Improved Multiclass AdaBoost Using Sparse Oblique Decision Trees
IEEE International Joint Conference on Neural Network (IJCNN)(2022)
摘要
Boosting, one of the most effective machine learning frameworks, has attracted an enduring interest since its introduction 30 years ago. The majority of boosting methods use trees as base learner and, while much work has focused on theoretical and empirical variations of boosting, there has been surprisingly little progress on the tree learning procedure itself. To this day, each individual tree is typically axis-aligned (which is ill-suited to model correlations and results in relatively weak classifiers), and is learned using a greedy divide-and-conquer approach such as CART or C5.0, which produces suboptimal trees. We show we can improve boosted forests drastically by making each tree a much stronger classifier. We do this by using sparse oblique trees, which are far more powerful than axis-aligned ones, and by optimizing them using "tree alternating optimization" (TAO), suitably modified to handle the base learner optimization problem dictated by the boosting framework. Focusing on two versions of AdaBoost, we show that the resulting forests not only are consistently and considerably more accurate than random forests or gradient boosting, but that they use a very small number of trees and a comparable number of parameters.
更多查看译文
关键词
supervised learning,AdaBoost,decision trees
AI 理解论文
溯源树
样例
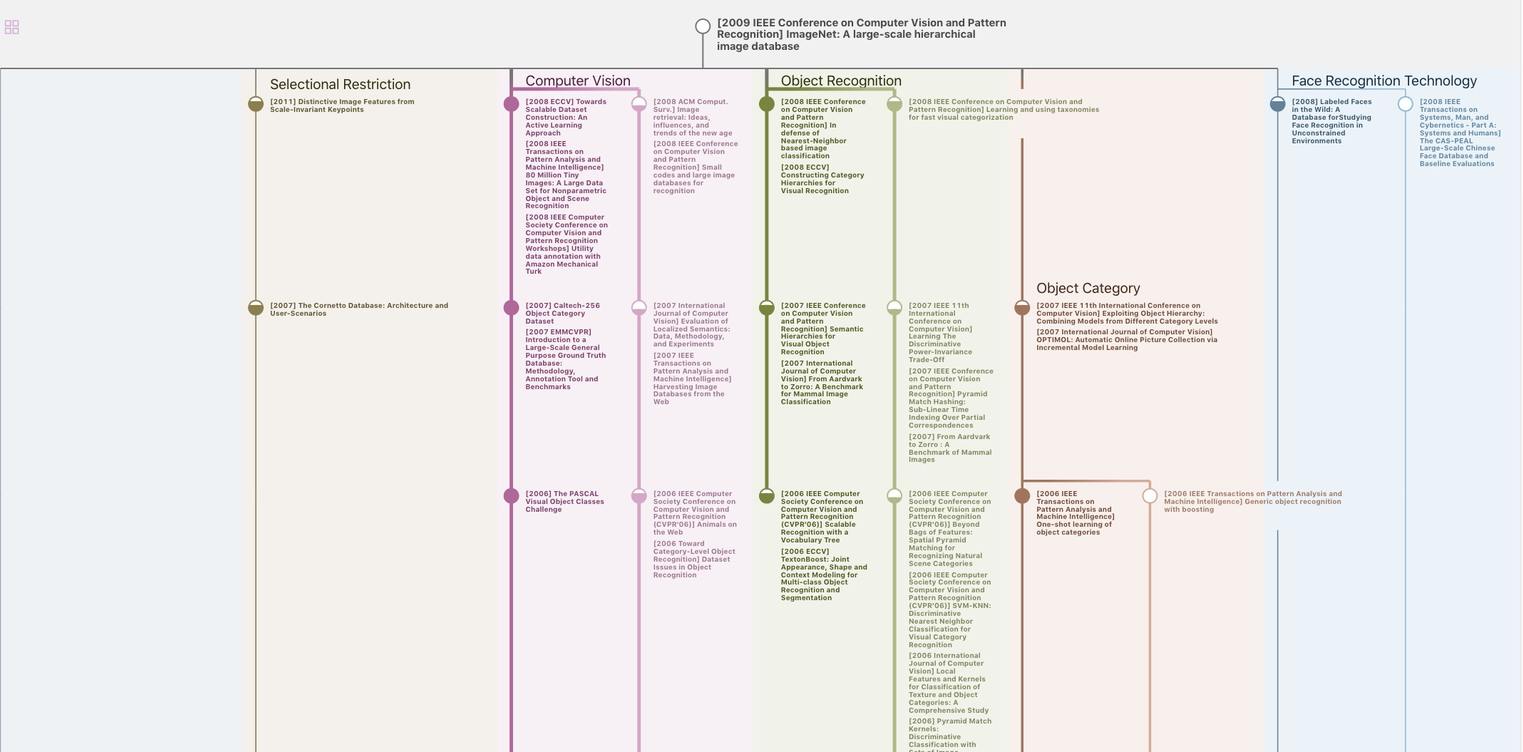
生成溯源树,研究论文发展脉络
Chat Paper
正在生成论文摘要