deepPIC: Deep Perceptual Image Clustering For Identifying Bias In Vision Datasets
IEEE Conference on Computer Vision and Pattern Recognition(2022)
摘要
Dataset bias in manually collected datasets is a known problem in computer vision. In safety-critical applications such as autonomous driving, these biases can lead to catastrophic errors from models trained on such datasets, jeopardizing the safety of users and their surroundings. Being able to unpuzzle the bias in a given dataset, and across datasets, is an essential tool for building safe and responsible AI. In this paper, we present deepPIC: deep Perceptual Image Clustering, a novel hierarchical clustering pipeline that leverages deep perceptual features to visualize and understand bias in unstructured and unlabeled datasets. It does so by effectively highlighting nuanced subcategories of information embedded within the data (such as multiple but repetitive shadow types) that typically are hard and/or expensive to annotate. Through experiments on a variety of image datasets, both open-source and internal, we demonstrate the effectiveness of deepPIC in (i) singling out errors in metadata from open-source datasets such as BDD100K; (ii) automatic nuanced metadata annotation; (iii) mining for edge cases; (iv) visualizing inherent bias both within and across multiple datasets; and (v) capturing synthetic data limitations; thus highlighting the wide variety of applications this pipeline can be applied to. All clustering results included here have been uploaded with image thumbnails on our project website - https://alchemz.github.io/unpuzzle_dataset_bias/. We recommend zooming in for best impact.
更多查看译文
关键词
deeppic perceptual image,bias,vision
AI 理解论文
溯源树
样例
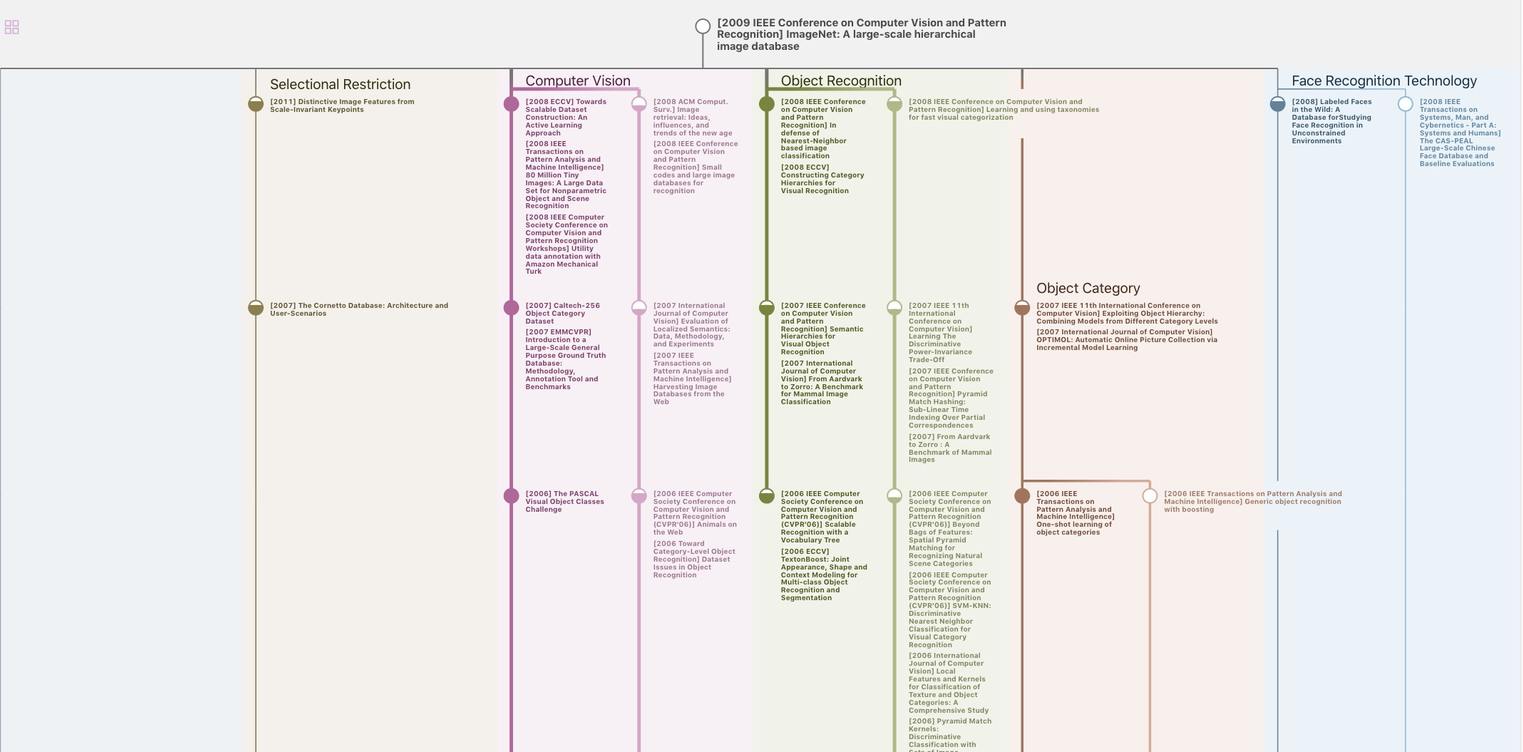
生成溯源树,研究论文发展脉络
Chat Paper
正在生成论文摘要