A2AE: Towards adaptive multi-view graph representation learning via all-to-all graph autoencoder architecture
Applied Soft Computing(2022)
摘要
The multi-view graph is a fundamental data model, which is used to describe complex networks in the real world. Learning the representation of multi-view graphs is a vital step for understanding complex systems and extracting knowledge accurately. However, most existing methods focus on a certain view or simply add multiple views together, which prevents them from making the best of the rich relational information in multiple views and ignores the importance of different views. In this paper, a novel all-to-all graph autoencoder is proposed for multi-view graph representation learning, namely A2AE. The all-to-all model first embeds the attribute multi-view graph into compact representations by semantic fusing the view-specific compact representations from multi-encoders, and then multi-decoders are trained to reconstruct graph structure and attributes. Finally, a self-training clustering module is attached for clustering tasks.
更多查看译文
关键词
Multi-view graph,Graph autoencoder,Network embedding,Graph neural networks
AI 理解论文
溯源树
样例
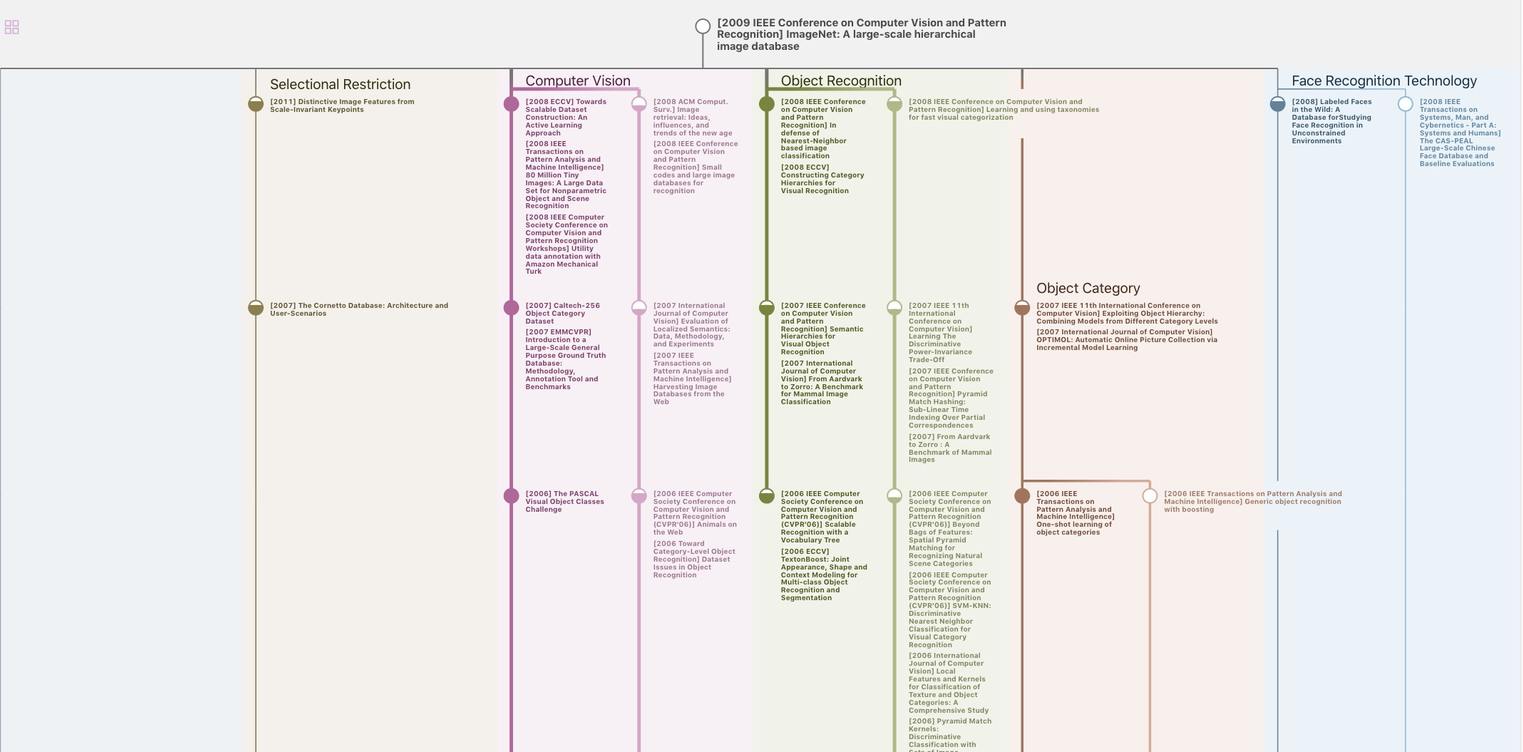
生成溯源树,研究论文发展脉络
Chat Paper
正在生成论文摘要