Performance Improvement of Image-Reconstruction-Based Defense against Adversarial Attack
ELECTRONICS(2022)
摘要
Deep Neural Networks (DNNs) used for image classification are vulnerable to adversarial examples, which are images that are intentionally generated to predict an incorrect output for a deep learning model. Various defense methods have been proposed to defend against such adversarial attacks, among which, image-reconstruction-based defense methods, such as DIPDefend, are known to be effective in getting rid of the adversarial perturbations injected in the image. However, this image-reconstruction-based defense approach suffers from a long execution time due to its iterative and time-consuming image reconstruction. The trade-off between the execution time and the robustness/accuracy of the defense method should be carefully explored, which is the main focus of this paper. In this work, we aim to improve the execution time of the existing state-of-the-art image-reconstruction-based defense method, DIPDefend, against the Fast Gradient Sign Method (FGSM). In doing so, we propose to take the input-specific properties into consideration when deciding the stopping point of the image reconstruction of DIPDefend. For that, we first applied a low-pass filter to the input image with various kernel sizes to make a prediction of the true label. Then, based on that, the parameters of the image reconstruction procedure were adaptively chosen. Experiments with 500 randomly chosen ImageNet validation set images show that we can obtain an approximately 40% improvement in execution time while keeping the accuracy drop as small as 0.4-3.9%.
更多查看译文
关键词
adversarial attack, adversarial example, image reconstruction, deep image prior, neural network
AI 理解论文
溯源树
样例
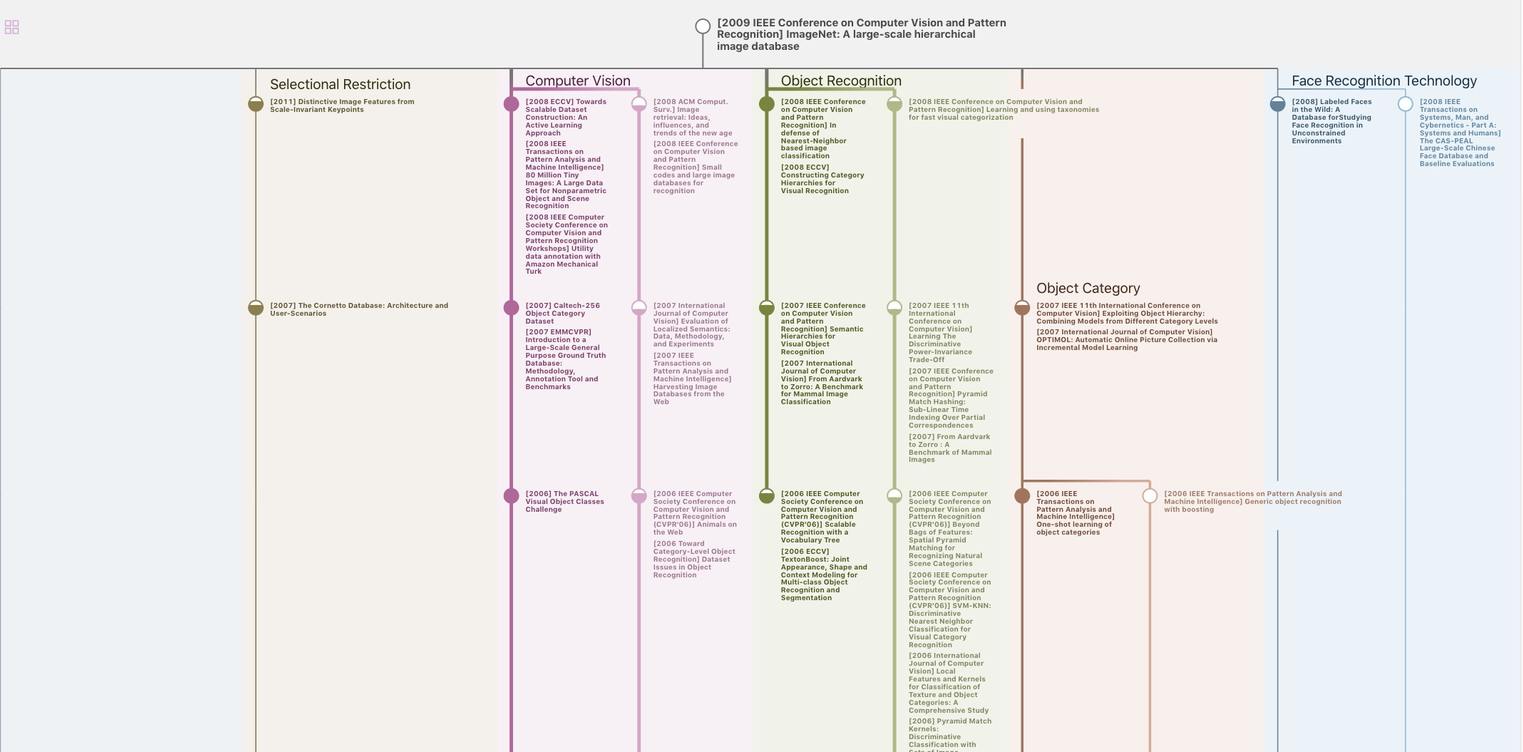
生成溯源树,研究论文发展脉络
Chat Paper
正在生成论文摘要