Self-Training Based Semi-Supervised and Semi-Paired Hashing Cross-Modal Retrieval
IEEE International Joint Conference on Neural Network (IJCNN)(2022)
摘要
The aim of cross-modal retrieval is to search for flexible results across different types of multimedia data. However, the labeled data is usually limited and not well paired with different modalities in practical applications. These issues are not well addressed in the existing works, which cannot consider the semantic information about unlabeled and unpaired data, synchronously. Self-training is a well-known strategy to handle semi-supervised problems. Motivated by the self-training, this paper proposes a self-training-based cross-modal hashing framework (STCH) to tackle the semi-supervised and semi-paired challenges. In the framework, graph neural networks are used to capture potential intra-modality and inter-modality similarities to produce pseudo labels. Then the inconsistent pseudo labels of different modalities are refined with a heuristic filter to enhance the model robustness. To train STCH, we propose an alternating learning strategy to conduct the self-train by predicting pseudo labels during the training procedure, which can be seamlessly incorporated into semi-supervised and supervised learning. In this way, the proposed method can leverage sufficient semantic information to enhance the semi-supervised effect and address the semi-paired problem. Experiments on the real-world datasets demonstrate that our approach outperforms related methods on hash cross-modal retrieval.
更多查看译文
关键词
cross-modal retrieval,self-training,semi-supervised,semi-paired
AI 理解论文
溯源树
样例
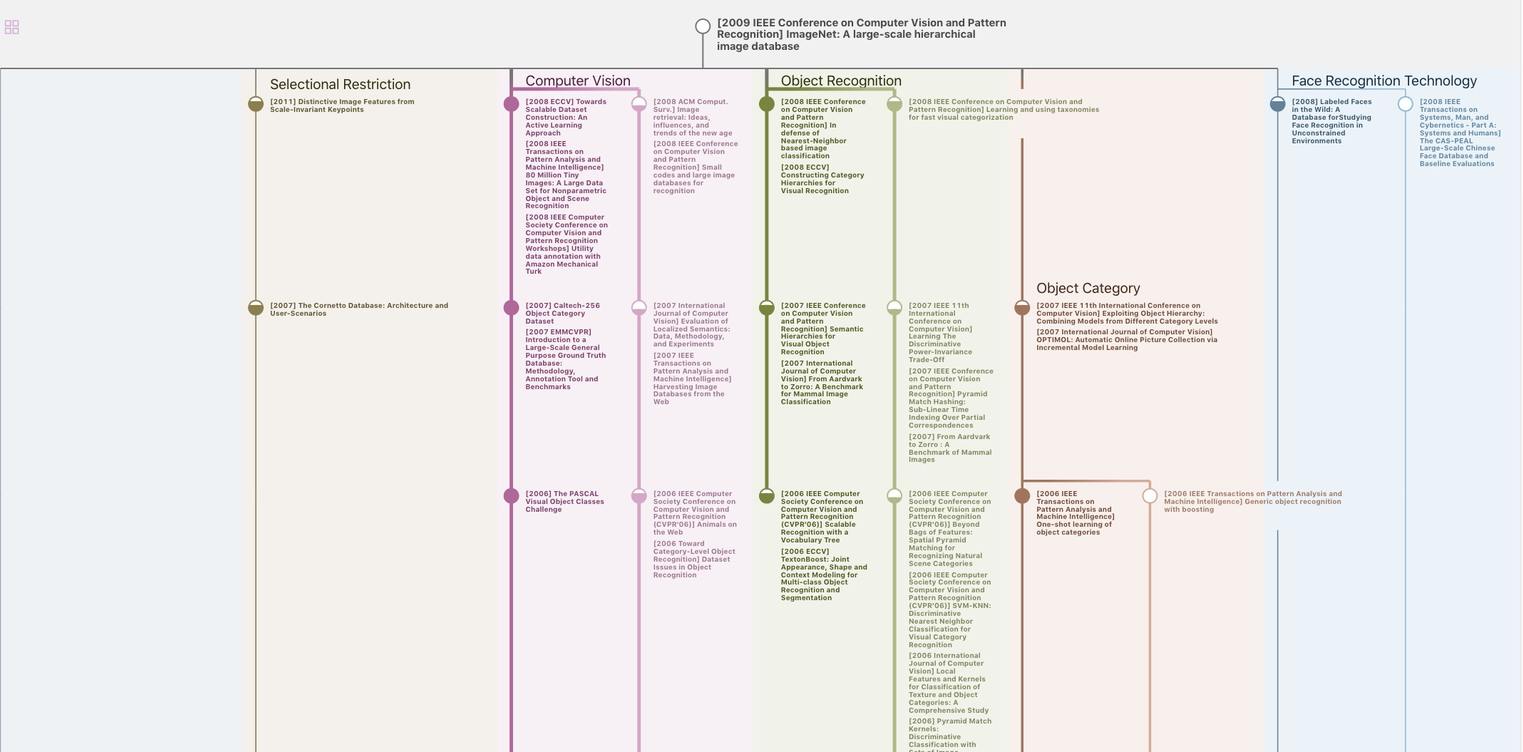
生成溯源树,研究论文发展脉络
Chat Paper
正在生成论文摘要