A Comprehensive Framework for Long-Tailed Learning via Pretraining and Normalization
IEEE TRANSACTIONS ON NEURAL NETWORKS AND LEARNING SYSTEMS(2024)
摘要
Data in the visual world often present long-tailed distributions. However, learning high-quality representations and classifiers for imbalanced data is still challenging for data-driven deep learning models. In this work, we aim at improving the feature extractor and classifier for long-tailed recognition via contrastive pretraining and feature normalization, respectively. First, we carefully study the influence of contrastive pretraining under different conditions, showing that current self-supervised pretraining for long-tailed learning is still suboptimal in both performance and speed. We thus propose a new balanced contrastive loss and a fast contrastive initialization scheme to improve previous long-tailed pretraining. Second, based on the motivative analysis on the normalization for classifier, we propose a novel generalized normalization classifier that consists of generalized normalization and grouped learnable scaling. It outperforms traditional inner product classifier as well as cosine classifier. Both the two components proposed can improve recognition ability on tail classes without the expense of head classes. We finally build a unified framework that achieves competitive performance compared with state of the arts on several long-tailed recognition benchmarks and maintains high efficiency.
更多查看译文
关键词
Training,Tail,Task analysis,Head,Visualization,Feature extraction,Data models,Classifier design,contrastive learning,long-tailed recognition,normalization
AI 理解论文
溯源树
样例
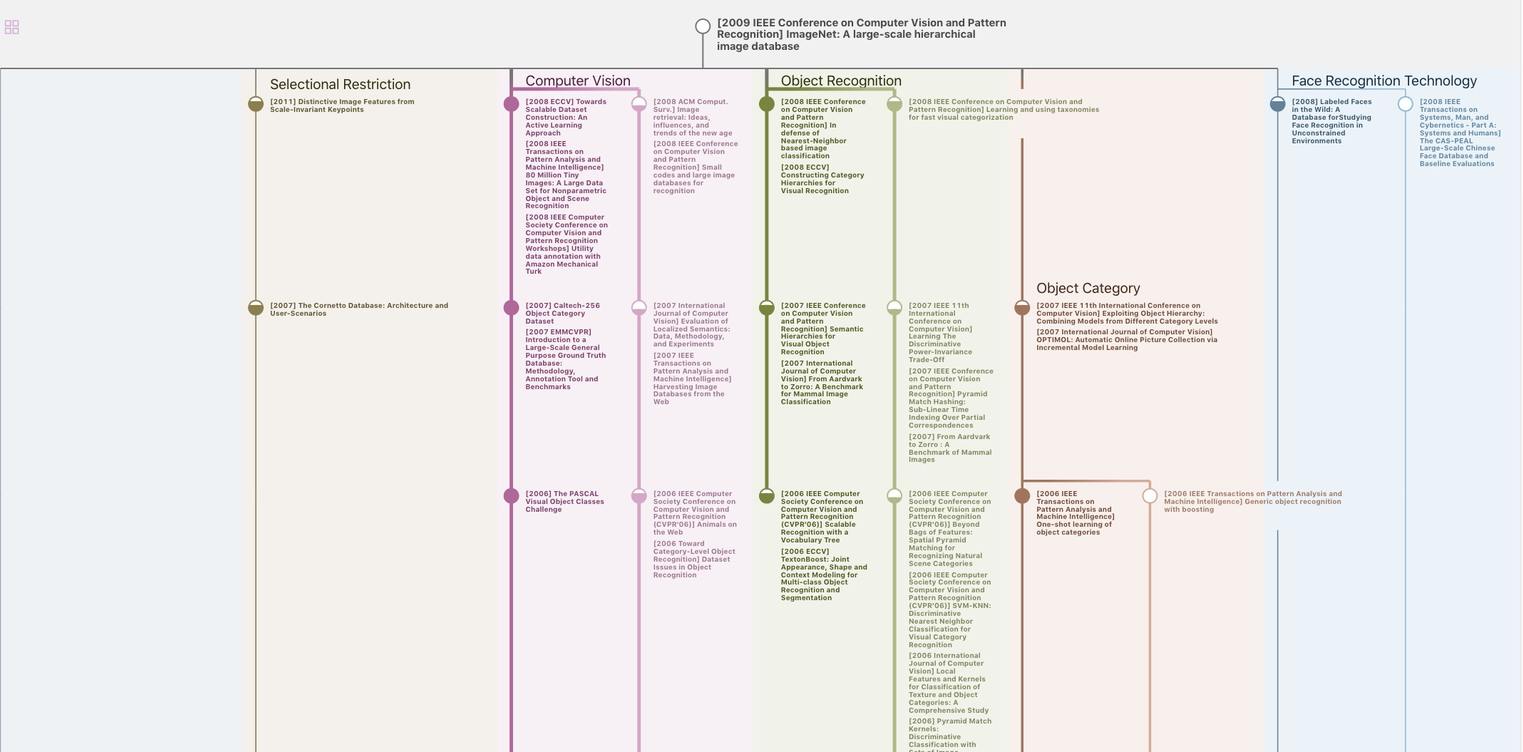
生成溯源树,研究论文发展脉络
Chat Paper
正在生成论文摘要