Improving the Semantic Consistency of Textual Adversarial Attacks via Prompt
IEEE International Joint Conference on Neural Network (IJCNN)(2022)
摘要
Adversarial examples can expose the vulnerabilities of neural networks. State-of-the-art textual adversarial attacks have demonstrated their effectiveness in triggering errors in the output of natural language processing models. However, these attacks are limited to ensuring the semantic consistency between the adversarial example and the original input, increasing the possibility of the attacks being detected by human judges. In this paper, we propose a novel textual adversarial attack, Prompt-Attack, which aims to generate the adversarial examples having consistent semantics with the original input. Specifically, Prompt-Attack enhances the input's semantics with the prompts that represent the semantics of the different target segments extracted from the original input, to predict the substitutions having consistent semantics with the target segments. Then, it crafts the adversarial examples by replacing the important segments with their substitutions that can most affect the victim model's output. Besides, Prompt-Attack proposes a span-level segment identification strategy to extract more target segments from the input and a novel masking strategy to ensure the grammatical correctness of the generated adversarial examples. Extensive experiments on public datasets illustrate that Prompt-Attack significantly improves the semantic consistency score of the baseline attacks by an average of 48%. Further, Prompt-Attack achieves the best attack success rate of 0.906, showing an average improvement of 40% to the baselines. Moreover, the experimental results demonstrate that Prompt-Attack can achieve good performance in attacking different language models and Prompt-Attack is not sensitive to different settings.
更多查看译文
关键词
Textual Adversarial Attack,Semantics,Prompt
AI 理解论文
溯源树
样例
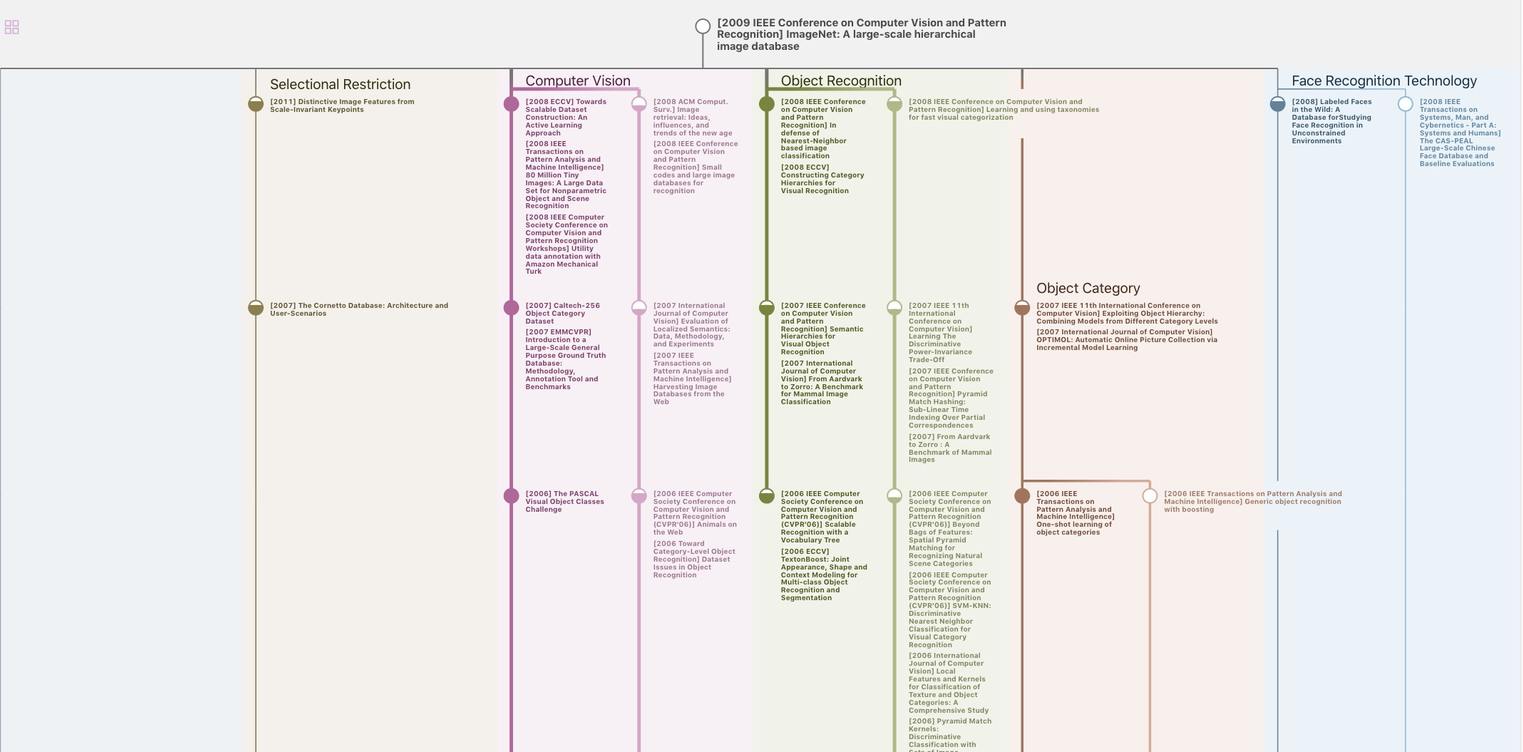
生成溯源树,研究论文发展脉络
Chat Paper
正在生成论文摘要