Architecting a Flash-Based Storage System for Low-Cost Inference of Extreme-Scale DNNs
IEEE Transactions on Computers(2022)
摘要
The size of deep neural network (DNN) models has been exploding rapidly, demanding a colossal amount of memory capacity. For example, Google has recently scaled its Switch Transformer to have a parameter size of up to 6.4 TB. However, today's HBM DRAM-based memory system for GPUs and DNN accelerators is suboptimal for these extreme-scale DNNs as it fails to provide enough capacity while its massive bandwidth is poorly utilized. Thus, we propose Leviathan, a DNN inference accelerator, which integrates a cost-effective flash-based storage system, instead. We carefully architect the storage system to provide enough memory bandwidth while preventing performance drop caused by read disturbance errors. Our evaluation of Leviathan demonstrates an 8.3x throughput gain compared to the iso-FLOPS DNN accelerator with conventional SSDs and up to 19.5x higher memory cost-efficiency than the HBM-based DNN accelerator.
更多查看译文
关键词
DNN inference,deep neural networks (DNNs),hardware accelerator,solid-state drive (SSD),storage device
AI 理解论文
溯源树
样例
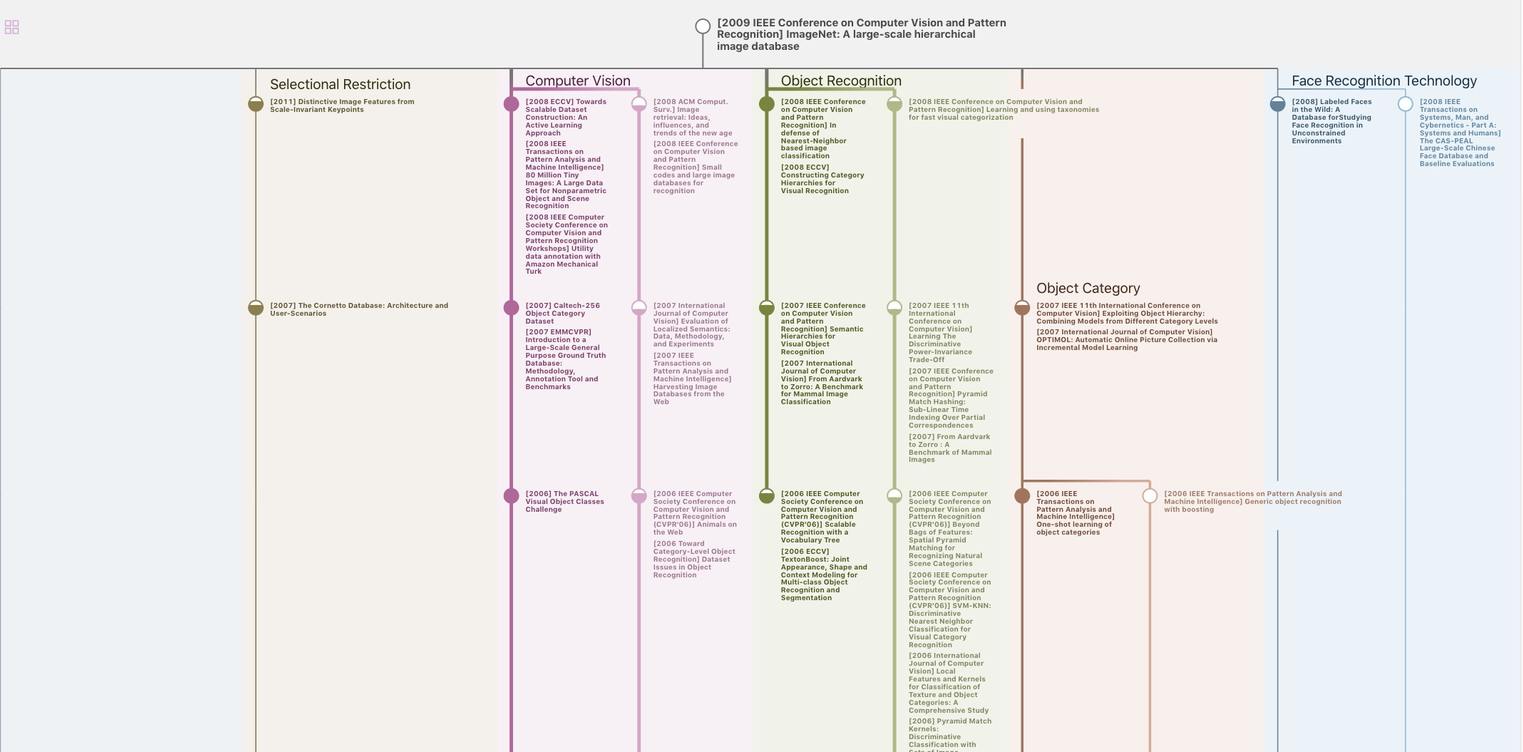
生成溯源树,研究论文发展脉络
Chat Paper
正在生成论文摘要