Model based control can give rise to devaluation insensitive choice
biorxiv(2022)
摘要
Influential recent work aims to ground psychiatric dysfunction in the brain’s basic computational mechanisms. For instance, compulsive symptoms as in drug abuse have been argued to arise from imbalance between multiple systems for instrumental learning. Computational models suggest that such multiplicity arises because the brain adaptively simplifies laborious “model-based” deliberation by sometimes relying on a cheaper, more habitual “model-free” shortcut. Support for this account comes in part from failures to appropriately change behavior in light of new events. Notably, instrumental responding can, in some circumstances, persist despite reinforcer devaluation, perhaps reflecting control by model-free mechanisms that are driven by past reinforcement rather than knowledge of the (now devalued) outcome. However, another important line of theory – heretofore mostly studied in Pavlovian conditioning – posits a different mechanism that can also modulate behavioral change. It concerns how animals identify different rules or contingencies that may apply in different circumstances, by covertly clustering experiences into distinct groups identified with different “latent causes” or contexts. Such clustering has been used to explain the return of Pavlovian responding following extinction.
Here we combine both lines of theory to investigate the consequences of latent cause inference on instrumental sensitivity to reinforcer devaluation. We show that because segregating events into different latent clusters prevents generalization between them, instrumental insensitivity to reinforcer devaluation can arise in this theory even using only model-based planning, and does not require or imply any habitual, model-free component. In simulations, these ersatz habits (like laboratory ones) emerge after overtraining, interact with contextual cues, and show preserved sensitivity to reinforcer devaluation on a separate consumption test, a standard control. While these results do not rule out a contribution of model-free learning per se , they point to a subtle and important role of state inference in instrumental learning and highlight the need for caution in using reinforcer devaluation procedures to rule in (or out) the contribution of different learning mechanisms. They also offer a new perspective on the neurocomputational substrates of drug abuse and the relevance of laboratory reinforcer devaluation procedures to this phenomenon.
### Competing Interest Statement
The authors have declared no competing interest.
更多查看译文
关键词
control,insensitive choice,devaluation,model
AI 理解论文
溯源树
样例
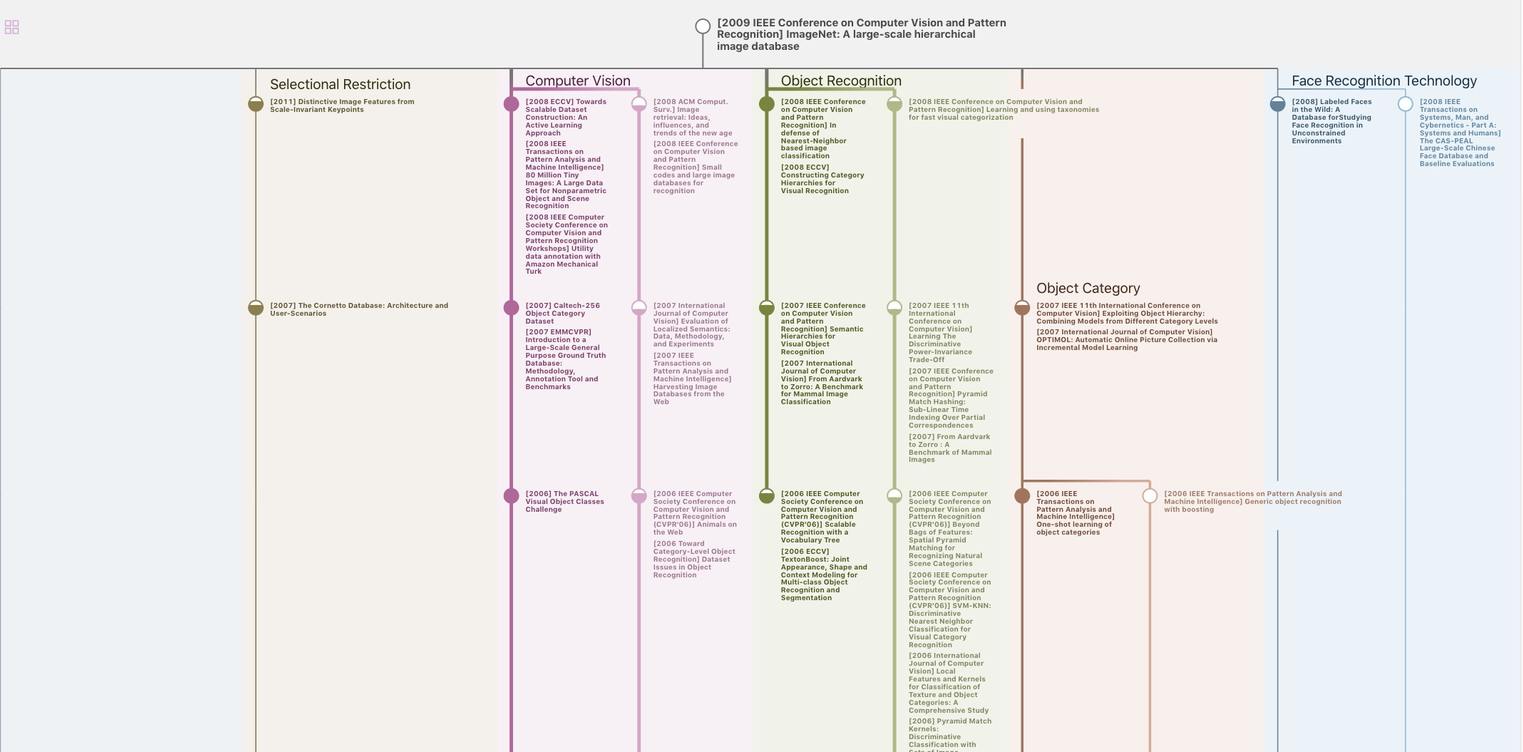
生成溯源树,研究论文发展脉络
Chat Paper
正在生成论文摘要