SILK-BPR: Identify Implicit Friends with Self-guided Walking for Social Recommendation
2022 International Joint Conference on Neural Networks (IJCNN)(2022)
摘要
With the increasing scale of data to be processed by the recommendation system and the rapid growth of the number of users and commodities, the problems of data sparsity and cold start become more and more severe. Many studies have found that introducing implicit social relationships into recommendation can alleviate the above problems. Among these studies, the method of identifying implicit friends for each user in heterogeneous information networks based on meta-paths achieves good performance. However, meta paths need to be preset artificially and require strong professional domain knowledge, which makes it impossible to capture semantic information accurately and effectively. In this paper, we propose a novel social recommendation model termed as SILK-BPR which is an extension of the Self Guided Walk(SILK) model to by bypass the meta-paths and identify implicit friends for recommendation. Specially, SILK-BPR first establishes a guidance matrix to record the transition probability from user nodes to interest nodes. And then conducts a self-guided walking on the matrix to mine the implicit friends which are classified into different types according to the appear times in different sequences. Finally, these implicit friends are integrated into an enhanced social Bayesian Personalized Ranking model for Top-N recommendation. Experimental results on real-world datasets demonstrate that SILK-BPR significantly outperforms state-of-the-art methods.
更多查看译文
关键词
Social Recommender Systems,Social Networks,Implicit friends,Heterogeneous Information Network
AI 理解论文
溯源树
样例
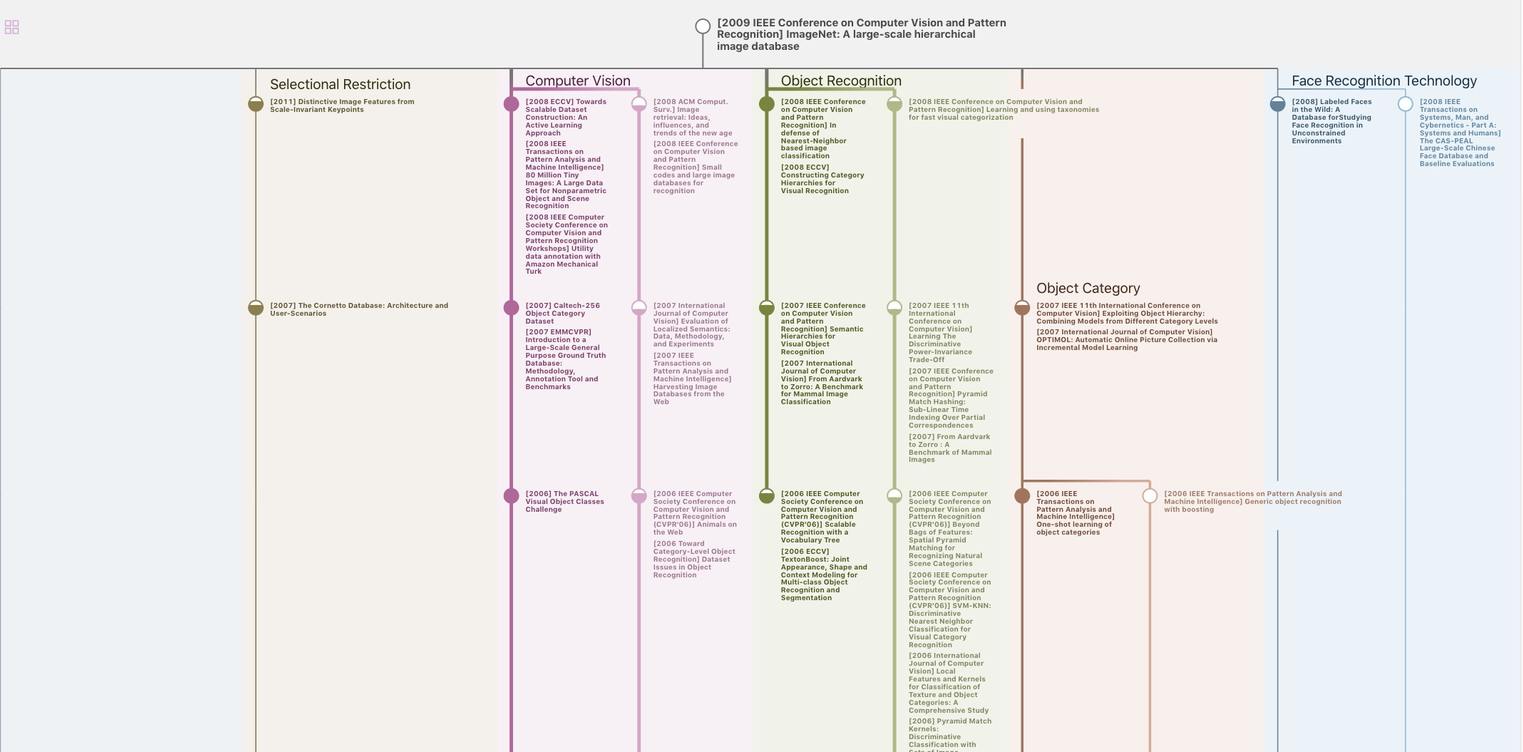
生成溯源树,研究论文发展脉络
Chat Paper
正在生成论文摘要