PointCAT: Contrastive Adversarial Training for Robust Point Cloud Recognition.
IEEE transactions on image processing : a publication of the IEEE Signal Processing Society(2024)
摘要
Notwithstanding the prominent performance shown in various applications, point cloud recognition models have often suffered from natural corruptions and adversarial perturbations. In this paper, we delve into boosting the general robustness of point cloud recognition, proposing Point-Cloud Contrastive Adversarial Training (PointCAT). The main intuition of PointCAT is encouraging the target recognition model to narrow the decision gap between clean point clouds and corrupted point clouds by devising feature-level constraints rather than logit-level constraints. Specifically, we leverage a supervised contrastive loss to facilitate the alignment and the uniformity of hypersphere representations, and design a pair of centralizing losses with dynamic prototype guidance to prevent features from deviating outside their belonging category clusters. To generate more challenging corrupted point clouds, we adversarially train a noise generator concurrently with the recognition model from the scratch. This differs from previous adversarial training methods that utilized gradient-based attacks as the inner loop. Comprehensive experiments show that the proposed PointCAT outperforms the baseline methods, significantly enhancing the robustness of diverse point cloud recognition models under various corruptions, including isotropic point noises, the LiDAR simulated noises, random point dropping, and adversarial perturbations. Our code is available at: https://github.com/shikiw/PointCAT.
更多查看译文
AI 理解论文
溯源树
样例
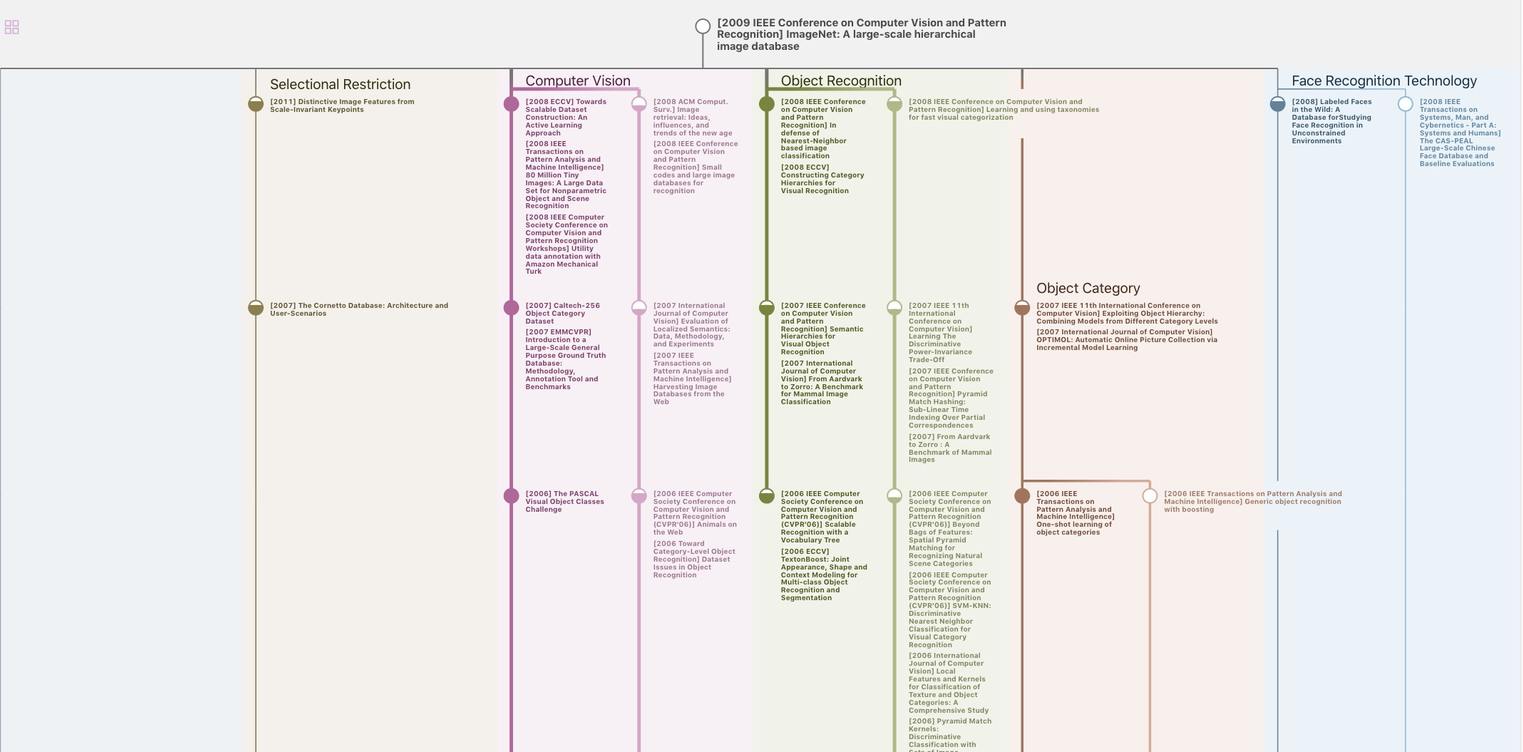
生成溯源树,研究论文发展脉络
Chat Paper
正在生成论文摘要