Antibody optimization enabled by artificial intelligence predictions of binding affinity and naturalness
biorxiv(2022)
摘要
Traditional antibody optimization approaches involve screening a small subset of the available sequence space, often resulting in drug candidates with suboptimal binding affinity, developability or immunogenicity. Based on two distinct antibodies, we demonstrate that deep contextual language models trained on high-throughput affinity data can quantitatively predict binding of unseen antibody sequence variants. These variants span a K D range of three orders of magnitude over a large mutational space. Our models reveal strong epistatic effects, which highlight the need for intelligent screening approaches. In addition, we introduce the modeling of “naturalness”, a metric that scores antibody variants for similarity to natural immunoglobulins. We show that naturalness is associated with measures of drug developability and immunogenicity, and that it can be optimized alongside binding affinity using a genetic algorithm. This approach promises to accelerate and improve antibody engineering, and may increase the success rate in developing novel antibody and related drug candidates.
### Competing Interest Statement
The authors are current or former employees, contractors or executives of Absci Corporation and may hold shares in Absci Corporation.
更多查看译文
AI 理解论文
溯源树
样例
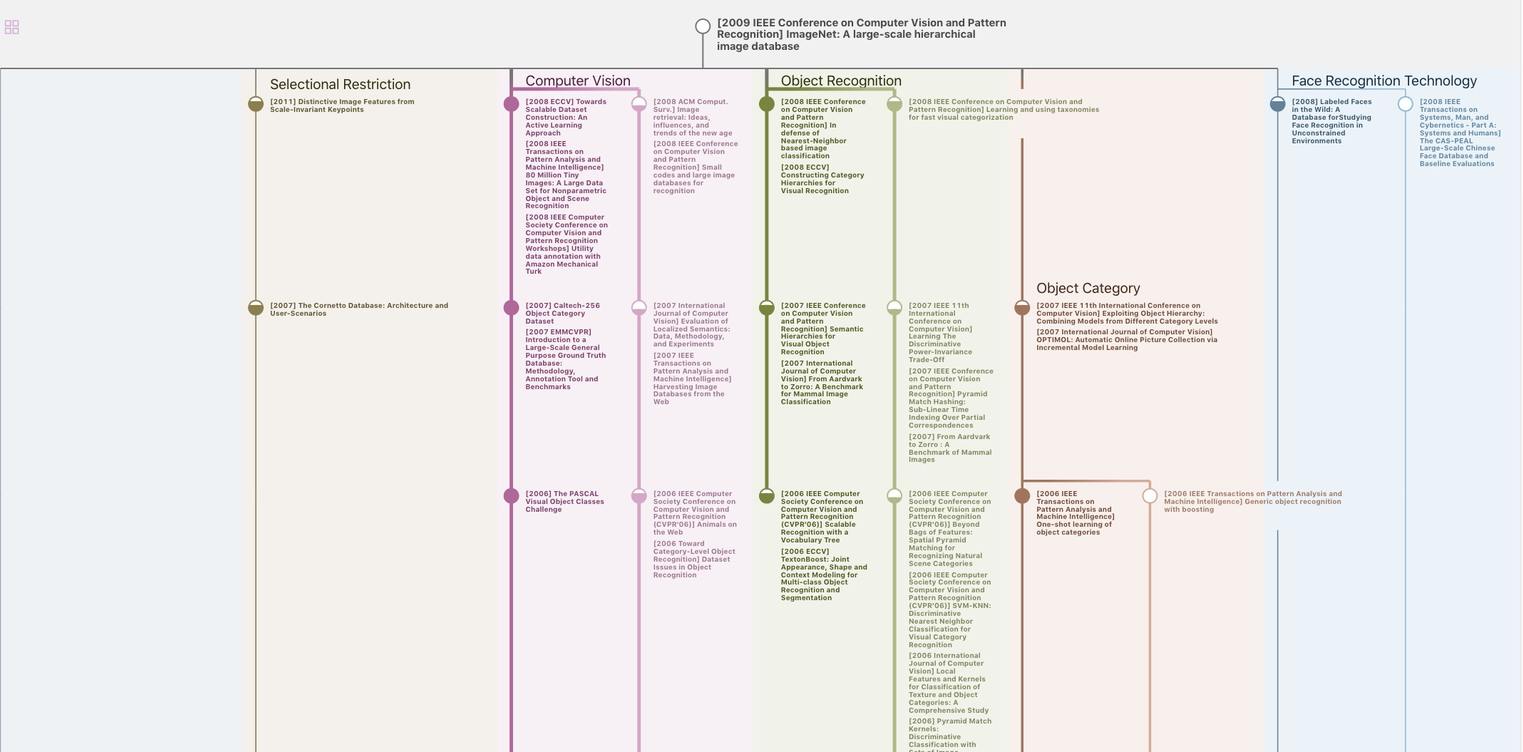
生成溯源树,研究论文发展脉络
Chat Paper
正在生成论文摘要