One-shot learning of stochastic differential equations with data adapted kernels
Physica D: Nonlinear Phenomena(2023)
摘要
We consider the problem of learning Stochastic Differential Equations of the form dXt=f(Xt)dt+σ(Xt)dWt from one sample trajectory. This problem is more challenging than learning deterministic dynamical systems because one sample trajectory only provides indirect information on the unknown functions f, σ, and stochastic process dWt representing the drift, the diffusion, and the stochastic forcing terms, respectively. We propose a method that combines Computational Graph Completion [1] and data adapted kernels learned via a new variant of cross validation. Our approach can be decomposed as follows: (1) Represent the time-increment map Xt→Xt+dt as a Computational Graph in which f, σ and dWt appear as unknown functions and random variables. (2) Complete the graph (approximate unknown functions and random variables) via Maximum a Posteriori Estimation (given the data) with Gaussian Process (GP) priors on the unknown functions. (3) Learn the covariance functions (kernels) of the GP priors from data with randomized cross-validation. Numerical experiments illustrate the efficacy, robustness, and scope of our method.
更多查看译文
关键词
Stochastic differential equations,Times series forecasting,Computational graph completion,Kernel methods,Machine learning,Gaussian Processes
AI 理解论文
溯源树
样例
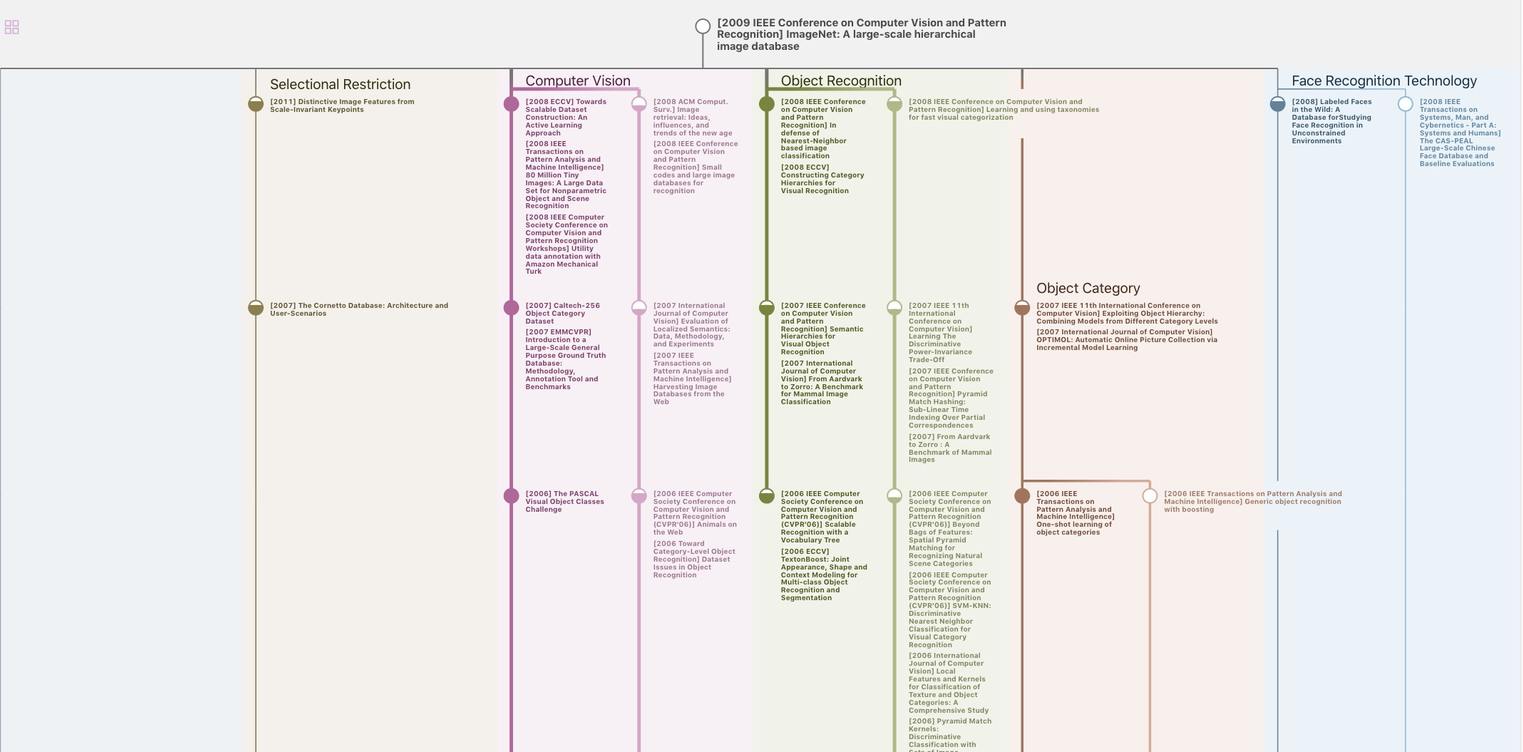
生成溯源树,研究论文发展脉络
Chat Paper
正在生成论文摘要