CoGNet: Cooperative Graph Neural Networks
2022 International Joint Conference on Neural Networks (IJCNN)(2022)
摘要
Graph representation learning has received increasing attention in recent years for many real-world applications. A major challenge in graph representation learning is the lack of labeled data. To address this challenge, Graph Neural Networks (GNNs) use message passing frameworks to combine information from unlabeled data with labeled data. However, the use of unlabeled data under the message passing framework is indirect in the training process where unlabeled data does not supervise the training process. To fully exploit the potential of unlabeled data, we propose a novel dual-view cooperative training framework for graph data where unlabeled data is involved in the training process for supervision. Specifically, we regard different views as the reasoning processes of two GNN models with which the models make predictions, integrating the understanding of different models on the underlying graph. To exchange information between models, we design a pseudo-label-based approach, where the two models mutually provide pseudo labels to each other iteratively. Moreover, to ensure the quality of pseudo labels, we propose an entropy-based pseudo-labels selection procedure and we adopt GNNExplainer to visualize different views in our framework. Our comprehensive experimental evaluation shows that our methods can boost the performance of state-of-the-art models.
更多查看译文
关键词
unlabeled data,training process,graph neural networks,graph representation learning,message passing framework,graph data,dual-view cooperative training framework,CoGNet,entropy-based pseudolabels selection procedure,GNNExplainer
AI 理解论文
溯源树
样例
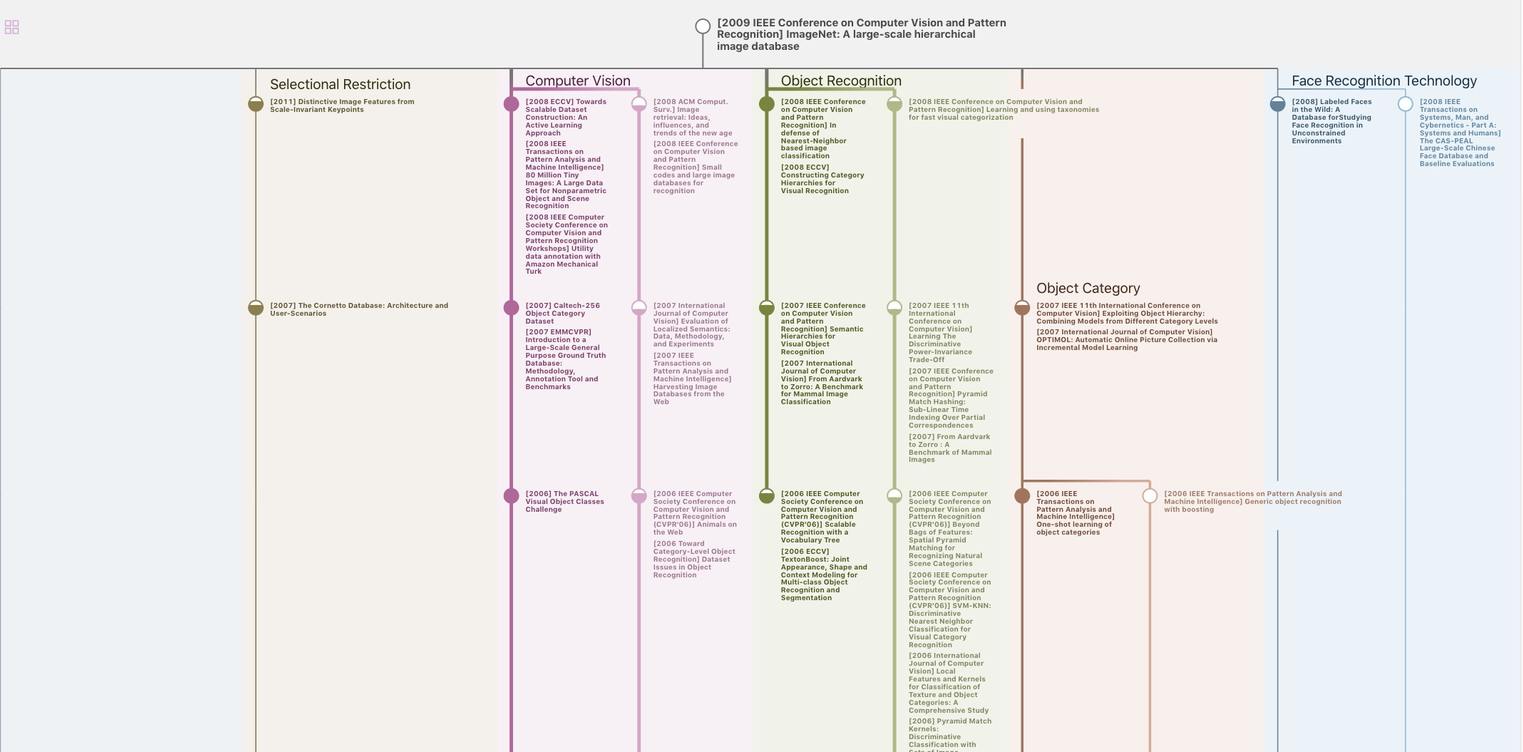
生成溯源树,研究论文发展脉络
Chat Paper
正在生成论文摘要