Explaining Deep Learning Models for Per-packet Encrypted Network Traffic Classification
2022 IEEE International Symposium on Measurements & Networking (M&N)(2022)
摘要
Machine learning is increasingly applied to network traffic analysis to aid in tasks such as quality of service management, trend monitoring, and security. Recent advances in deep learning have enabled not only the classification of encrypted transits, but classification on a per-packet level. End-to-end deep learning models are becoming increasingly ubiq-uitous given their ease of use, i.e., developers do not need to engineer features, and their apparent versatility. However, deep learning entails black-box models that hinder the capability to debug and explain classifications. Moreover, the computational complexity of deep learning can incur unnecessary latency, which is problematic for real-time classification needs. In this paper, we propose a methodology to interpret black-box, deep learning-based encrypted network traffic classification models, with an attempt to understand the dominant features a classifier is focusing on for a given task. We evaluate our approach on state-of-the-art deep learning classification techniques for encrypted per-packet classification and demonstrate how interpretability can be used to debug and improve the training pipeline while significantly reducing the size of the deep learning model. We propose future directions toward optimizing model performance while maintaining explainability.
更多查看译文
关键词
Encrypted Network Traffic Classification,Inter-pretable Machine Learning
AI 理解论文
溯源树
样例
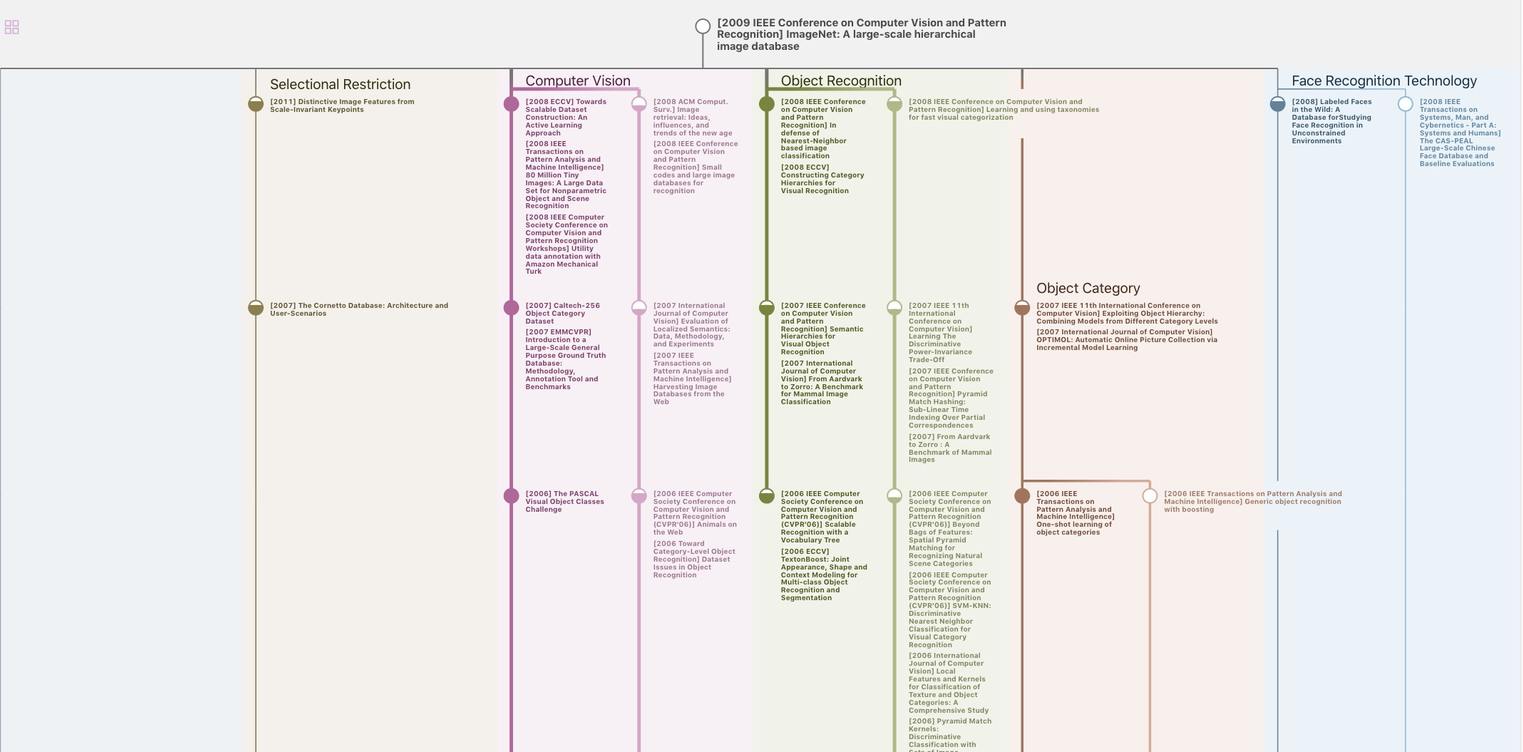
生成溯源树,研究论文发展脉络
Chat Paper
正在生成论文摘要