Predicting hospital admission from emergency department triage data for patients presenting with fall-related fractures
Internal and emergency medicine(2022)
摘要
Purpose Predict in advance the need for hospitalization of adult patients for fall-related fractures based on information available at the time of triage to help decision-making at the emergency department (ED). Methods We developed machine learning models using routinely collected triage data at a regional hospital chain in Pennsylvania to predict admission to an inpatient unit. We considered all patients presenting to the ED for fall-related fractures. Patients who were 18 years or younger, who left the ED against medical advice, left the ED waiting room without being seen by a provider, and left the ED after initial diagnostics were excluded from the analysis. We compared models obtained using triage data (pre-model) with models developed using additional data obtained after physicians’ diagnoses (post-model). Results Our results show good discriminatory power on predicting hospital admissions. Neural network models performed the best (AUC: pre-model = 0.938 [CI 0.920–0.956], post-model = 0.983 [0.974–0.992]). The logistic regression analysis provides additional insights into the data and the relationships between the variables. Conclusions Using limited data available at the time of triage, we developed four machine learning models aimed at predicting hospitalization for patients presenting to the ED for fall-related fractures. All the four models were robust and performed well. Neural network method, however, performed the best for both pre- and post-models. Simple, parsimonious machine learning models can provide high accuracy for predicting hospital admission.
更多查看译文
关键词
Emergency department,Hospitalization,Machine learning,Predictive models
AI 理解论文
溯源树
样例
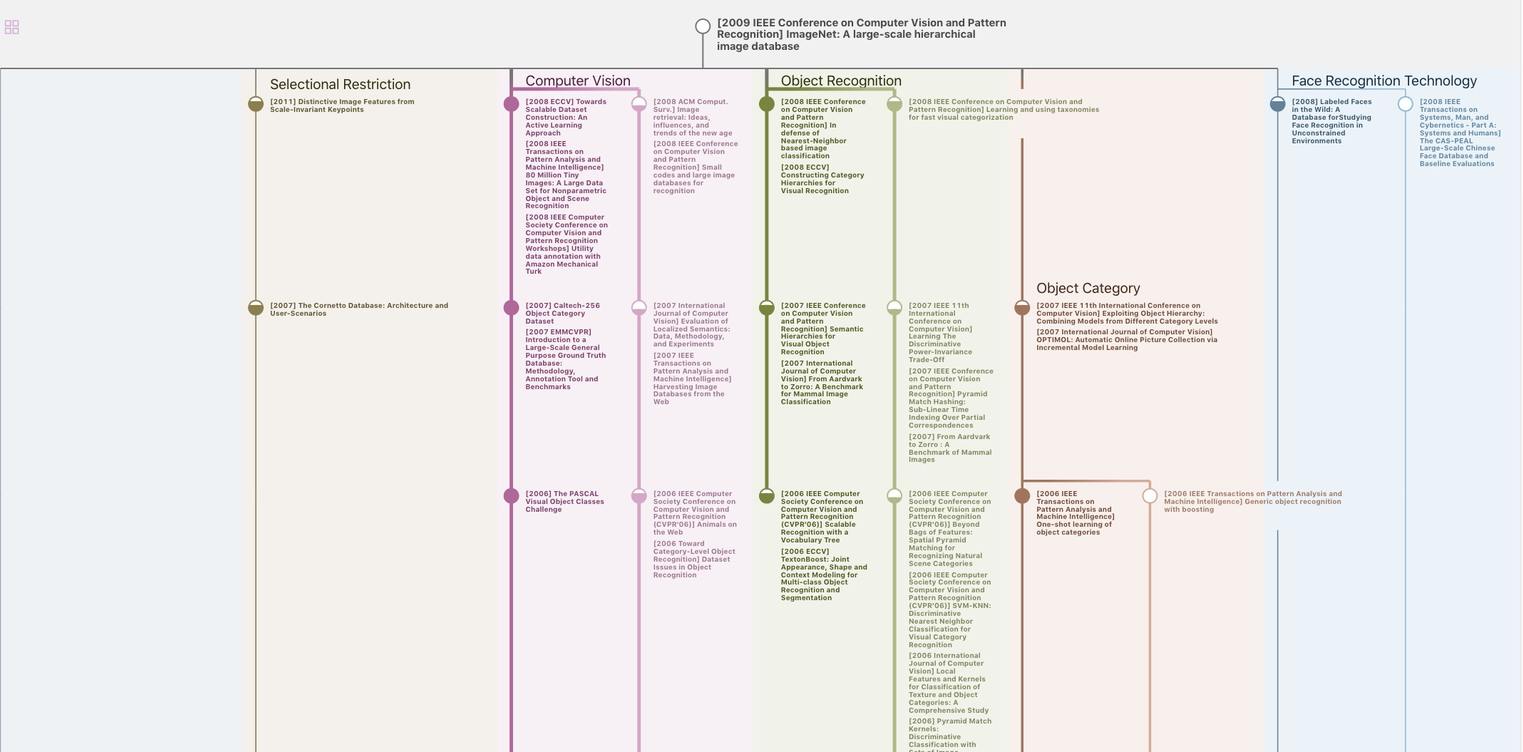
生成溯源树,研究论文发展脉络
Chat Paper
正在生成论文摘要