Toward High-Performance Map-Recovery of Air Pollution Using Machine Learning
ACS ES&T Engineering(2022)
摘要
Mobile and pervasive sampling of urban air pollution has been increasingly valued as a sustainable method, in terms of economic and operational factors, for surveying atmospheric environment with high space-time resolution. Specifically, fine granular air quality (AQ) inference provides fundamental progress toward data-driven urban management, as it estimates grid-level pollutant concentrations constantly using pollutant measurement data collected from fixed and mobile sensors. In this paper, we propose a tree-based multicascade space-time learning model (MCST-Tree) for AQ inference to recover pollution maps by exploiting multisource AQ samples (fixed and mobile) and heterogeneous urban feature sets (land-use, meteorology, population, traffic, etc.). This is implemented and evaluated in a study case of Chengdu (4900 km2, 14 June to 14 July 2018), which achieves map-recovery of PM2.5 distribution based upon the sparse measurements (ca. 16.2% space-time coverage) with high-performance (symmetric mean average percentage error (SMAPE) (%) = 14.13%; R2 = 0.94). Detailed evaluations are presented through the analysis of model performance, space-time coverage of mobile sampling, and AQ inference. We conduct a series of sensitivity analyses of mobile sampling coverage, and the experimental results show that it is a critical issue to enhance the model trust, which contributes to improve the R-square from 0.81 (fixed data + 10% mobile data) to 0.94 (fixed data + 100% mobile data). The results show that the mobile sampling significantly improves the space-time modeling capability, and our proposed model has great potential to achieve map-recovery for air pollution at high spatial-temporal resolution with high performance.
更多查看译文
关键词
map-recovery,MCST-Tree,low-cost sensors,big data
AI 理解论文
溯源树
样例
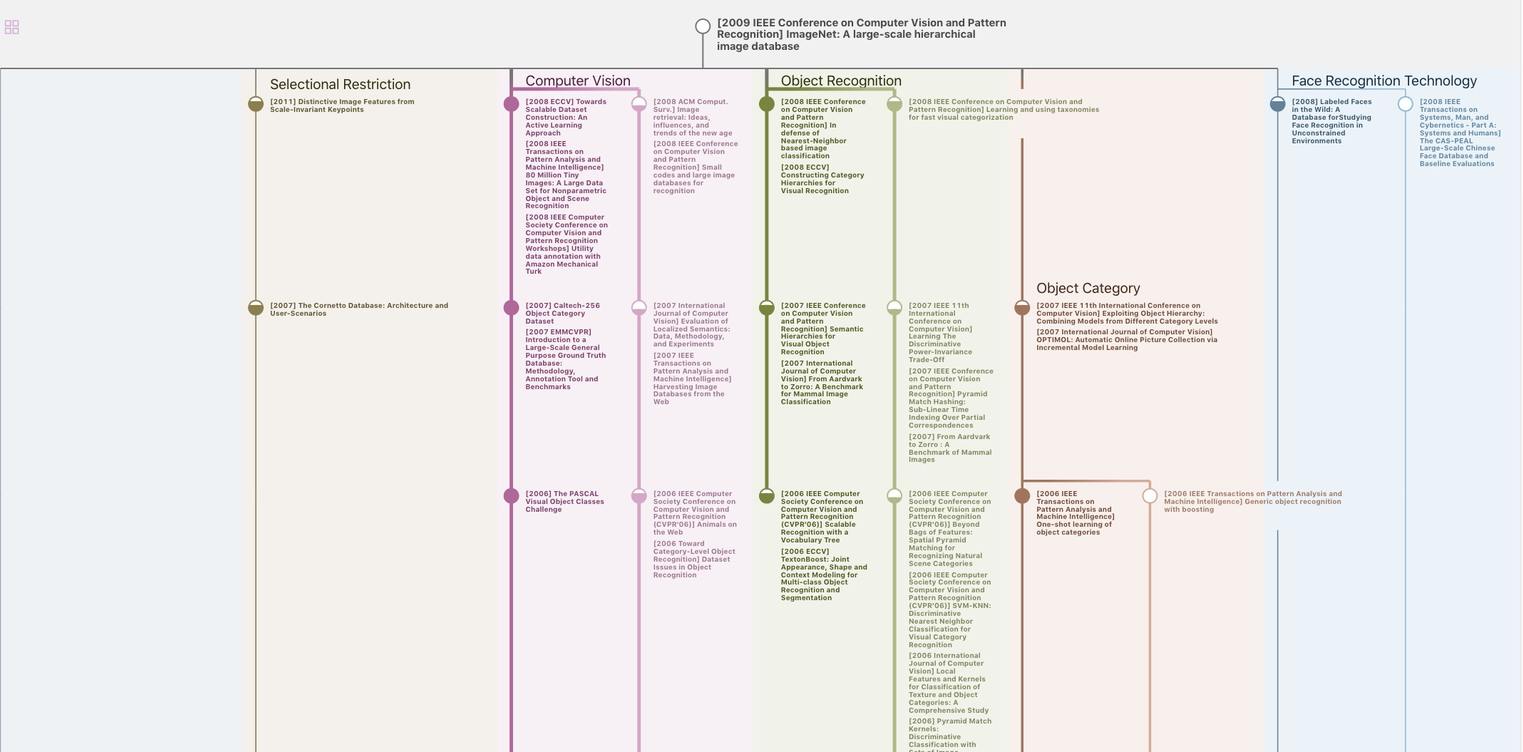
生成溯源树,研究论文发展脉络
Chat Paper
正在生成论文摘要