FairGBM: Gradient Boosting with Fairness Constraints
ICLR 2023(2022)
摘要
Machine Learning (ML) algorithms based on gradient boosted decision trees (GBDT) are still favored on many tabular data tasks across various mission critical applications, from healthcare to finance. However, GBDT algorithms are not free of the risk of bias and discriminatory decision-making. Despite GBDT's popularity and the rapid pace of research in fair ML, existing in-processing fair ML methods are either inapplicable to GBDT, incur in significant train time overhead, or are inadequate for problems with high class imbalance. We present FairGBM, a learning framework for training GBDT under fairness constraints with little to no impact on predictive performance when compared to unconstrained LightGBM. Since common fairness metrics are non-differentiable, we employ a "proxy-Lagrangian" formulation using smooth convex error rate proxies to enable gradient-based optimization. Additionally, our open-source implementation shows an order of magnitude speedup in training time when compared with related work, a pivotal aspect to foster the widespread adoption of FairGBM by real-world practitioners.
更多查看译文
关键词
fairness,gradient boosting,constrained optimization,tabular data
AI 理解论文
溯源树
样例
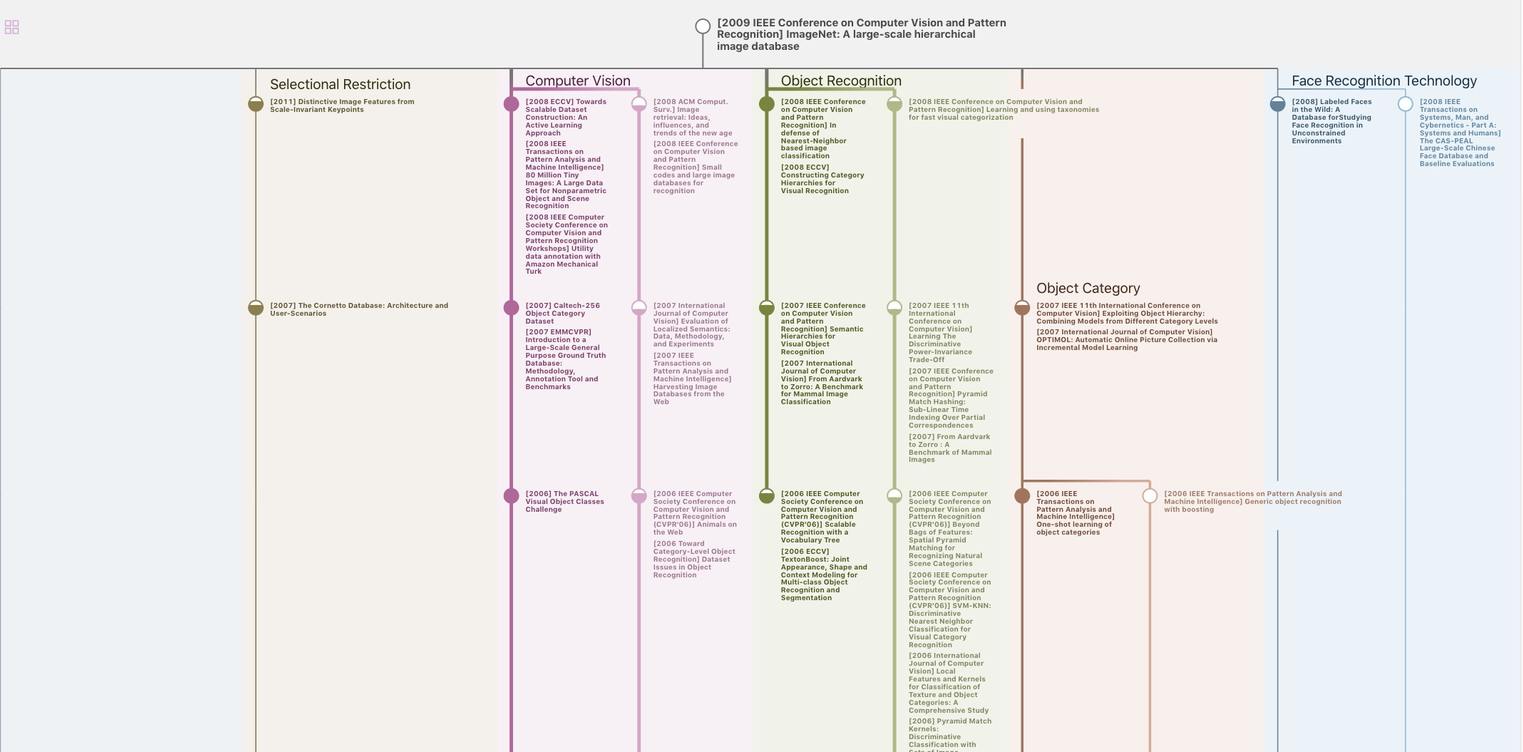
生成溯源树,研究论文发展脉络
Chat Paper
正在生成论文摘要