Tackling Over-Smoothing: Graph Hollow Convolution Network with Topological Layer Fusion
IEEE International Joint Conference on Neural Network (IJCNN)(2022)
摘要
In recent years, Graph Convolutional Networks (GCNs) have achieved great success in graph representations learning by incorporating features and topology information in each layer. Yet most GCNs are limited to the shallow network architecture due to over-smoothing which leads to indistinguishable nodes representations. In this paper, we provide a unique perspective on the key factor of over-smoothing which is the topological expressiveness loss when the stacked graph diffusion operators are over-densifying in deep layers. To tackle the over-smoothing issue, we propose the Graph Hollow Convolution Network (GHCN) with two key innovations. First, we design a hollow filter applied to the stacked graph diffusion operators to retain the topological expressiveness. Second, in order to further exploit the topology information, we integrate information from different layers based on graph local structures using topological layer fusion without propagating through nodes repeatedly. Extensive experiments on benchmark datasets show that our proposed method outperforms the state-of-the-art methods and effectively relieves the over-smoothing problem.
更多查看译文
关键词
Deep Learning,Graph Representation Learning,Graph Neural Networks
AI 理解论文
溯源树
样例
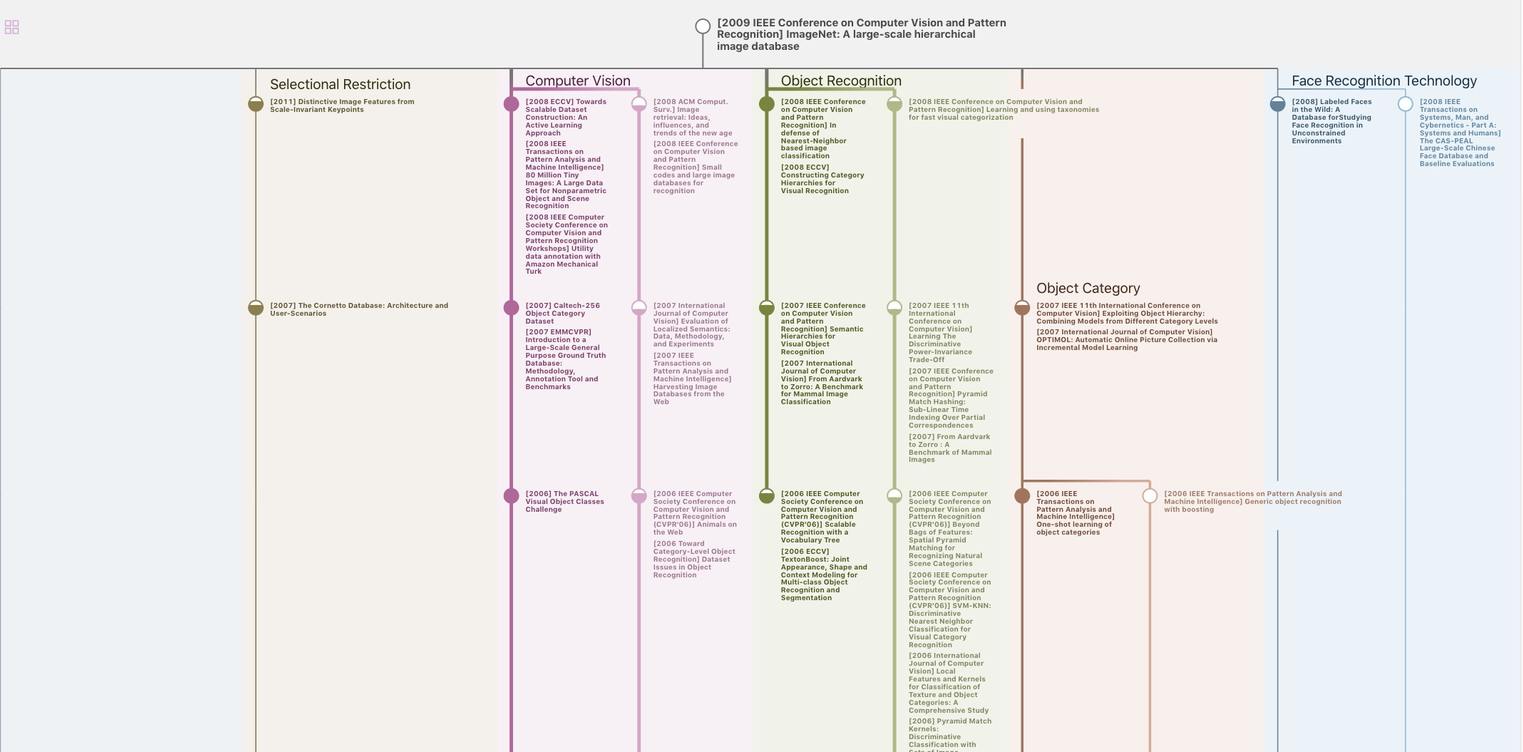
生成溯源树,研究论文发展脉络
Chat Paper
正在生成论文摘要