Computer vision models for comparing spatial patterns: understanding spatial scale
INTERNATIONAL JOURNAL OF GEOGRAPHICAL INFORMATION SCIENCE(2023)
摘要
Comparison of landscapes and patterns is a long-standing challenge in spatial analysis research. Recently, new models and tools developed for non-geographic image data are being used to study geographic problems involving classification or prediction. Specifically, computer vision models and artificial neural networks have been deployed in an ever-growing number of geographical analyses. In this paper, we review the use of these models in geographical analysis, focusing on the representation and comparison of spatial patterns. We review artificial neural networks and provide semantic linking across domains using similar model constructs through the lens of scale. We note that scale, a contextual element in geographical research, is typically considered a model parameter in computer vision. Scale impacts both computer vision techniques and traditional pixel-based or object-oriented analysis, yet computer vision methods such as CNNs are relatively robust to small-scale variations due to their capability to learn multiscale features via spatial filtering and the formation of scale-space tensors across layers. Parameterization of computer vision models to represent multiscale patterns however remains ad hoc. A typology of scales, therefore, provides a framework for mapping model constructs to develop guidelines for parameterizing and evaluating computer vision models in a geographic context.
更多查看译文
关键词
Computer vision, pattern comparison, scale, convolutional neural networks
AI 理解论文
溯源树
样例
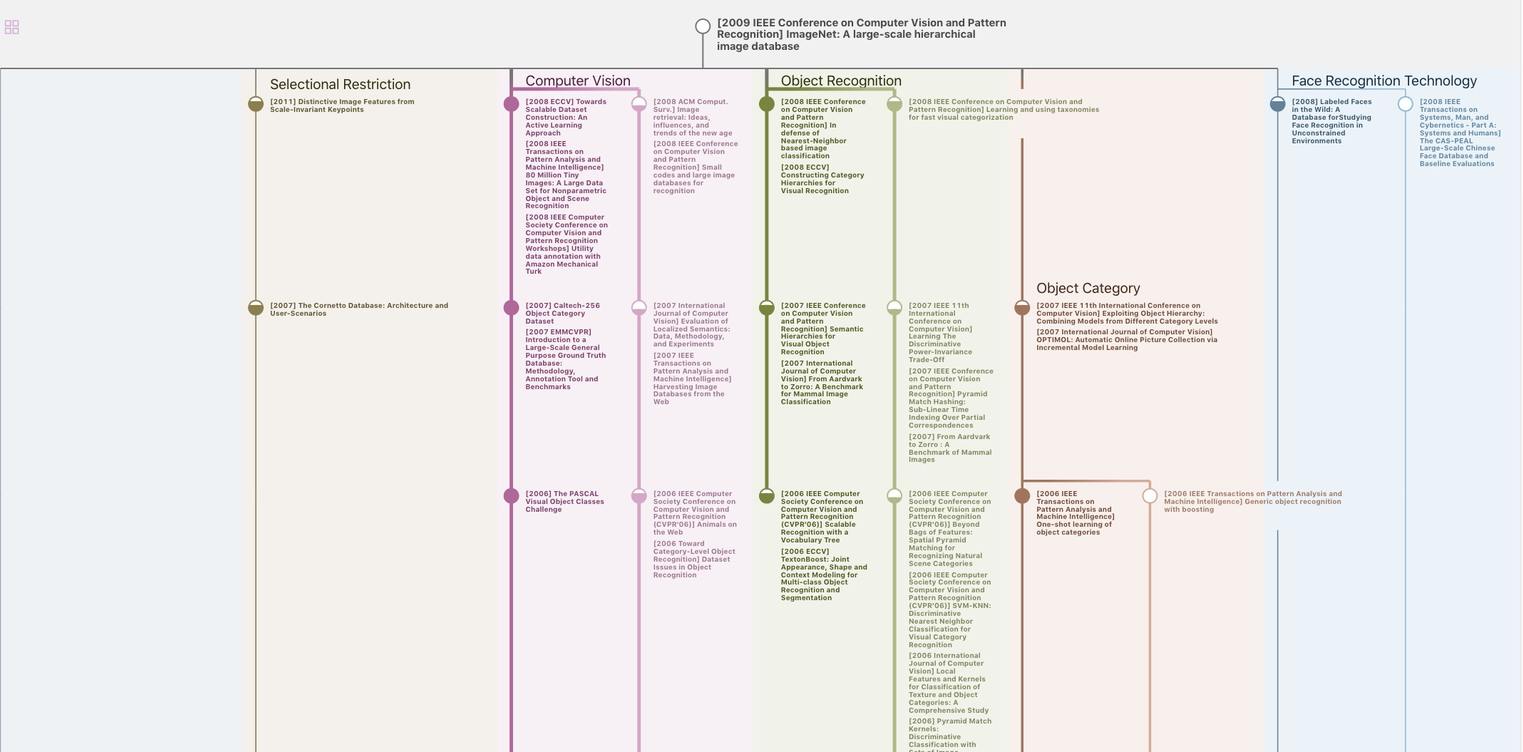
生成溯源树,研究论文发展脉络
Chat Paper
正在生成论文摘要