Reinforcement Learning-Based Adaptive Biofeedback Engine for Overground Walking Speed Training
IEEE ROBOTICS AND AUTOMATION LETTERS(2022)
摘要
Wearable biofeedback systems (WBS) have been proposed to aid physical rehabilitation of individuals with motor impairments. Due to significant inter- and intra-individual differences, the effectiveness of a given biofeedback strategy may vary for different users and across therapeutic sessions, as a patient's functional recovery progresses. To date, only a paucity of research has investigated the use of biofeedback strategies that can self-adapt based on the user's response. This letter introduces a novel reinforcement learning with fuzzy logic biofeedback engine (RLFLE) for personalized overground walking speed training. The method leverages reinforcement learning and a fuzzy inference strategy to continuously modulate underfoot vibrotactile stimuli that encourage users to achieve a target walking speed. This stimulation strategy also enables the determination of a user's maximum steady-state walking speed during a gait training session overground. The RLFLE was implemented in a custom-engineered WBS and validated against two simpler biofeedback strategies during walking tests with healthy adults. Participants showed lower walking speed errors when training with the RLFLE. Additionally, results indicate that the new method is more effective in determining an individual's maximum steady-state walking speed. Given the importance of walking speed as an indicator of health status and as an essential outcome of exercise-based interventions, these results show promise for implementation in future technology-enhanced gait rehabilitation protocols.
更多查看译文
关键词
Wearable biofeedback system, instrumented footwear, human-in-the-loop, reinforcement learning, fuzzy logic, gait training
AI 理解论文
溯源树
样例
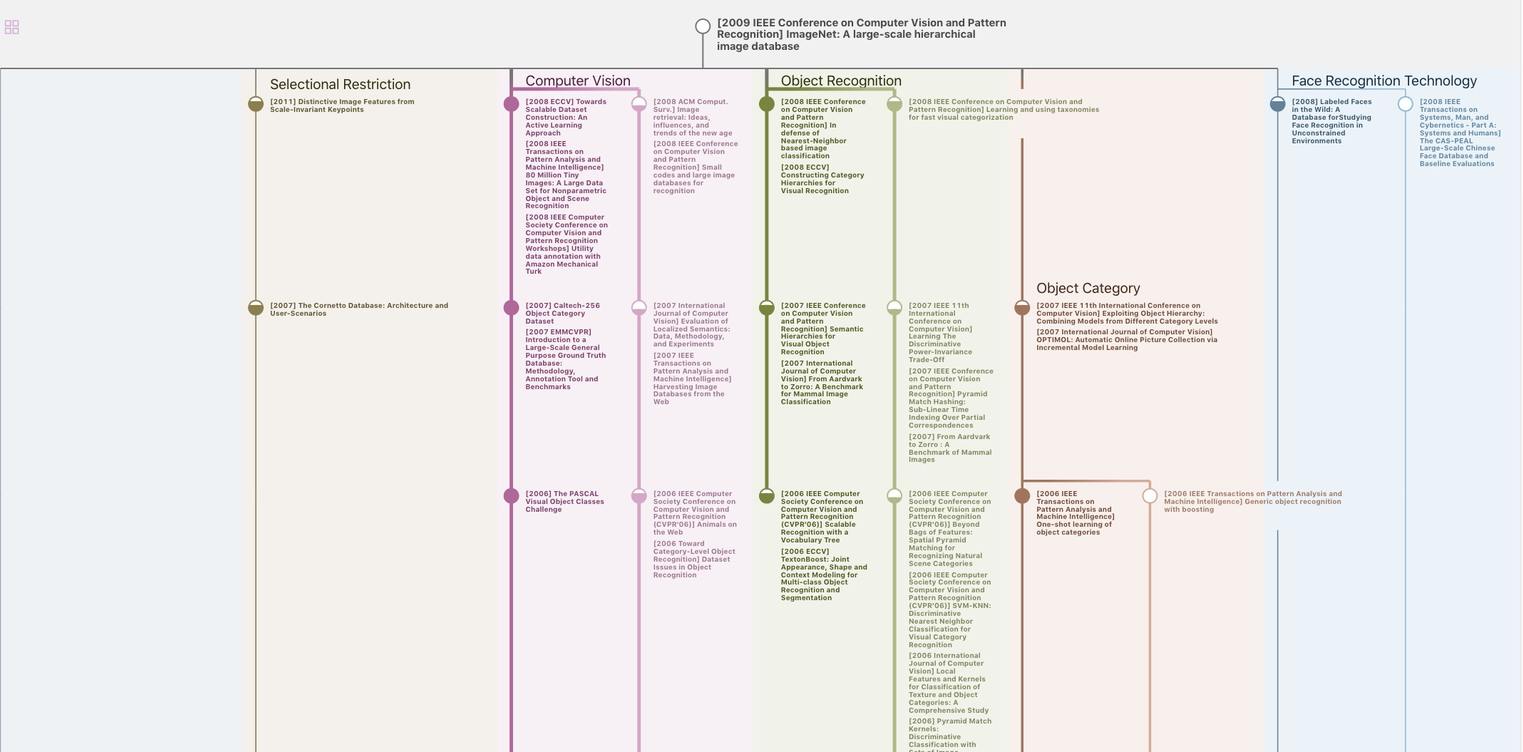
生成溯源树,研究论文发展脉络
Chat Paper
正在生成论文摘要