High-efficient hierarchical federated learning on non-IID data with progressive collaboration
Future Generation Computer Systems(2022)
摘要
Hierarchical federated learning (HFL) allows multiple edge aggregations at edge devices before one global aggregation to address both issues of non-independent and identically distributed (non-IID) data and communication bottleneck in federated learning (FL). To solve the non-IID issue, most HFL algorithms assume that clients can be assigned to any edge device. In practice, however, these assumptions are always unrealistic. In this paper, we propose a high-efficient HFL algorithm, named FedPEC, which introduces progressive edge collaboration rather than unrealistic client allocation. FedPEC estimates the initial number of collaborators based on our proved convergence upper bound, and then constantly adjusts the estimated number of collaborators according to the characteristics of each stage in the following rounds. Guided by the estimated number of collaborators, each edge device can be assigned an appropriate collaborator set based on an adaptive similarity threshold. Extensive experiments are conducted to investigate FedPEC in terms of accuracy, loss, and convergence speed with various data sets. Our experimental results demonstrate that FedPEC can significantly outperform state-of-the-art FL algorithms.
更多查看译文
关键词
Federated learning,Hierarchical architecture,Non-IID data,Edge collaboration,Optimization,Model training efficiency
AI 理解论文
溯源树
样例
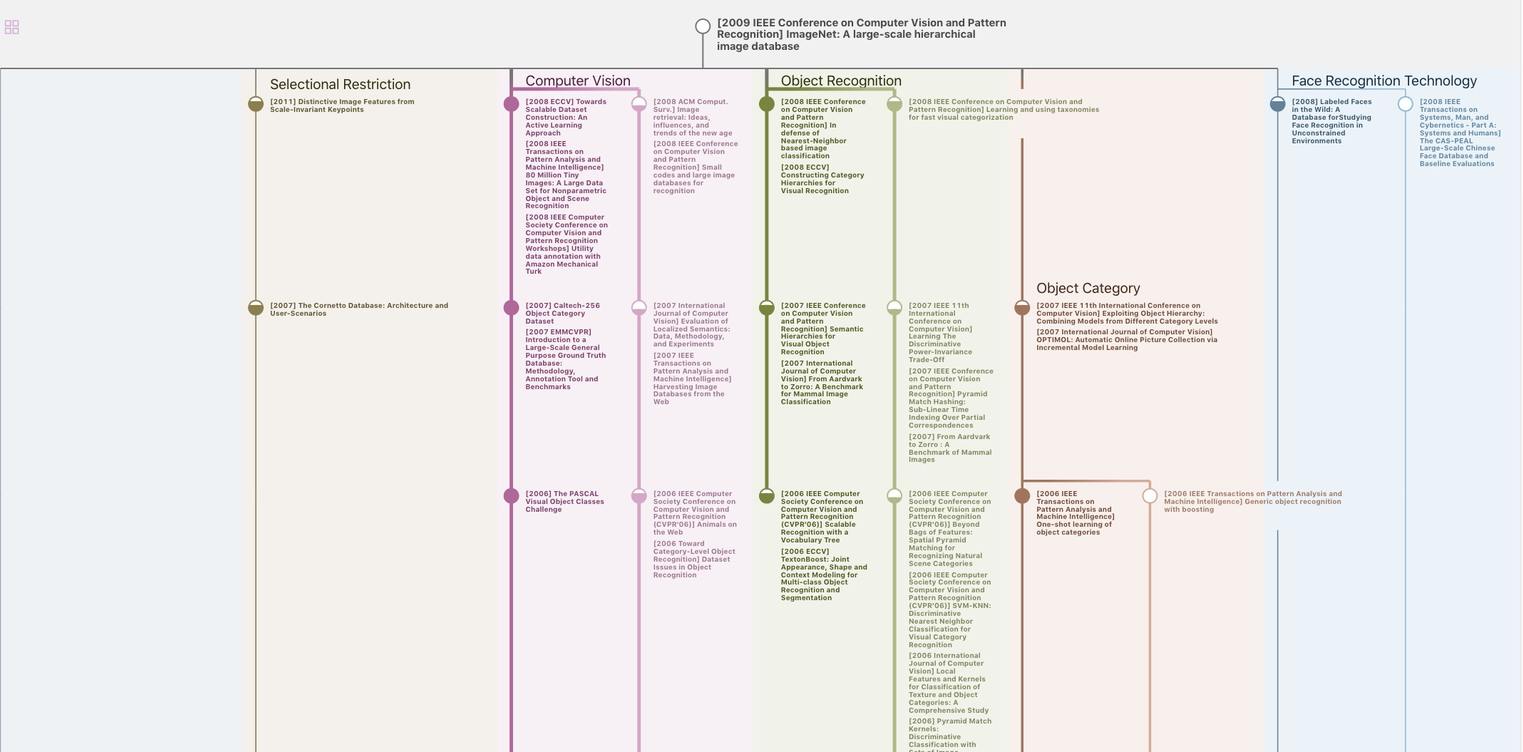
生成溯源树,研究论文发展脉络
Chat Paper
正在生成论文摘要