The effect of the perturber population on subhalo measurements in strong gravitational lenses
MONTHLY NOTICES OF THE ROYAL ASTRONOMICAL SOCIETY(2024)
摘要
Analyses of extended arcs in strong gravitational lensing images to date have constrained the properties of dark matter by measuring the parameters of one or two individual subhaloes. However, since such analyses are reliant on likelihood-based methods like Markov-chain Monte Carlo or nested sampling, they require various compromises to the realism of lensing models for the sake of computational tractability, such as ignoring the numerous other subhaloes and line-of-sight haloes in the system, assuming a particular form for the source model and requiring the noise to have a known likelihood function. Here, we show that a simulation-based inference method called truncated marginal neural ratio estimation (TMNRE) makes it possible to relax these requirements by training neural networks to directly compute marginal posteriors for subhalo parameters from lensing images. By performing a set of inference tasks on mock data, we verify the accuracy of TMNRE and show it can compute posteriors for subhalo parameters marginalized over populations of hundreds of substructures, as well as lens and source uncertainties. We also find that the multilayer perceptron (MLP) mixer network works far better for such tasks than the convolutional architectures explored in other lensing analyses. Furthermore, we show that since TMNRE learns a posterior function it enables direct statistical checks that would be extremely expensive with likelihood-based methods. Our results show that TMNRE is well-suited for analysing complex lensing data, and that the full subhalo and line-of-sight halo population must be included when measuring the properties of individual dark matter substructures with this technique.
更多查看译文
关键词
gravitational lensing: strong,methods: statistical,dark matter
AI 理解论文
溯源树
样例
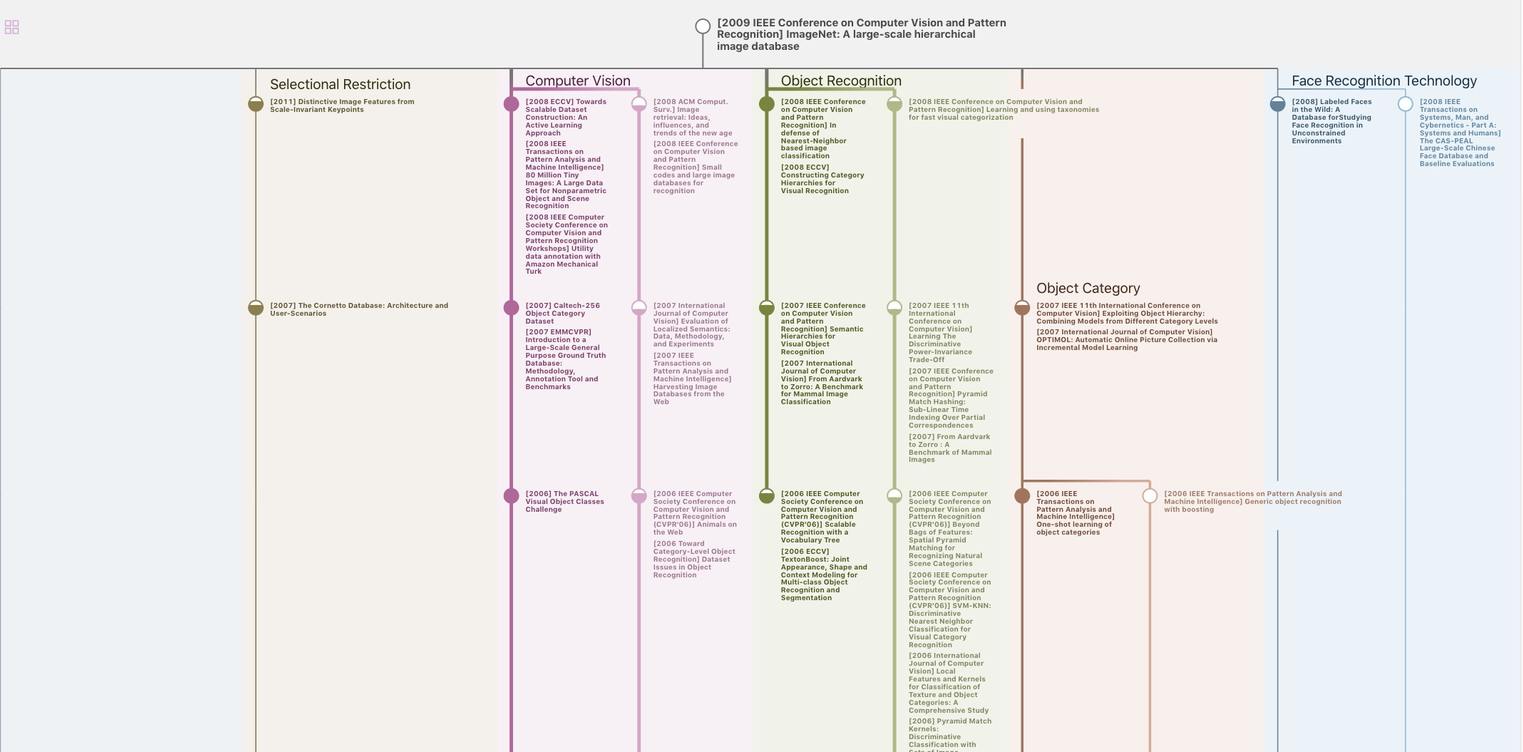
生成溯源树,研究论文发展脉络
Chat Paper
正在生成论文摘要