Whodunit? Learning to Contrast for Authorship Attribution.
AACL/IJCNLP (1)(2022)
摘要
Authorship attribution is the task of identifying the author of a given text. The key is finding representations that can differentiate between authors. Existing approaches typically use manually designed features that capture a dataset's content and style, but these approaches are dataset-dependent and yield inconsistent performance across corpora. In this work, we propose \textit{learning} author-specific representations by fine-tuning pre-trained generic language representations with a contrastive objective (Contra-X). We show that Contra-X learns representations that form highly separable clusters for different authors. It advances the state-of-the-art on multiple human and machine authorship attribution benchmarks, enabling improvements of up to 6.8% over cross-entropy fine-tuning. However, we find that Contra-X improves overall accuracy at the cost of sacrificing performance for some authors. Resolving this tension will be an important direction for future work. To the best of our knowledge, we are the first to integrate contrastive learning with pre-trained language model fine-tuning for authorship attribution.
更多查看译文
关键词
contrast,learning
AI 理解论文
溯源树
样例
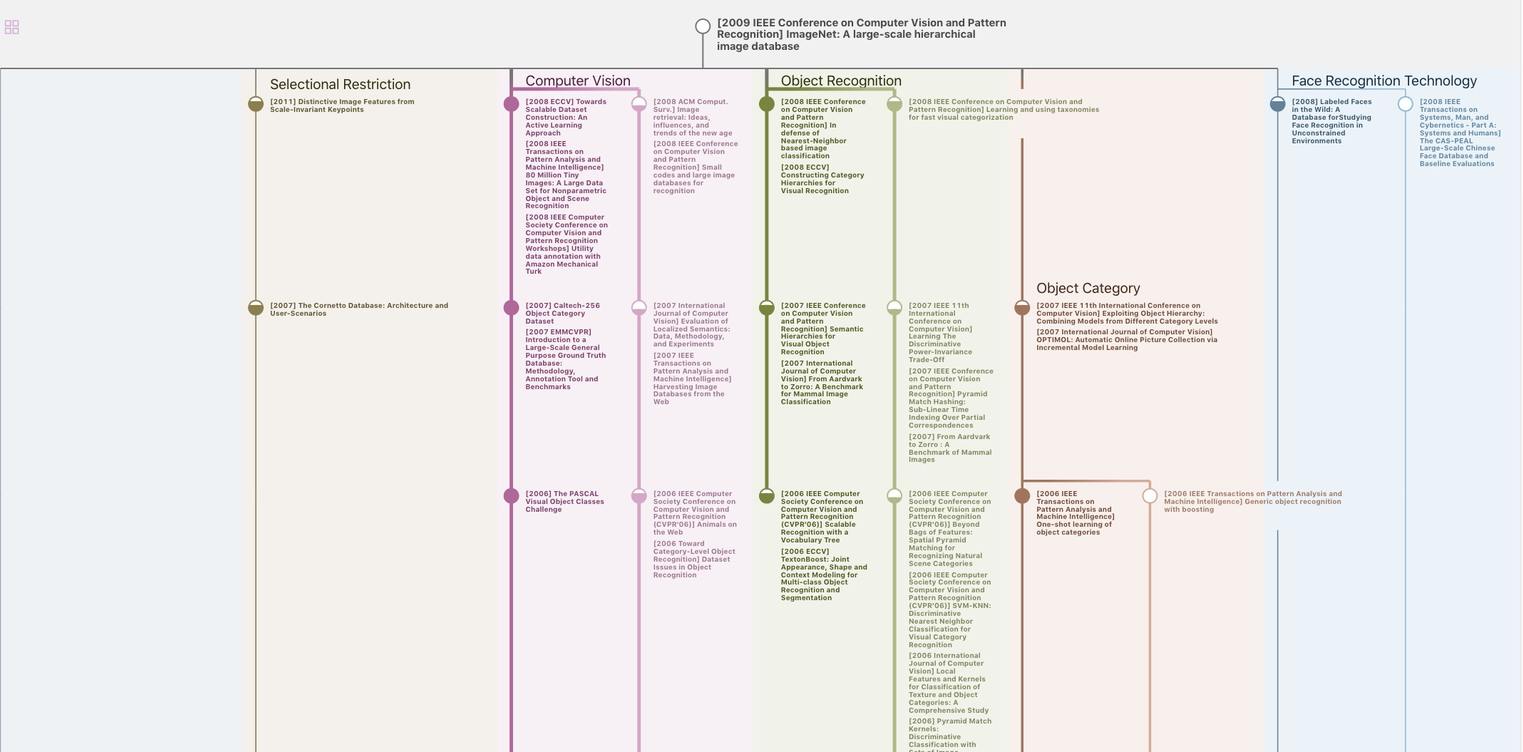
生成溯源树,研究论文发展脉络
Chat Paper
正在生成论文摘要