Attenuating Catastrophic Forgetting by Joint Contrastive and Incremental Learning
IEEE Conference on Computer Vision and Pattern Recognition(2022)
摘要
In class incremental learning, discriminative models are trained to classify images while adapting to new instances and classes incrementally. Training a model to adapt to new classes without total access to previous class data, however, leads to the known problem of catastrophic forgetting of the previously learnt classes. To alleviate this problem, we show how we can build upon recent progress on contrastive learning methods. In particular, we develop an incremental learning approach for deep neural networks operating both at classification and representation level which alleviates forgetting and learns more general features for data classification. Experiments performed on several datasets demonstrate the superiority of the proposed method with respect to well known state-of-the-art methods.
更多查看译文
关键词
state-of-the-art methods,joint contrastive,class incremental learning,discriminative models,class data,contrastive learning methods,incremental learning approach,deep neural networks,representation level,data classification
AI 理解论文
溯源树
样例
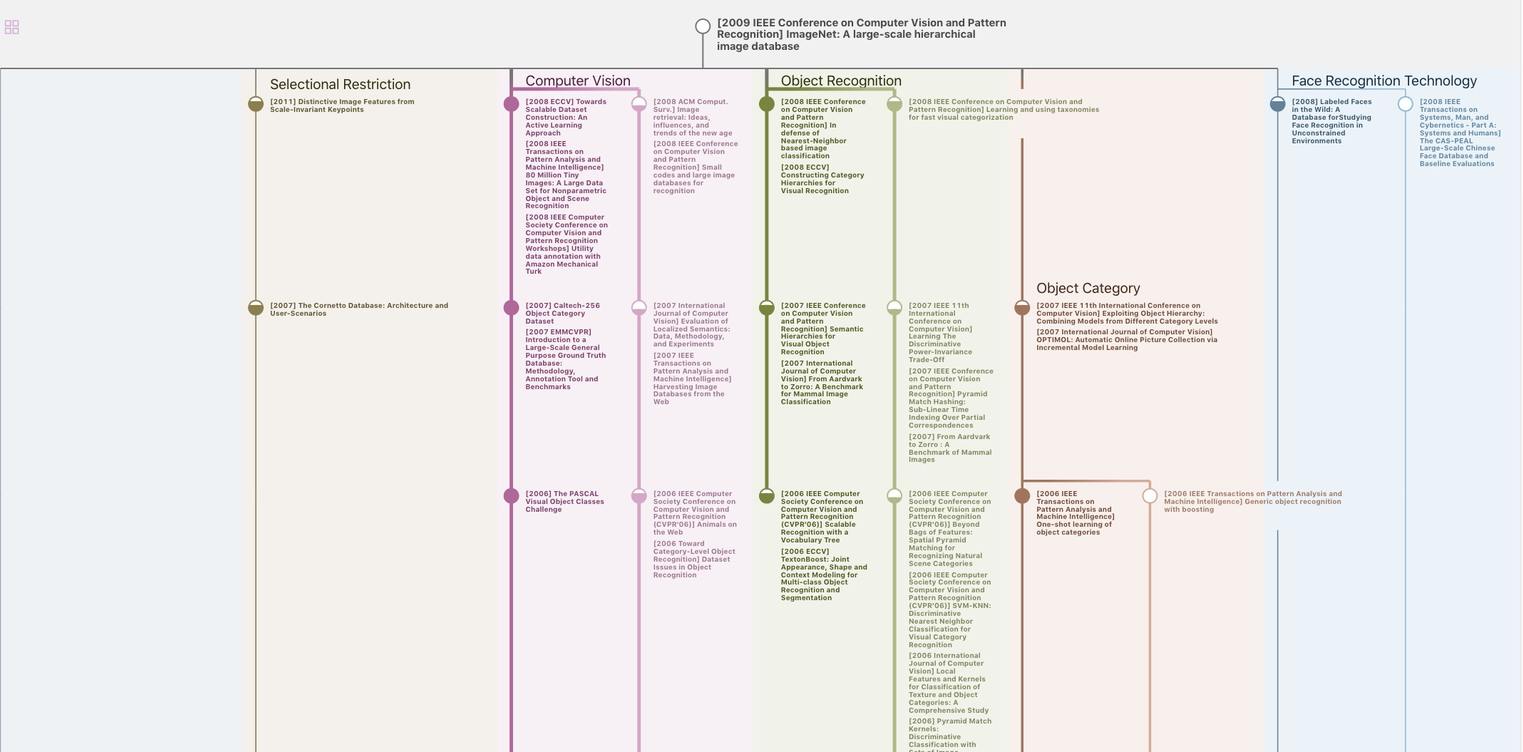
生成溯源树,研究论文发展脉络
Chat Paper
正在生成论文摘要