Influences of vegetation, model, and data parameters on forest aboveground biomass assessment using an area-based approach
Ecological Informatics(2022)
摘要
Although aboveground biomass (AGB) estimation using area-based approaches (ABAs) and its application to forestry have been actively researched through three decades, this technology has been little operationalized in the Central European forest sector. That means specific recommendations are needed in order to apply ABA for forest biomass modelling in this region. The present study was directed to filling such gaps while examining the effect of input ABA parameters on AGB model quality in conditions of mixed mountainous forests in Central Europe. Specific objectives were to assess whether the strength of the AGB model can be impacted by 1) canopy conditions (leaf-on and leaf-off), 2) airborne LiDAR point density (2.5, 5.0, 7.5, 10.0 points/m2), 3) field methods to estimate AGB (with regeneration components or without), and 4) machine learning methods (AdaBoost, Random decision forest, multilayer neural network, and Bayesian ridge regression). The results show that canopy conditions and airborne LiDAR point densities did not affect the strength of the AGB model, but that model's strength was affected by the vegetation regeneration component in the field biomass reference and by the machine learning method tested for modelling. AdaBoost and random decision forest were the most successful methods. To evaluate the quality of an AGB model it is recommended to combine several individual evaluation functions into the model score. The study highlights several recommendations to follow when estimating AGB from ALS using an ABA in Central European forests.
更多查看译文
关键词
Airborne laser scanning,Machine learning,Model score,Mixed forest,Central Europe
AI 理解论文
溯源树
样例
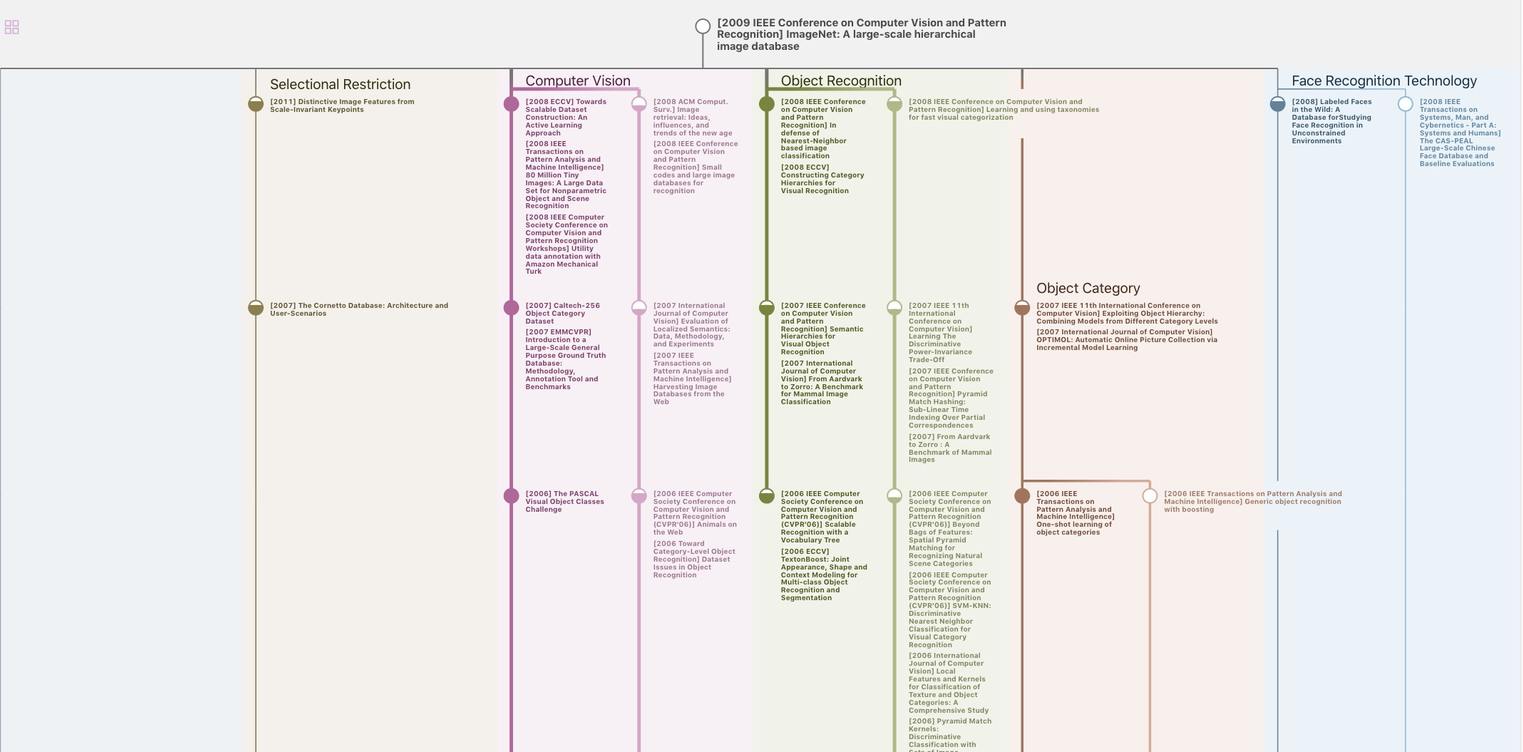
生成溯源树,研究论文发展脉络
Chat Paper
正在生成论文摘要