Gated Recurrent Unit-Based RNN for Remote Photoplethysmography Signal Segmentation
IEEE Conference on Computer Vision and Pattern Recognition(2022)
摘要
Remote Photoplethysmography (rPPG) enables quantifying blood volume variations in the skin tissues from an input video recording, using a regular RGB camera. Obtained pulse signals often contain noisy portions due to motion, leading researchers to put aside a great number of rPPG signals in their studies. In this paper, an approach using a Gated Recurrent Unit-based neural network model in order to identify reliable portions in rPPG signals is proposed. This is done by classifying rPPG signal samples into reliable and unreliable samples. For this purpose, rPPG and electrocardiography signals (ECG) were collected from 11 participants, rPPG signal samples were labeled (ECG was used as ground truth), and data were augmented to reach a total number of 11000 1-minute-long rPPG signals. We developed a model composed of a unidimensional CNN and a Bidirectional GRU (1D-CNN+B-GRU) for this study, and obtained an accuracy rate of 85.88%.
更多查看译文
关键词
rnn,segmentation,unit-based
AI 理解论文
溯源树
样例
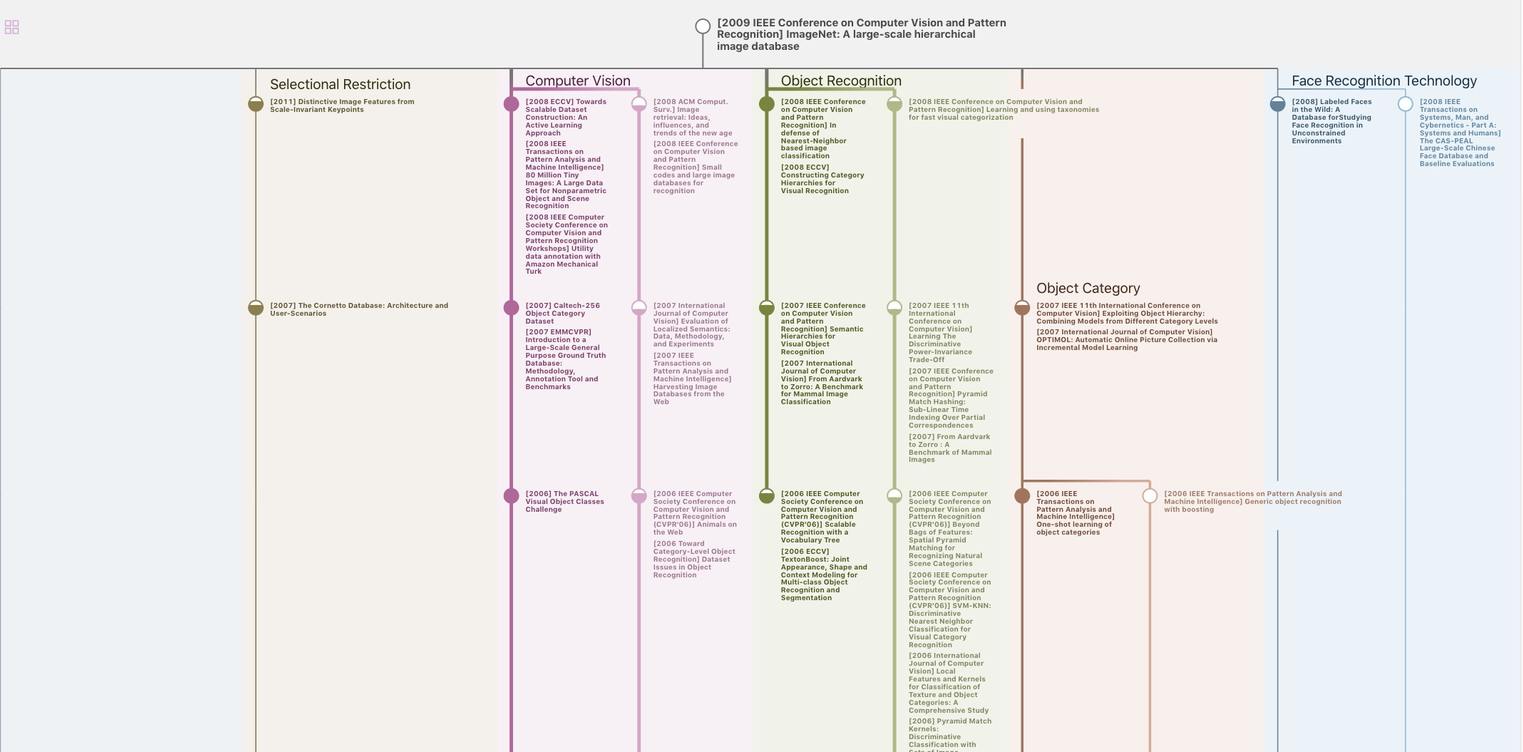
生成溯源树,研究论文发展脉络
Chat Paper
正在生成论文摘要