Antigen-Specific Antibody Design and Optimization with Diffusion-Based Generative Models for Protein Structures
NeurIPS 2022(2022)
摘要
Antibodies are immune system proteins that protect the host by binding to specific antigens such as viruses and bacteria. The binding between antibodies and antigens are mainly determined by the complementarity-determining regions (CDR) on the antibodies. In this work, we develop a deep generative model that jointly models sequences and structures of CDRs based on diffusion processes and equivariant neural networks. Our method is the first deep learning-based method that can explicitly target specific antigen structures and generate antibodies at atomic resolution. The model is a "Swiss Army Knife" which is capable of sequence-structure co-design, sequence design for given backbone structures, and antibody optimization. For antibody optimization, we propose a special sampling scheme that first perturbs the given antibody and then denoises it. As the number of available antibody structures is relatively scarce, we curate a new dataset that contains antibody-like proteins as a complement to the original antibody dataset for training. We conduct extensive experiments to evaluate the quality of both sequences and structures of designed antibodies. We find that our model could yield highly competitive results in terms of binding affinity measured by biophysical energy functions and other protein design metrics.
### Competing Interest Statement
The authors have declared no competing interest.
更多查看译文
关键词
antibody design,diffusion probabilistic model,protein structure
AI 理解论文
溯源树
样例
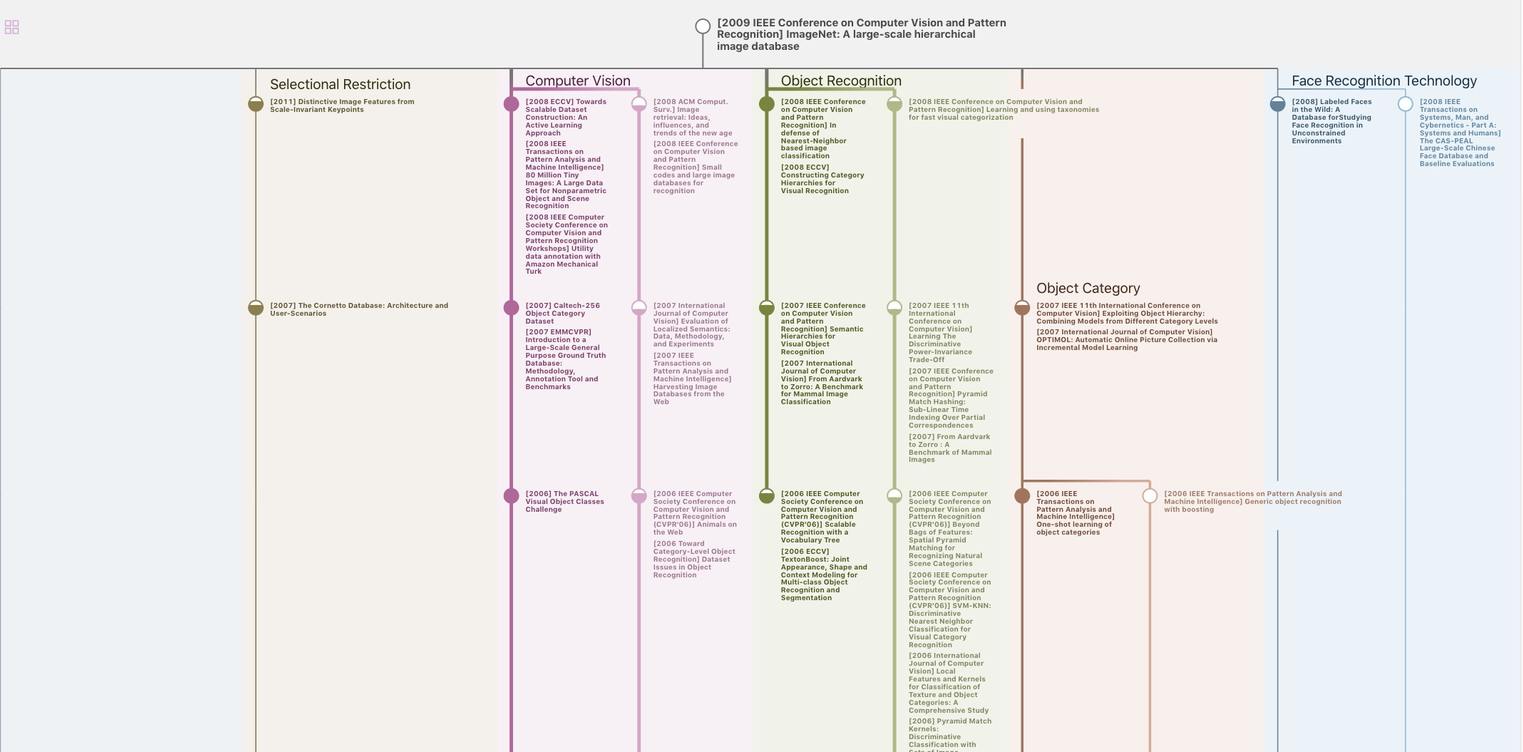
生成溯源树,研究论文发展脉络
Chat Paper
正在生成论文摘要