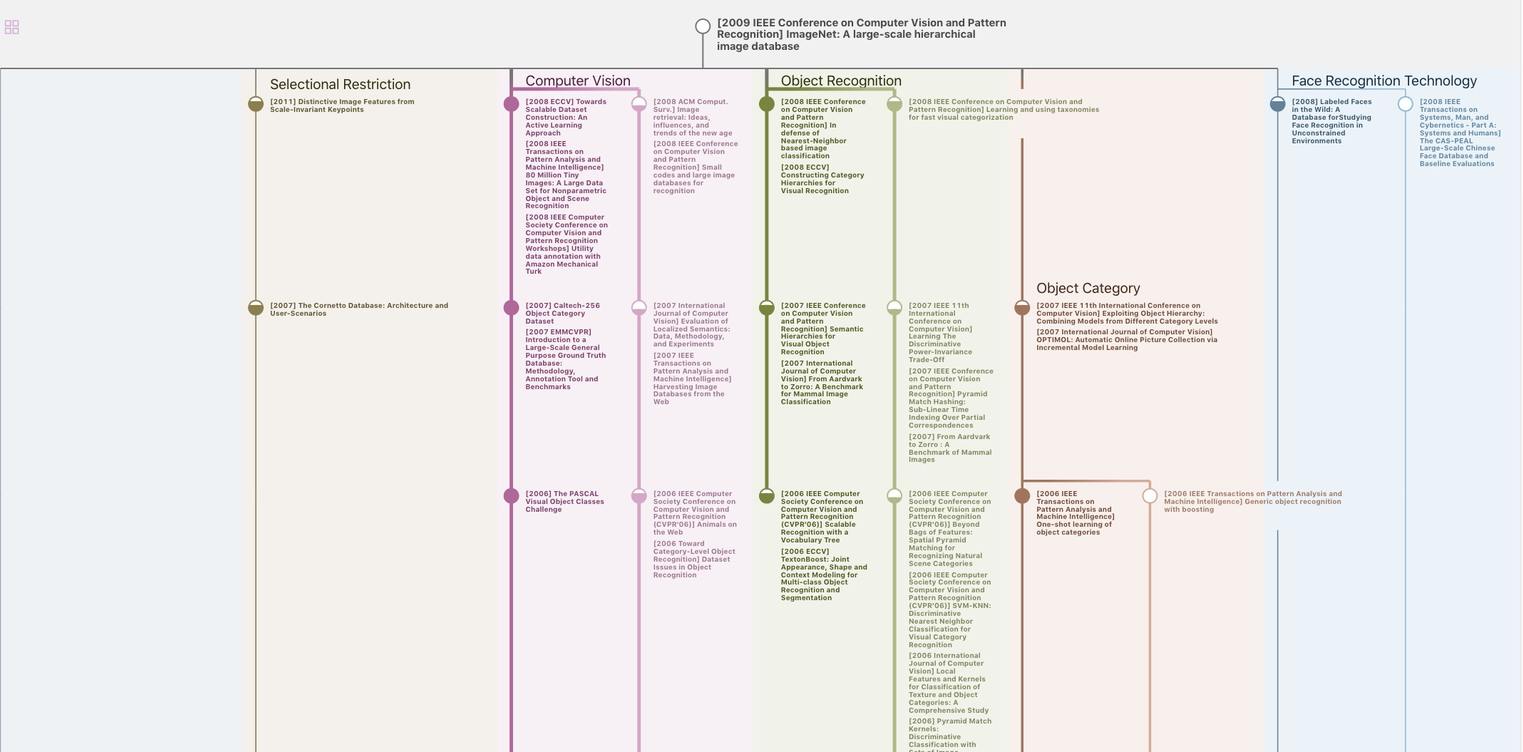
生成溯源树,研究论文发展脉络
Chat Paper
正在生成论文摘要