DenseCL: A simple framework for self-supervised dense visual pre-training
Visual Informatics(2023)
摘要
Self-supervised learning aims to learn a universal feature representation without labels. To date, most existing self-supervised learning methods are designed and optimized for image classification. These pre-trained models can be sub-optimal for dense prediction tasks due to the discrepancy between image-level prediction and pixel-level prediction. To fill this gap, we aim to design an effective, dense self-supervised learning framework that directly works at the level of pixels (or local features) by tak-ing into account the correspondence between local features. Specifically, we present dense contrastive learning (DenseCL), which implements self-supervised learning by optimizing a pairwise contrastive (dis)similarity loss at the pixel level between two views of input images. Compared to the supervised ImageNet pre-training and other self-supervised learning methods, our self-supervised DenseCL pre -training demonstrates consistently superior performance when transferring to downstream dense prediction tasks including object detection, semantic segmentation and instance segmentation. Specif-ically, our approach significantly outperforms the strong MoCo-v2 by 2.0% AP on PASCAL VOC object detection, 1.1% AP on COCO object detection, 0.9% AP on COCO instance segmentation, 3.0% mIoU on PASCAL VOC semantic segmentation and 1.8% mIoU on Cityscapes semantic segmentation. The improvements are up to 3.5% AP and 8.8% mIoU over MoCo-v2, and 6.1% AP and 6.1% mIoU over supervised counterpart with frozen-backbone evaluation protocol.Code and models are available at: https://git.io/DenseCL (c) 2023 The Authors. Published by Elsevier B.V. on behalf of Zhejiang University and Zhejiang University Press Co. Ltd. This is an open access article under the CC BY-NC-ND license (http://creativecommons.org/licenses/by-nc-nd/4.0/).
更多查看译文
关键词
Self-supervised learning,Visual pre-training,Dense prediction tasks
AI 理解论文
溯源树
样例
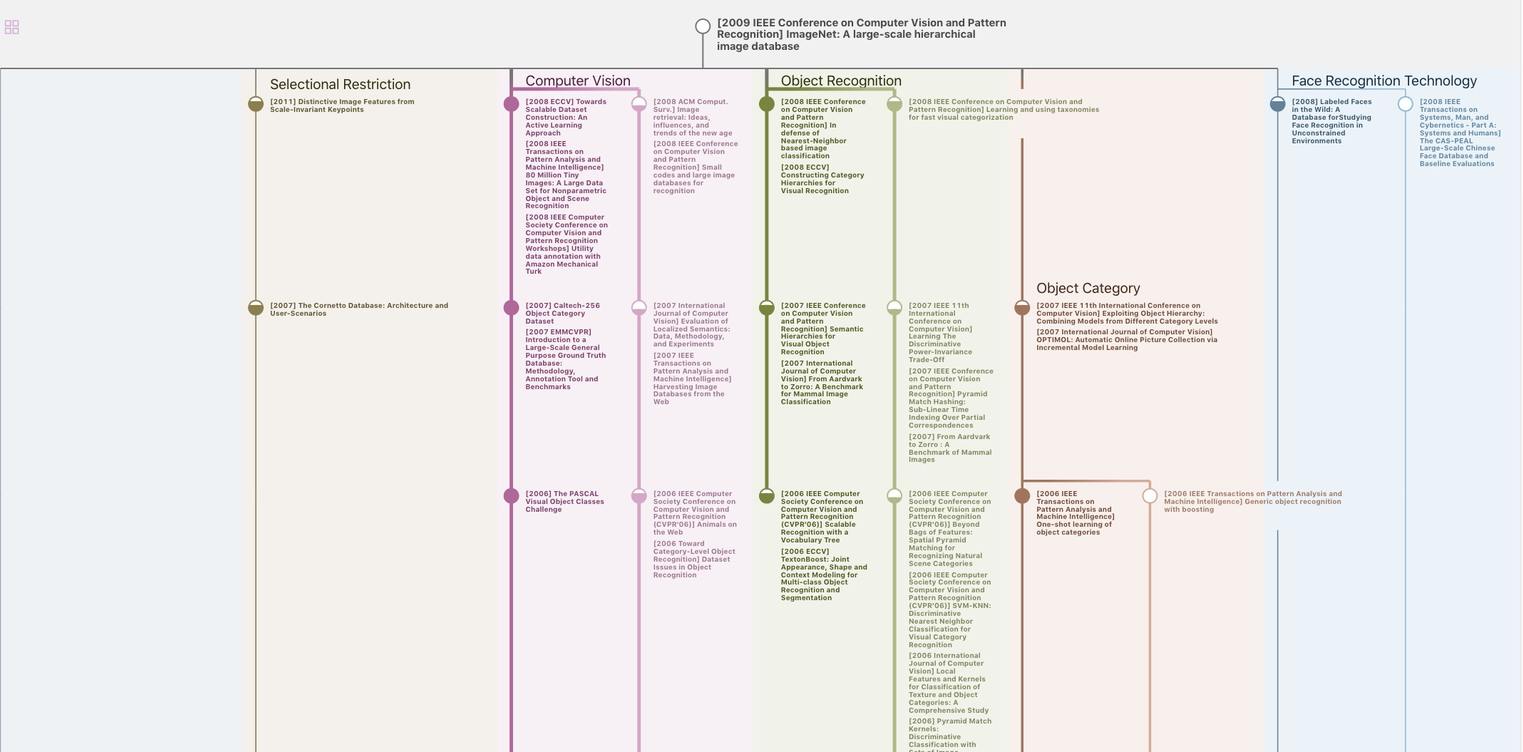
生成溯源树,研究论文发展脉络
Chat Paper
正在生成论文摘要