LATITUDE: Robotic Global Localization with Truncated Dynamic Low-pass Filter in City-scale NeRF
arxiv(2023)
摘要
Neural Radiance Fields (NeRFs) have made great success in representing complex 3D scenes with high-resolution details and efficient memory. Nevertheless, current NeRF - based pose estimators have no initial pose prediction and are prone to local optima during optimization. In this paper, we present LATITUDE: Global Localization with Truncated Dynamic Low-pass Filter, which introduces a two-stage localization mechanism in city-scale NeRF. In place recognition stage, we train a regressor through images generated from trained NeRFs, which provides an initial value for global localization. In pose optimization stage, we minimize the residual between the observed image and rendered image by directly optimizing the pose on the tangent plane. To avoid falling into local optimum, we introduce a Truncated Dynamic Low-pass Filter (TDLF) for coarse-to-fine pose registration. We evaluate our method on both synthetic and real-world data and show its potential applications for high-precision navigation in large-scale city scenes. Codes and dataset will be publicly available at https://github.com/jike5/LATITUDE.
更多查看译文
关键词
city-scale NeRF,current NeRF,initial pose prediction,large-scale city scenes,optimization stage,robotic Global Localization,trained NeRFs,Truncated Dynamic Low-pass Filter,two-stage localization mechanism
AI 理解论文
溯源树
样例
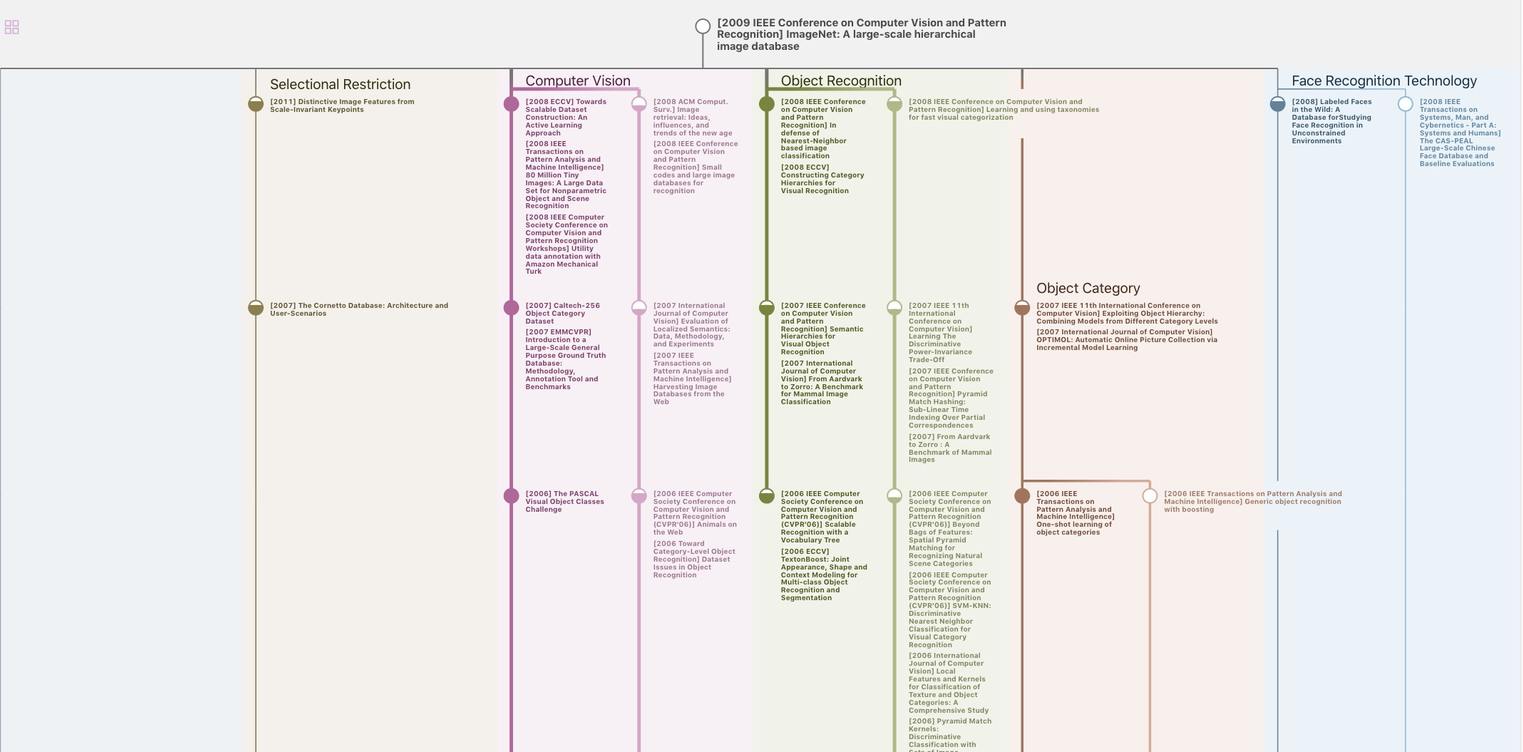
生成溯源树,研究论文发展脉络
Chat Paper
正在生成论文摘要