Hierarchical Cache Transformer: Dynamic Early Exit for Language Translation
IEEE International Joint Conference on Neural Network (IJCNN)(2022)
摘要
The transformer model significantly improves the performance of natural language processing tasks. However, the downside of employing the transformer-based model is its heavy inference cost, which raises the concern of putting transformer-based models into industrial operations. Thus, studies for improving inference performance were reported. However, the major studies mainly consider classification tasks but not for natural language generation (NLG). In this paper, we propose Hierarchical Cache (HC) Transformer model tailored to NLG tasks. Our experiments show the feasible results on German-English translation dataset. The experiment result demonstrates that HC-Transformer can speed up inference by 32% with a 3% loss in performance.
更多查看译文
关键词
Dynamical Early Exit,Hierarchical Decoding,NLG,Model Inference Performance
AI 理解论文
溯源树
样例
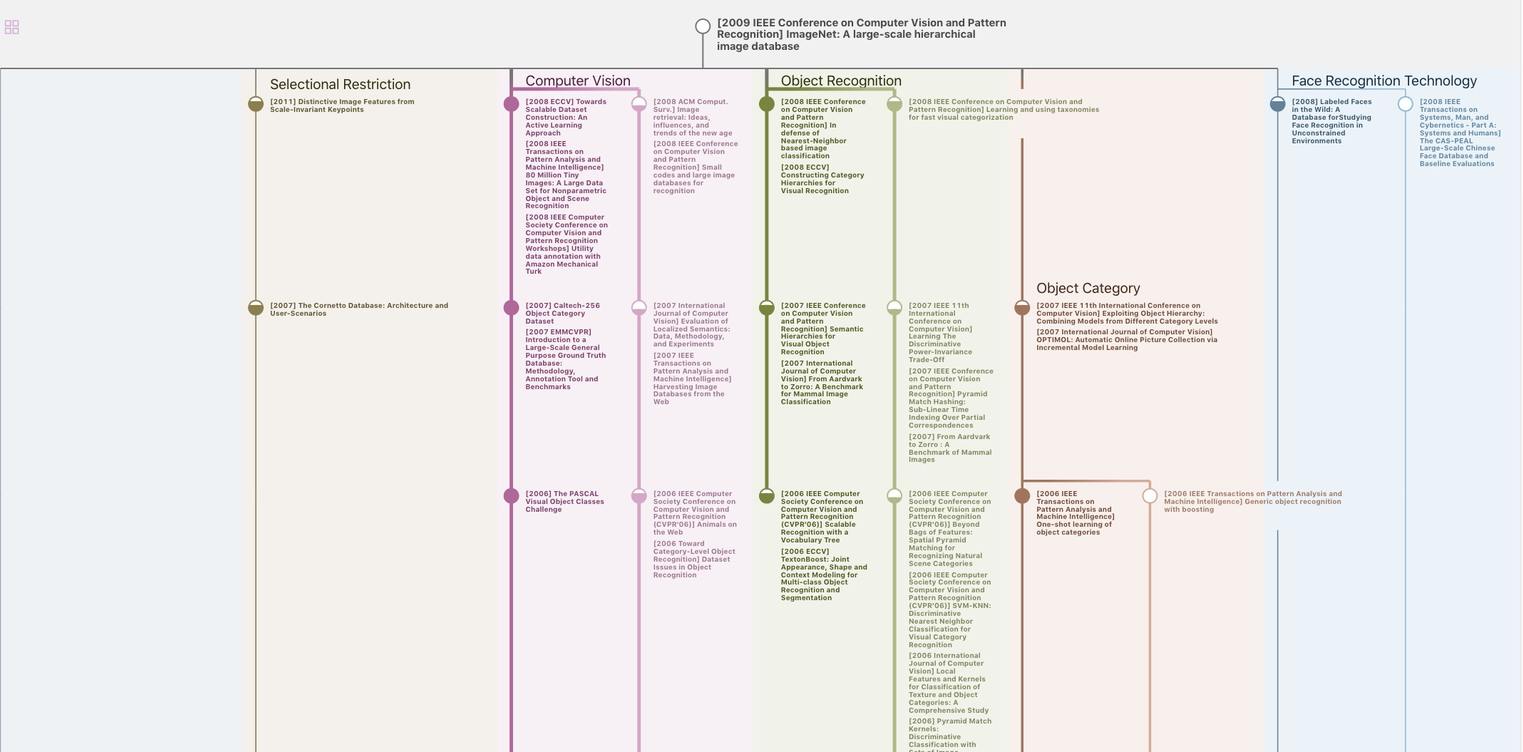
生成溯源树,研究论文发展脉络
Chat Paper
正在生成论文摘要