Case study: few-shot pill recognition
Meta-Learning with Medical Imaging and Health Informatics Applications(2023)
摘要
In this chapter, we present our previous study of few-shot pill recognition [1] as a case study to demonstrate how few-shot/meta learning could be applied for medical use-cases. Pill image recognition is vital for many personal/public healthcare applications and should be robust to diverse unconstrained real-world conditions. Most existing pill recognition models are limited in tackling this challenging few-shot/meta learning problem due to the insufficient instances per category. With limited training data, neural network based models have limitations in discovering the most discriminating features or going deeper. Especially, existing models fail to handle the hard samples taken under less controlled imaging conditions. More specifically, in this chapter, we will introduce 1) a new pill image database, namely CURE, is first developed with more varied imaging conditions and instances for each pill category; 2) a lightweight W2-net is proposed for better pill segmentation; 3) a MultiStream (MS) deep network that captures task-related features along with a novel two-stage training methodology is proposed. Within the proposed framework, a Batch All strategy that considers all the samples is first employed for the substreams, and then a Batch Hard strategy that considers only the hard samples mined in the first stage is utilized for the fusion network. By doing so, complex samples that could not be represented by one type of feature could be focused and the model could be forced to exploit other domain-related information more effectively. Experiment results show that the proposed model outperforms state-of-the-art models on both the National Institute of Health (NIH) and our CURE database.
更多查看译文
关键词
recognition,few-shot
AI 理解论文
溯源树
样例
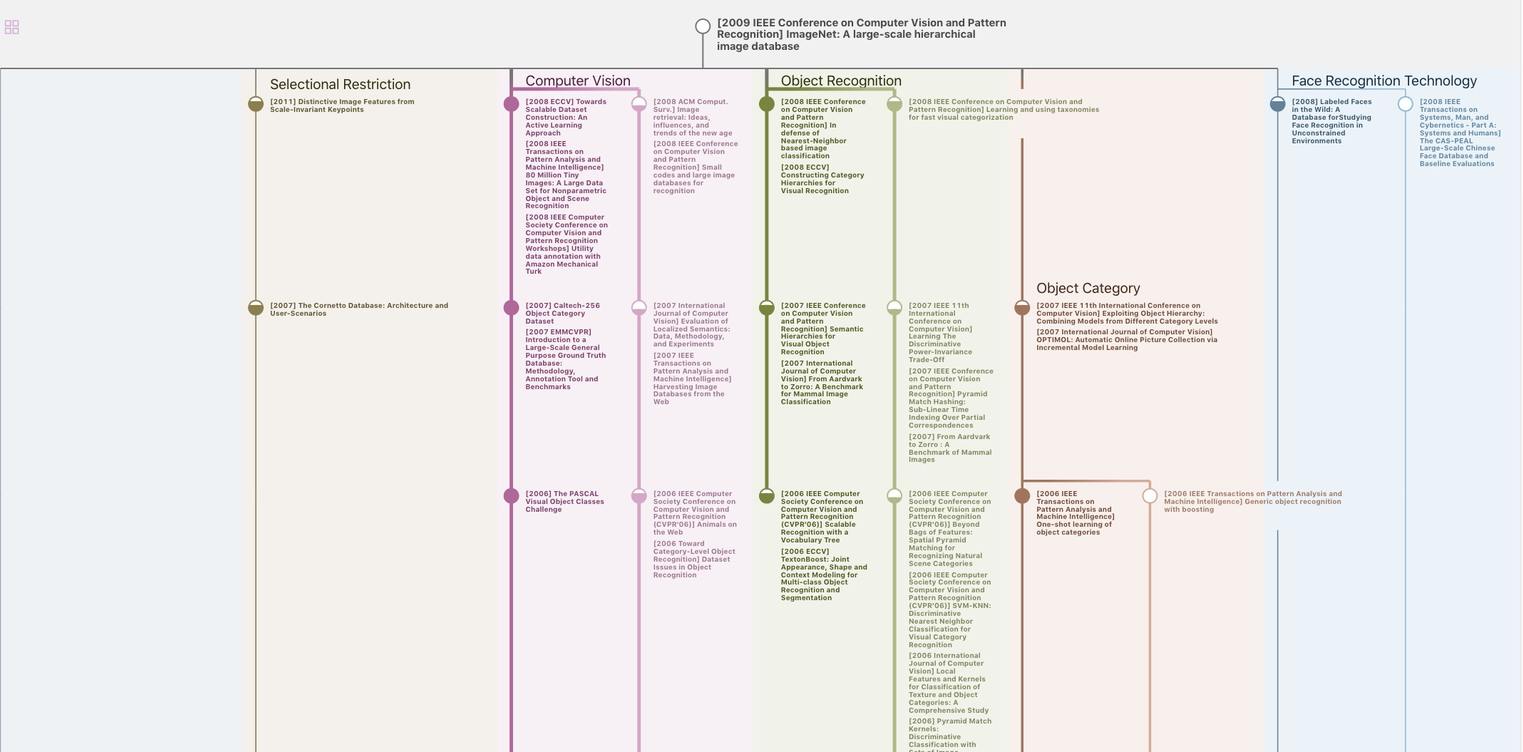
生成溯源树,研究论文发展脉络
Chat Paper
正在生成论文摘要