Adaptive Sparse Self-attention for Object Detection
2022 International Joint Conference on Neural Networks (IJCNN)(2022)
摘要
Object detection is a fundamental task for computer vision. The majority of prior methods employ global contextual information to enhance features representation. However, we argue that prior methods suffer from the superfluous features. To address the above mentioned problems, we explore the sparsity on object detection tasks in two dimensions. Specifically, a sparse spatial attention is proposed to capture global sparse long-range relationship into local features adaptively, where a learnable channel-wise mask is obtained to reduce the superfluous channels. Meanwhile, a sparse channel attention module is used to enhance the representation of each channel by introducing sparse global semantics. Experiments demonstrate that our proposed method outperforms comparative methods on the commonly used benchmark dataset, i.e., MS-COCO. The ablative experiments show that the sparsity the effectiveness in feature extraction and bounding boxes selection.
更多查看译文
关键词
sparse global semantics,comparative methods,feature extraction,bounding boxes selection,adaptive sparse self-attention,computer vision,global contextual information,features representation,superfluous features,object detection tasks,sparse spatial attention,global sparse long-range relationship,local features,learnable channel-wise mask,superfluous channels,sparse channel attention module
AI 理解论文
溯源树
样例
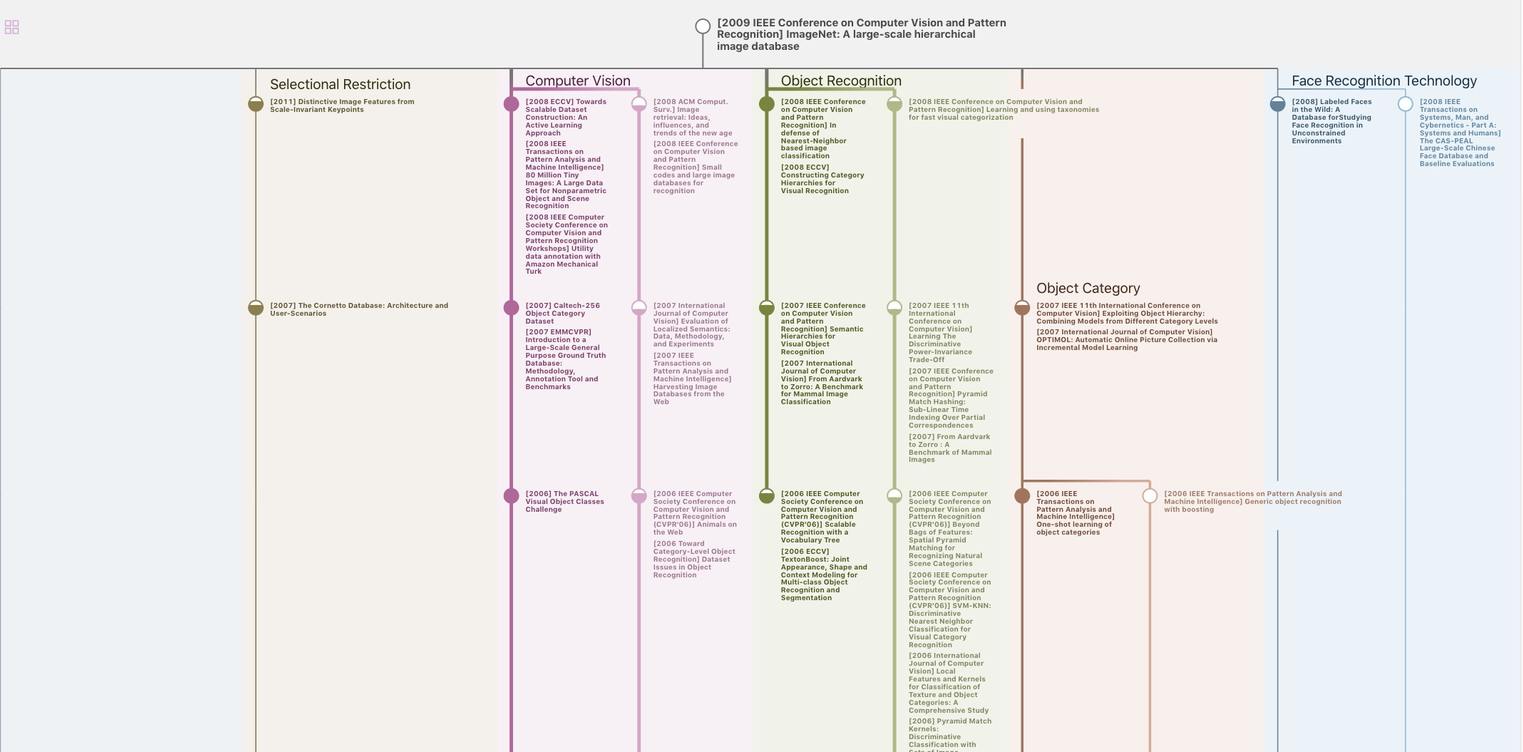
生成溯源树,研究论文发展脉络
Chat Paper
正在生成论文摘要