Enforcing the consensus between Trajectory Optimization and Policy Learning for precise robot control
2023 IEEE INTERNATIONAL CONFERENCE ON ROBOTICS AND AUTOMATION, ICRA(2023)
摘要
Reinforcement learning (RL) and trajectory optimization (TO) present strong complementary advantages. On one hand, RL approaches are able to learn global control policies directly from data, but generally require large sample sizes to properly converge towards feasible policies. On the other hand, TO methods are able to exploit gradient-based information extracted from simulators to quickly converge towards a locally optimal control trajectory which is only valid within the vicinity of the solution. Over the past decade, several approaches have aimed to adequately combine the two classes of methods in order to obtain the best of both worlds. Following on from this line of research, we propose several improvements on top of these approaches to learn global control policies quicker, notably by leveraging sensitivity information stemming from TO methods via Sobolev learning, and Augmented Lagrangian (AL) techniques to enforce the consensus between TO and policy learning. We evaluate the benefits of these improvements on various classical tasks in robotics through comparison with existing approaches in the literature.
更多查看译文
AI 理解论文
溯源树
样例
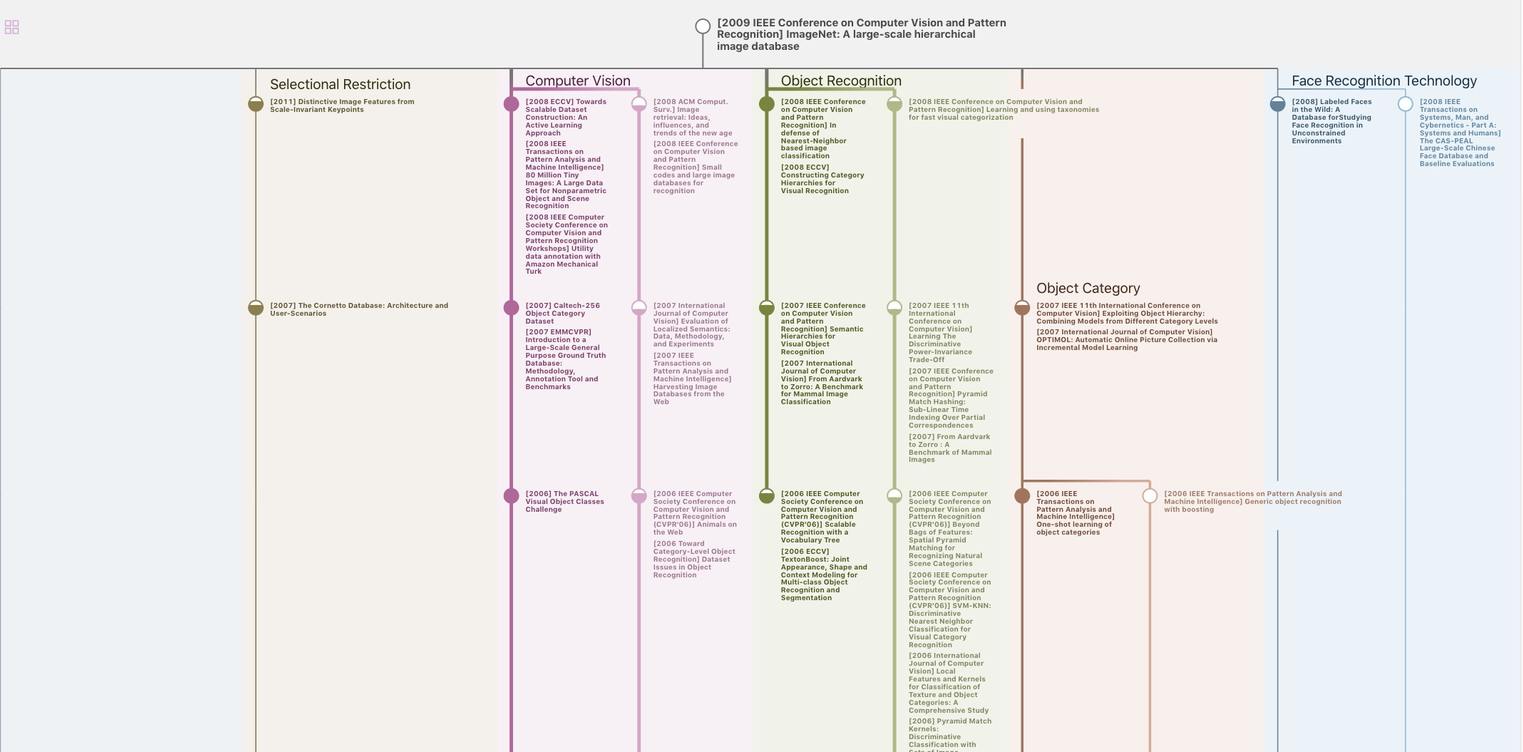
生成溯源树,研究论文发展脉络
Chat Paper
正在生成论文摘要