Attention-Fused Deep Relevancy Matching Network for Clickbait Detection
IEEE Transactions on Computational Social Systems(2023)
摘要
Clickbait is a sort of news that contains low-quality content but intriguing or overstated titles. Most of the existing studies usually utilize titles to detect clickbait and suffer from poor performance. However, from the perspective of user cognition, the essence of clickbait news is due to the fact that the body contents fall short of the titles’ expectations. So, in addition to the explicit features of titles, many extra informative signals could help to distinguish clickbait. In this article, we proposed a novel Multiview learning approach-based ClickBait Detection (MCBD) model that takes into account useful signals from both titles and body contents. From the view of titles, a multilayer gated convolutional network is employed to learn the local- and long-distance dependence relationships between words. From the view of body contents, we first extract the key topic sentences from the body content and then we developed a novel attention-fused deep relevance matching network (ARMN) to thoroughly mine the similarity between titles and body contents. Combined with the information from the views of titles and body contents, our model can distinguish well-hidden clickbait news. Finally, extensive experiments were conducted on two real-world datasets to demonstrate that the MCBD model outperforms the state-of-the-art models, improving the final performance by 3% in terms of the F1-score. The ablation studies demonstrate that the relevancy between titles and body contents can improve the model’s performance by 1.7% on the F1-score.
更多查看译文
关键词
Feature extraction,Data mining,Neural networks,Deep learning,Semantics,Noise measurement,Logic gates,Attention mechanism,clickbait detection,matching network,multiview learning
AI 理解论文
溯源树
样例
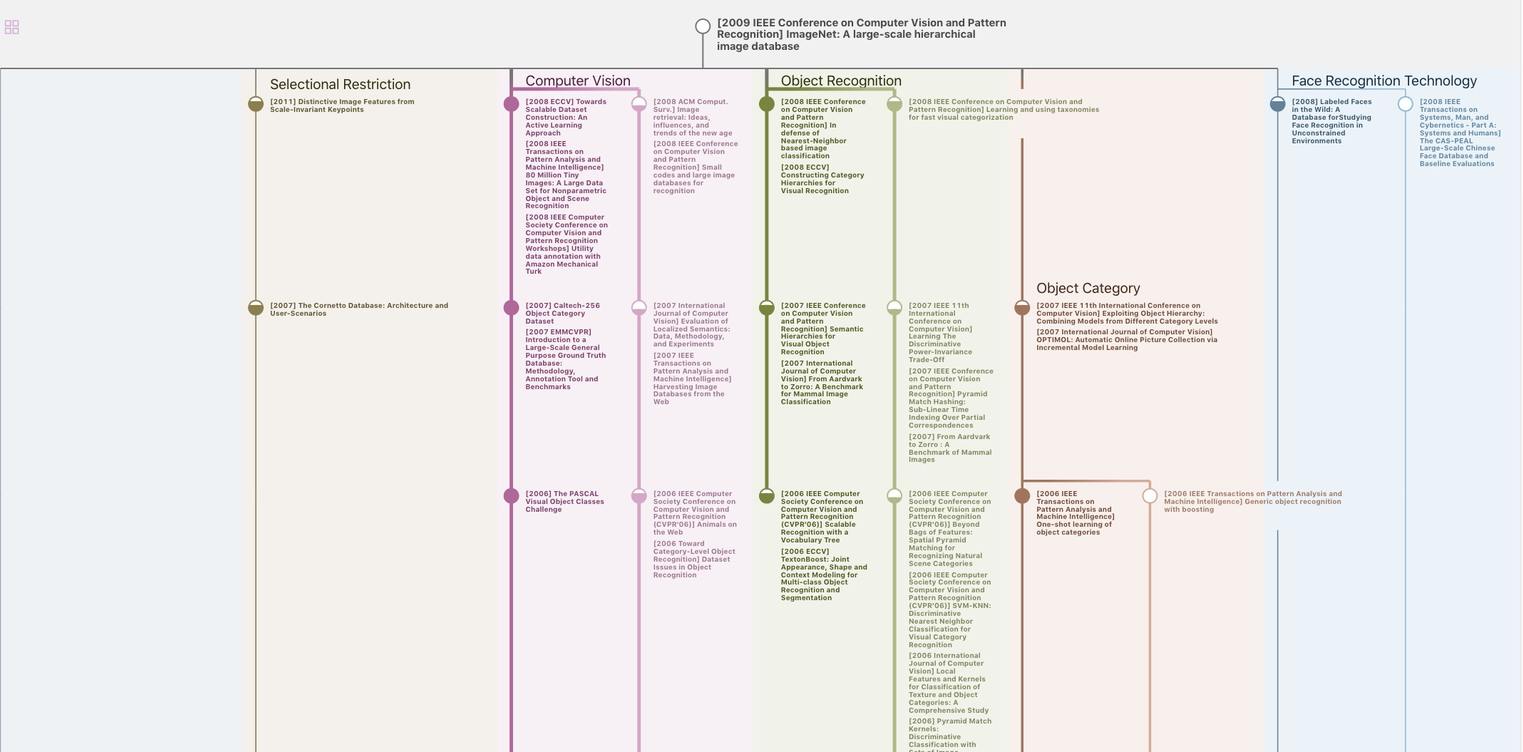
生成溯源树,研究论文发展脉络
Chat Paper
正在生成论文摘要