Linear expectile regression under massive data
Fundamental Research(2021)
摘要
In this paper, we study the large-scale inference for a linear expectile regression model. To mitigate the computational challenges in the classical asymmetric least squares (ALS) estimation under massive data, we propose a communication-efficient divide and conquer algorithm to combine the information from sub-machines through confidence distributions. The resulting pooled estimator has a closed-form expression, and its consistency and asymptotic normality are established under mild conditions. Moreover, we derive the Bahadur representation of the ALS estimator, which serves as an important tool to study the relationship between the number of sub-machines K and the sample size. Numerical studies including both synthetic and real data examples are presented to illustrate the finite-sample performance of our method and support the theoretical results.
更多查看译文
关键词
Divide and conquer algorithm,Expectile regression,(Asymptotic) confidence distribution,Massive data
AI 理解论文
溯源树
样例
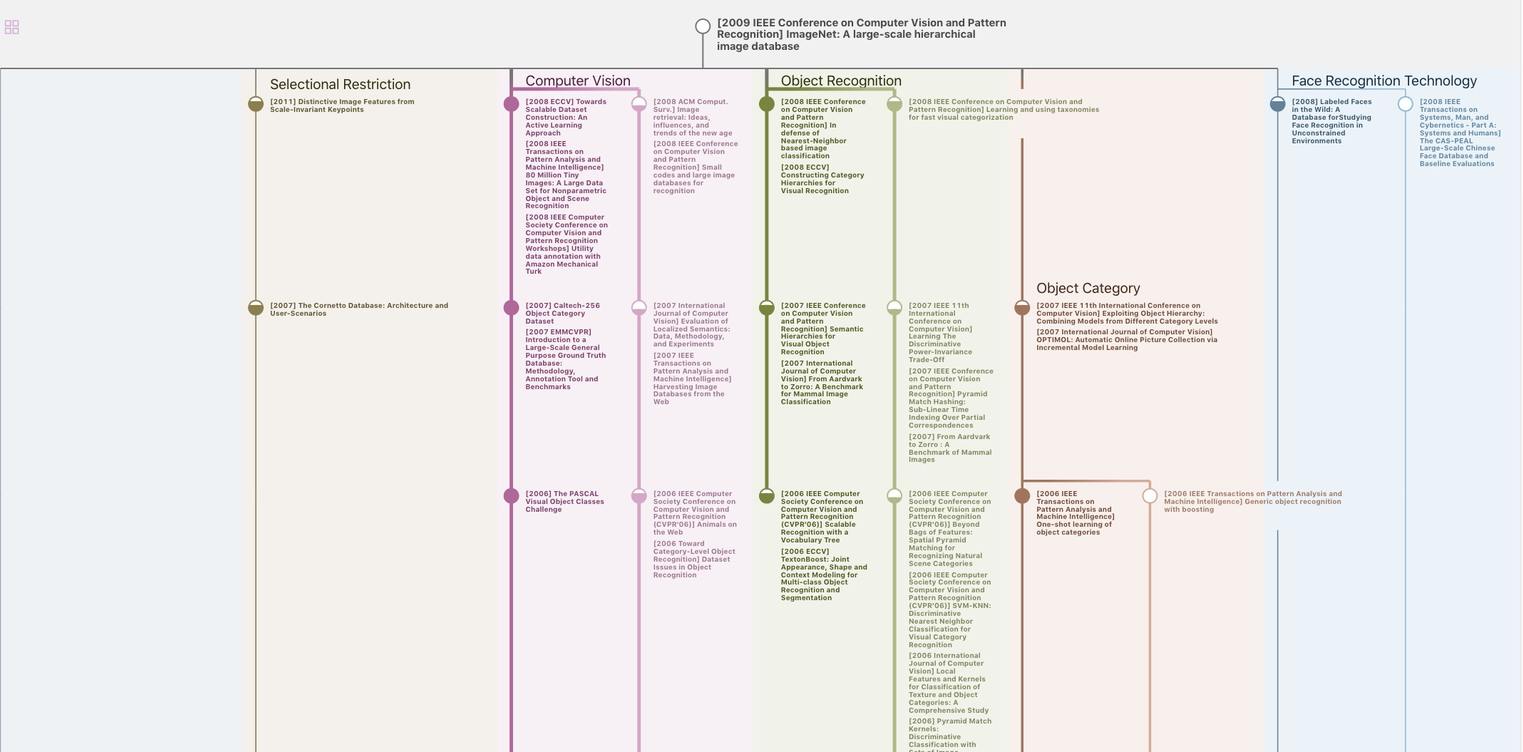
生成溯源树,研究论文发展脉络
Chat Paper
正在生成论文摘要