Unsupervised learning of multi-task deep variational model
Journal of Visual Communication and Image Representation(2022)
摘要
We propose a general deep variational model (reduced version, full version as well as the extension) via a comprehensive fusion approach in this paper. It is able to realize various image tasks in a completely unsupervised way without learning from samples. Technically, it can properly incorporate the CNN based deep image prior (DIP) architecture into the classic variational image processing models. The minimization problem solving strategy is transformed from iteratively minimizing the sub-problem for each variable to automatically minimizing the loss function by learning the generator network parameters. The proposed deep variational (DV) model contributes to the high order image edition and applications such as image restoration, inpainting, decomposition and texture segmentation. Experiments conducted have demonstrated significant advantages of the proposed deep variational model in comparison with several powerful techniques including variational methods and deep learning approaches.
更多查看译文
关键词
Unsupervised learning,Integration approach,Deep neural networks,Variational general frameworks,Diverse applications
AI 理解论文
溯源树
样例
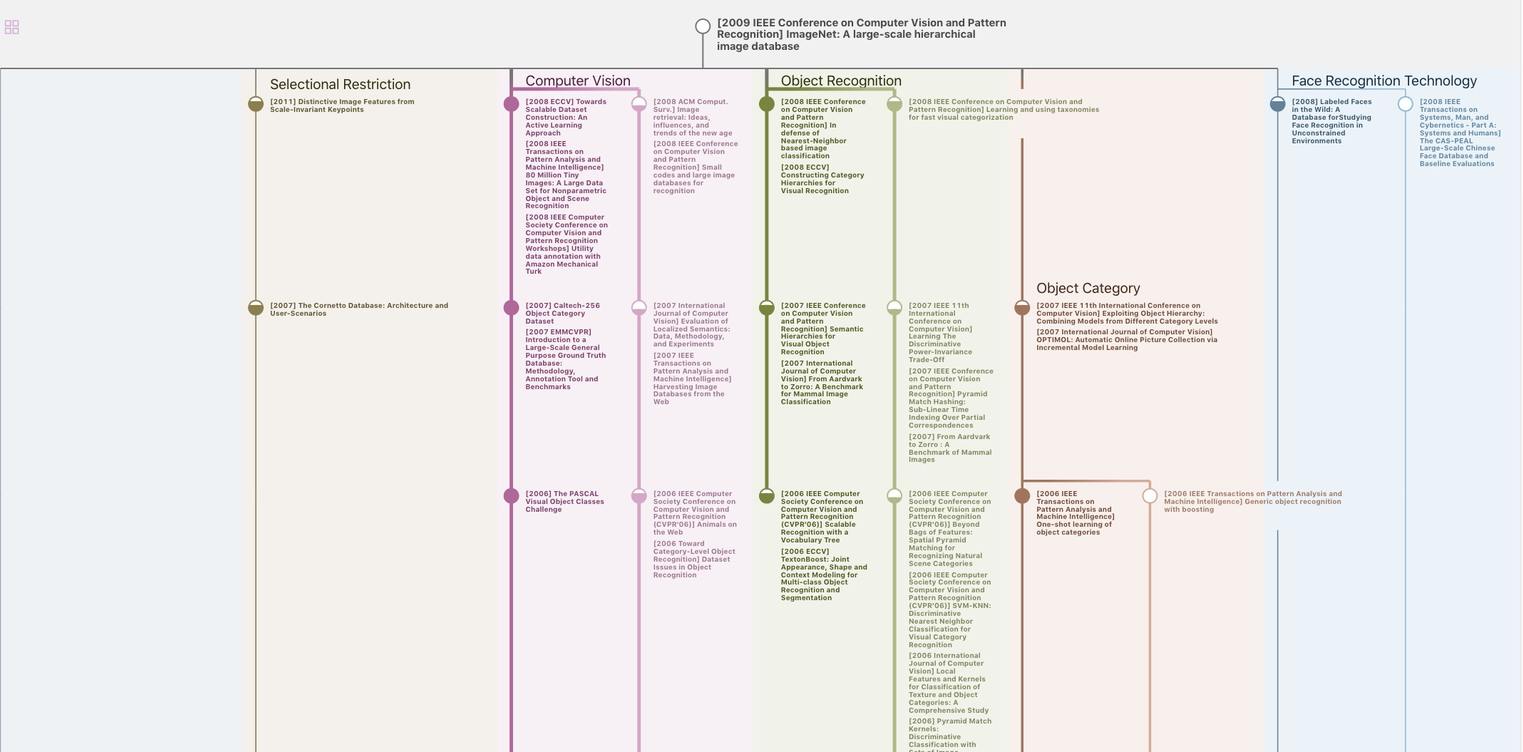
生成溯源树,研究论文发展脉络
Chat Paper
正在生成论文摘要