Characterizing The Landscape Of Viral Expression In Cancer By Deep Learning.
biorxiv(2022)
摘要
About 15% of human cancer cases are attributed to viral infections. To date, virus expression in tumor tissues has been mostly studied by aligning tumor RNA sequencing reads to databases of known viruses. To allow identification of divergent viruses and rapid characterization of the tumor virome, we developed viRNAtrap, an alignment-free pipeline to identify viral reads and assemble viral contigs. We apply viRNAtrap, which is based on a deep learning model trained to discriminate viral RNAseq reads, to 14 cancer types from The Cancer Genome Atlas (TCGA). We find that expression of exogenous cancer viruses is associated with better overall survival. In contrast, expression of human endogenous viruses is associated with worse overall survival. Using viRNAtrap, we uncover expression of unexpected and divergent viruses that have not previously been implicated in cancer. The viRNAtrap pipeline provides a way forward to study viral infections associated with different clinical conditions.
### Competing Interest Statement
The authors have declared no competing interest.
更多查看译文
关键词
viral expression,cancer,deep,learning
AI 理解论文
溯源树
样例
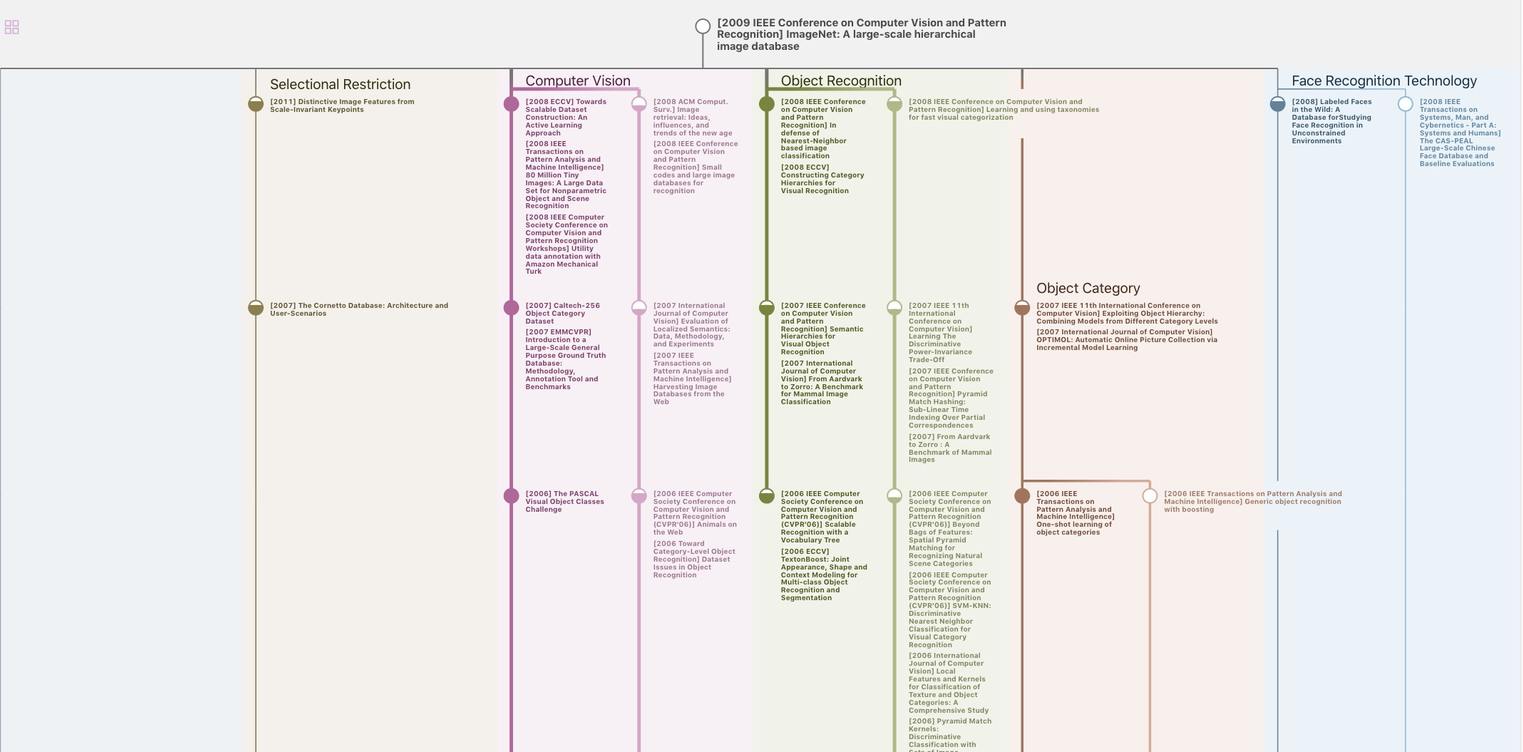
生成溯源树,研究论文发展脉络
Chat Paper
正在生成论文摘要