A geometric proof of the equivalence between AUC_ROC and Gini index area metrics for binary classifier performance assessment
IEEE International Joint Conference on Neural Network (IJCNN)(2022)
摘要
Some area metrics are used in different domains for assessing inequalities / discriminating power. The Area Under the ROC curve is used for assessing the quality of binary classifiers while the Gini index is used for measuring inequalities in populational studies related to dichotomic wealth distribution, in general. Do they have anything in common? This paper proves they are related and quantifies the equivalence between them. Despite the apparent difference between the curves, this paper presents a geometrically inspired proof which considers a compression of the area under the Lorenz curve which defines the Gini index. To overcome the area compression, the proof preserves the 1-to-1 correspondence between the ideal classifier on the ROC and Lorenz curves domains. Quantitatively, this paper proves that the Gini index normalized by that of the ideal classifier is equivalent to the area under the ROC curve above the chance diagonal (minus 0.5) also normalized by that of the ideal classifier. This also happens to be exactly the already known Somers' D metric and the Area Under the Kolmogorov-Smirnov curve (AUC_KS), recently proven. Therefore, this paper proposes these equivalent metrics to be a unified metric for binary classifiers' assessment Gini Index Ratio = 2*(AUC_ROC - 0.5) = 2 * AUC_KS = Somers' D metric. This integrates theoretical approaches used independently in different domains for measuring classes' separability in binary problems with the Somers' D metric already proved quantitatively equivalent to 2*(AUC ROC-0.5).
更多查看译文
关键词
Binary classification performance metrics,Area Under the ROC curve,Gini index,Area Under the KS curve
AI 理解论文
溯源树
样例
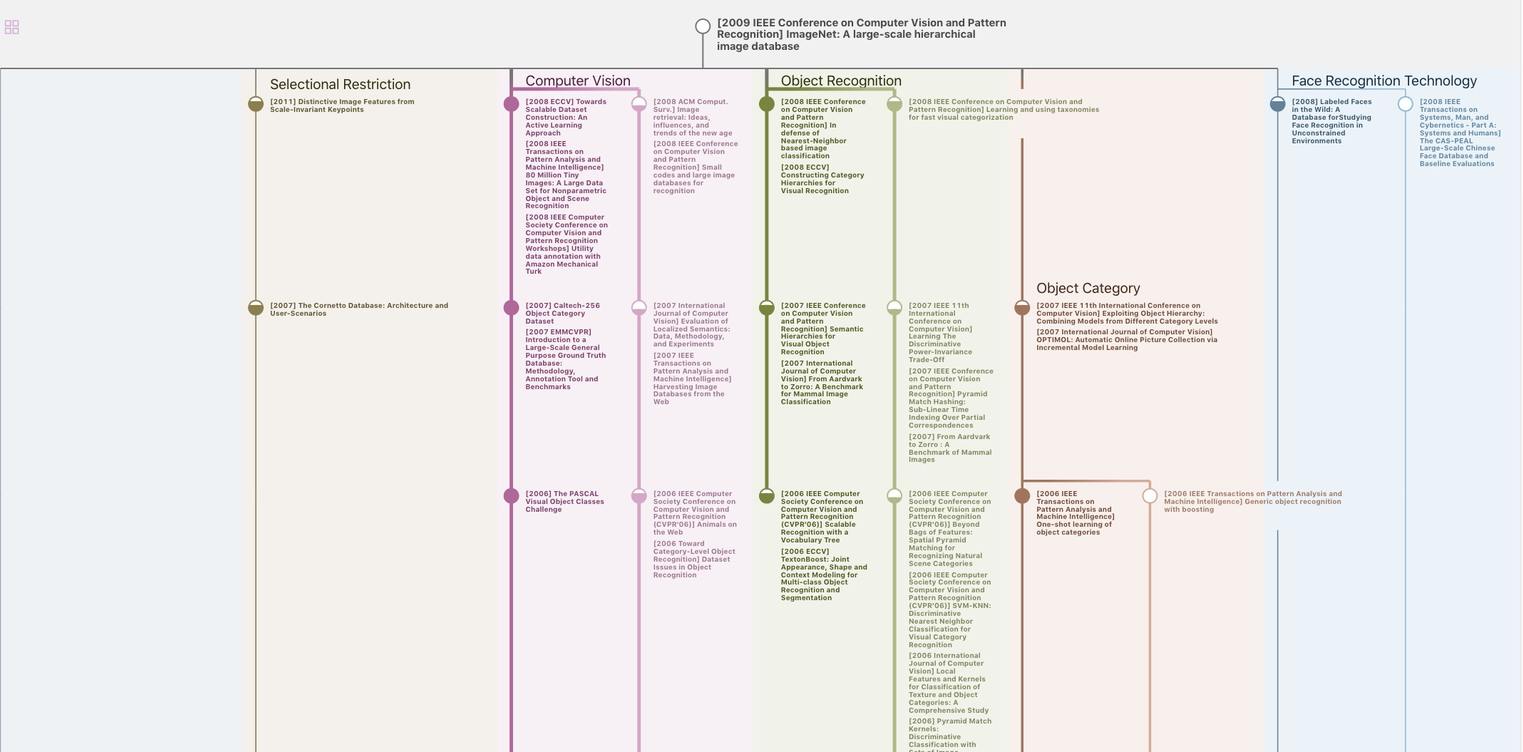
生成溯源树,研究论文发展脉络
Chat Paper
正在生成论文摘要