Taming Client Dropout and Improving Efficiency for Distributed Differential Privacy in Federated Learning
arxiv(2023)
摘要
Federated learning (FL) is increasingly deployed among multiple clients (e.g., mobile devices) to train a shared model over decentralized data. To address the privacy concerns, FL systems need to protect the clients' data from being revealed during training, and also control the data leakage through trained models when exposed to untrusted domains. Distributed differential privacy (DP) offers an appealing solution in this regard as it achieves an informed tradeoff between privacy and utility without a trusted server. However, existing distributed DP mechanisms work impractically in the presence of client dropout, resulting in either poor privacy guarantees or degraded training accuracy. In addition, these mechanisms also suffer from severe efficiency issues with long time-to-accuracy training performance. We present Hyades, a distributed differentially private FL framework that is highly efficient and resilient to client dropout. Specifically, we develop a novel 'add-then-remove' scheme where a required noise level can be enforced in each FL training round even though some sampled clients may drop out in the end; therefore, the privacy budget is consumed carefully even in the presence of client dropout. To boost performance, Hyades runs as a distributed pipeline architecture via encapsulating the communication and computation operations into stages. It automatically divides the global model aggregation into several chunk-aggregation tasks and pipelines them for optimal speedup. Evaluation through large-scale cloud deployment shows that Hyades can efficiently handle client dropout in various realistic FL scenarios, attaining the optimal privacy-utility tradeoff and accelerating the training by up to 2.1$\times$ compared to existing solutions.
更多查看译文
AI 理解论文
溯源树
样例
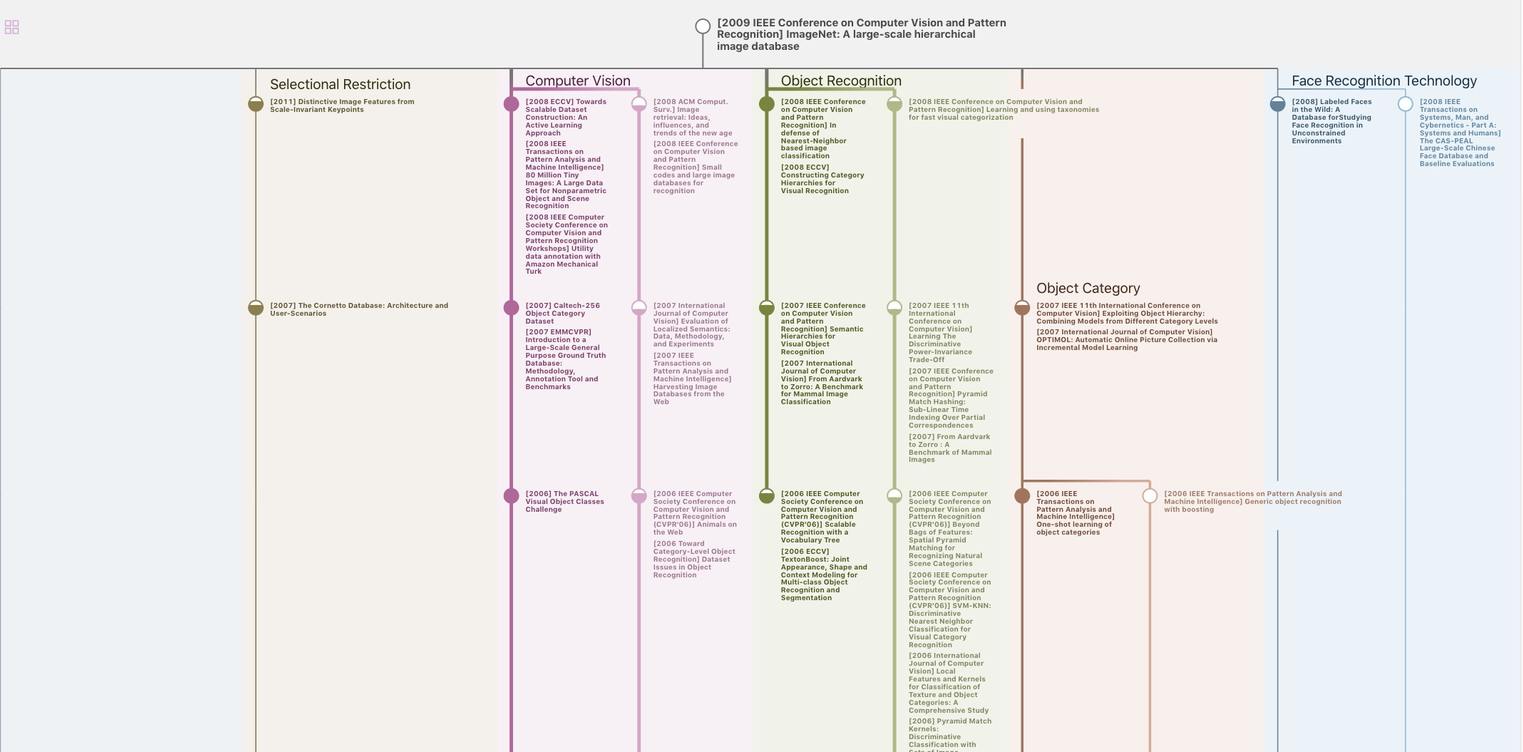
生成溯源树,研究论文发展脉络
Chat Paper
正在生成论文摘要