Dynamic Time-Alignment of Dimensional Annotations of Emotion using Recurrent Neural Networks
arxiv(2022)
摘要
Most automatic emotion recognition systems exploit time-continuous annotations of emotion to provide fine-grained descriptions of spontaneous expressions as observed in real-life interactions. As emotion is rather subjective, its annotation is usually performed by several annotators who provide a trace for a given dimension, i.e. a time-continuous series describing a dimension such as arousal or valence. However, annotations of the same expression are rarely consistent between annotators, either in time or in value, which adds bias and delay in the trace that is used to learn predictive models of emotion. We therefore propose a method that can dynamically compensate inconsistencies across annotations and synchronise the traces with the corresponding acoustic features using Recurrent Neural Networks. Experimental evaluations were carried on several emotion data sets that include Chinese, French, German, and Hungarian participants who interacted remotely in either noise-free conditions or in-the-wild. The results show that our method can significantly increase inter-annotator agreement, as well as correlation between traces and audio features, for both arousal and valence. In addition, improvements are obtained in the automatic prediction of these dimensions using simple light-weight models, especially for valence in noise-free conditions, and arousal for recordings captured in-the-wild.
更多查看译文
关键词
dimensional annotations,emotion,recurrent,neural networks,time-alignment
AI 理解论文
溯源树
样例
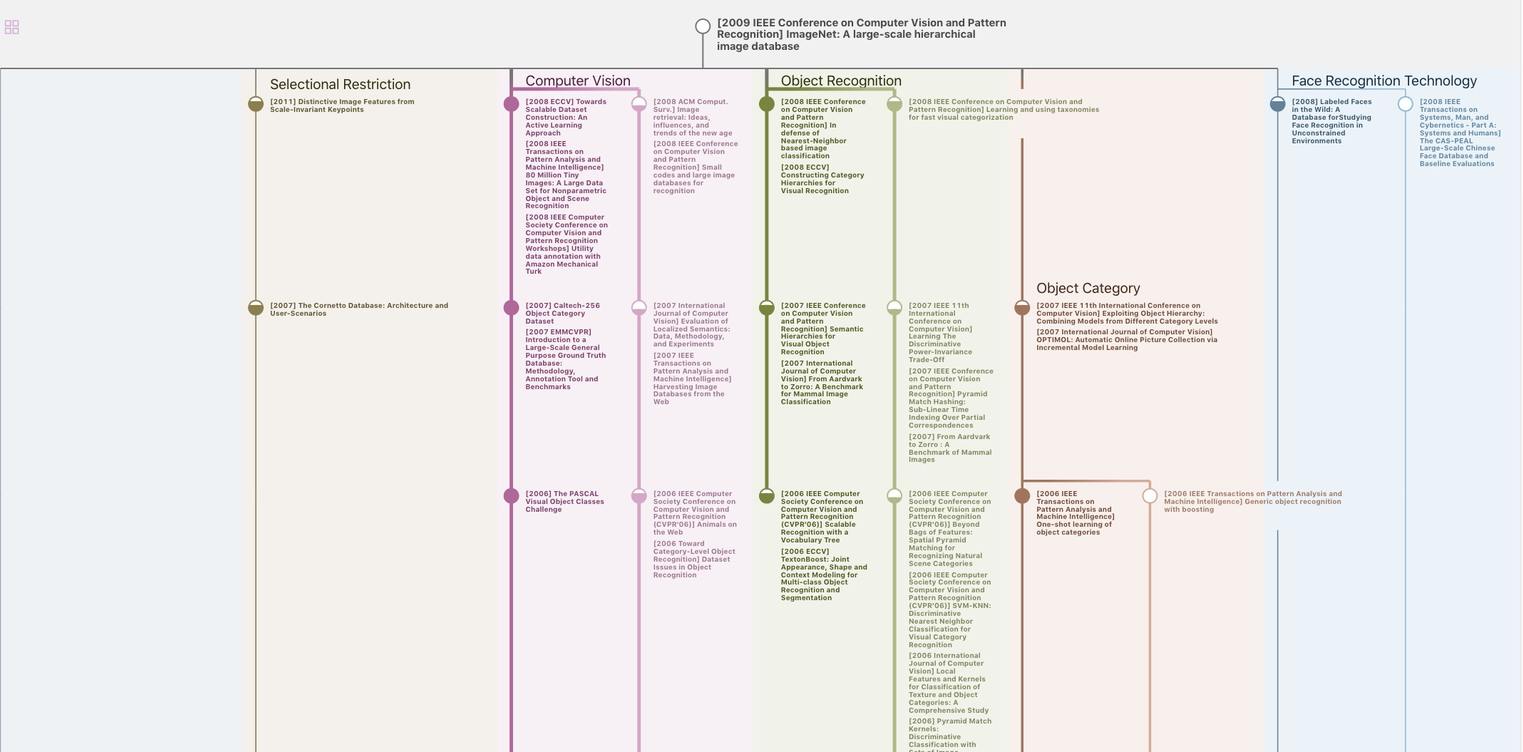
生成溯源树,研究论文发展脉络
Chat Paper
正在生成论文摘要