Machine Learning Methods to Analyze and Predict Crash Injury Severity Based on Contributing Factors for Southeast Michigan
TRANSPORTATION RESEARCH RECORD(2023)
摘要
Traffic safety is a critical aspect of transportation. Transportation agencies and researchers have been continuously making an effort to ensure minimal error margins in the road system. There is a growing interest in the Southeast Michigan region because of the active role of original equipment manufacturers in spurring research in the new mobility field and autonomous vehicle technology. As new jobs are created and commuting trips increase, traffic safety becomes even more important. Thus, this study presents one of the first efforts to study crash patterns for Southeast Michigan using machine learning models to analyze and predict crash severity in the region based on various contributing factors. We combine a three-step method of correlation analysis, machine learning predictive models, and spatial analysis to develop a rigid schema for crash prediction. Results show that decision tree classifiers provide accurate predictions and rapid computations. Also, spatial plots of predicted injury severities reveal disproportionate errors in model predictions at intersections prompting the need to stratify the crash data for further analyses. This significantly improves severe injury predictions at non-intersection locations and highlights pedestrians as a main important feature. The converse holds for intersections that identify motorcycles as the main feature for severity prediction. Further results are elucidated in this paper.
更多查看译文
关键词
data and data science, data analysis, safety, crash analysis, crash data, crash prediction models
AI 理解论文
溯源树
样例
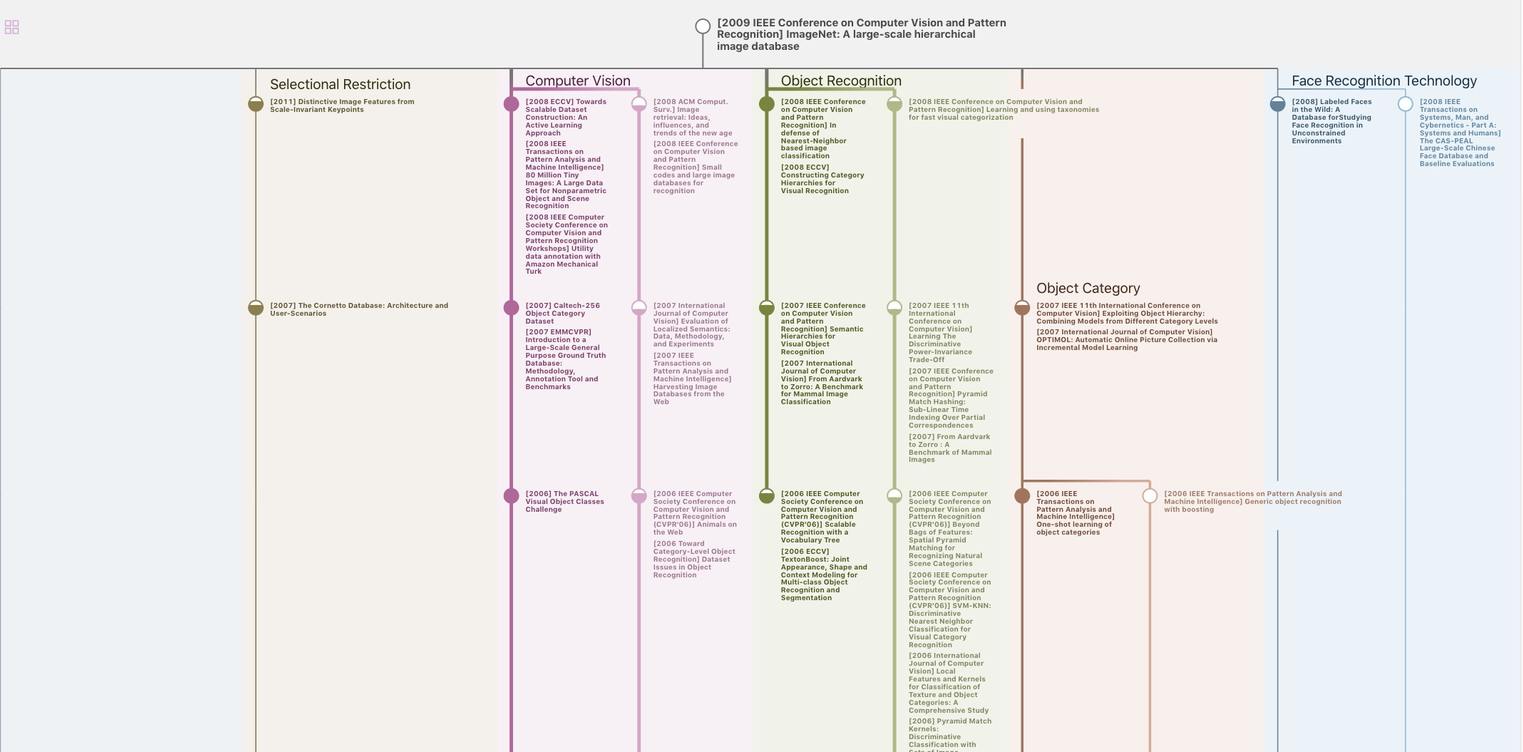
生成溯源树,研究论文发展脉络
Chat Paper
正在生成论文摘要