Quantitative Trading through Random Perturbation Q-Network with Nonlinear Transaction Costs
STATS(2022)
摘要
In recent years, reinforcement learning (RL) has seen increasing applications in the financial industry, especially in quantitative trading and portfolio optimization when the focus is on the long-term reward rather than short-term profit. Sequential decision making and Markov decision processes are rather suited for this type of application. Through trial and error based on historical data, an agent can learn the characteristics of the market and evolve an algorithm to maximize the cumulative returns. In this work, we propose a novel RL trading algorithm utilizing random perturbation of the Q-network and account for the more realistic nonlinear transaction costs. In summary, we first design a new near-quadratic transaction cost function considering the slippage. Next, we develop a convolutional deep Q-learning network (CDQN) with multiple price input based on this cost functions. We further propose a random perturbation (rp) method to modify the learning network to solve the instability issue intrinsic to the deep Q-learning network. Finally, we use this newly developed CDQN-rp algorithm to make trading decisions based on the daily stock prices of Apple (AAPL), Meta (FB), and Bitcoin (BTC) and demonstrate its strengths over other quantitative trading methods.
更多查看译文
关键词
deep reinforcement learning, Markov decision process, quantitative finance, random perturbation algorithm, transaction costs model
AI 理解论文
溯源树
样例
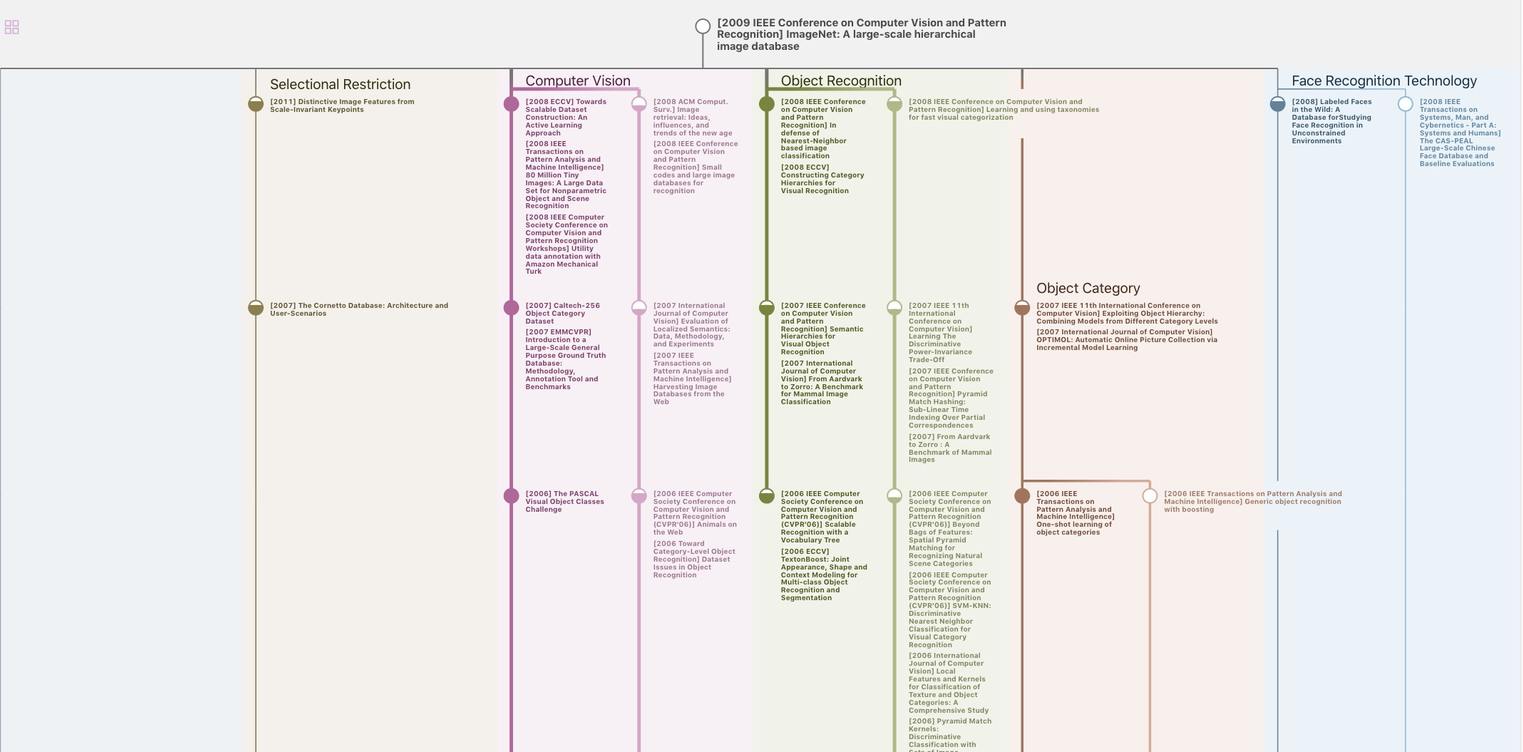
生成溯源树,研究论文发展脉络
Chat Paper
正在生成论文摘要