Alternating Direction Method of Multipliers for Convolutive Non-Negative Matrix Factorization
IEEE TRANSACTIONS ON CYBERNETICS(2023)
摘要
Non-negative matrix factorization (NMF) has become a popular method for learning interpretable patterns from data. As one of the variants of standard NMF, convolutive NMF (CNMF) incorporates an extra time dimension to each basis, known as convolutive bases, which is well suited for representing sequential patterns. Previously proposed algorithms for solving CNMF use multiplicative updates which can be derived by either heuristic or majorization–minimization (MM) methods. However, these algorithms suffer from problems, such as low convergence rates, difficulty to reach exact zeroes during iterations and prone to poor local optima. Inspired by the success of alternating direction method of multipliers (ADMMs) on solving NMF, we explore variable splitting (i.e., the core idea of ADMM) for CNMF in this article. New closed-form algorithms of CNMF are derived with the commonly used
$\beta $
-divergences as optimization objectives. Experimental results have demonstrated the efficacy of the proposed algorithms on their faster convergence, better optima, and sparser results than state-of-the-art baselines.
更多查看译文
关键词
Alternating direction method of multipliers (ADMMs),beta-divergence,convolutive basis,non-negative matrix factorization (NMF)
AI 理解论文
溯源树
样例
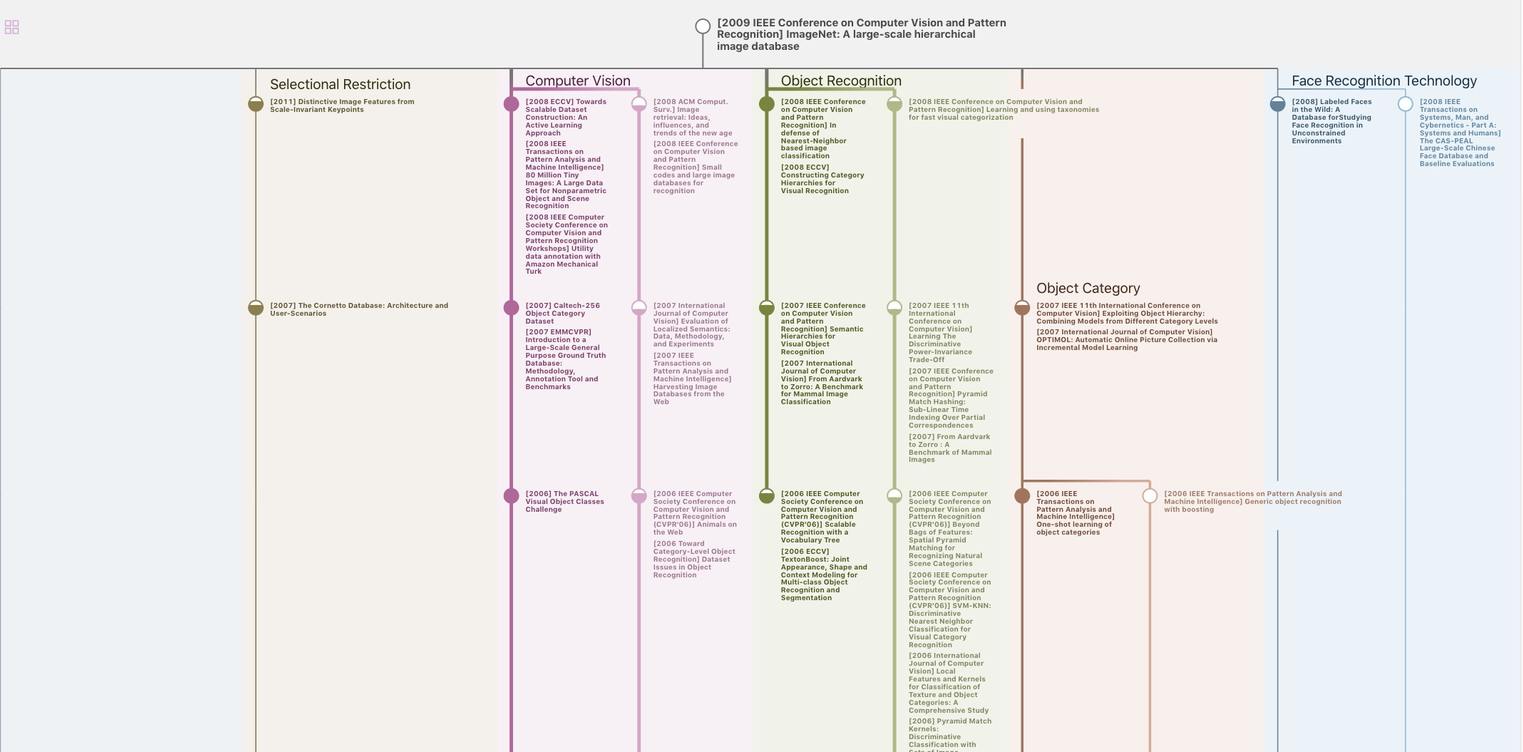
生成溯源树,研究论文发展脉络
Chat Paper
正在生成论文摘要