A feasibility study of computer-aided diagnosis with DECT Bayesian reconstruction for polyp classification
MEDICAL IMAGING 2022: COMPUTER-AIDED DIAGNOSIS(2022)
摘要
Dual-energy computed tomography (DECT) has emerged as a promising imaging modality in the field of clinical diagnosis, which expands applications of CT imaging in its capability to acquire two datasets, one at high and the other at low energy. With the Bayesian reconstruction directly from projection measurements at two energies, the energy-independent densities of the two basis materials (e.g. bone/soft-tissue) of the scanned objects are obtained. This work investigated the feasibility of the computer-aided diagnosis with DECT Bayesian reconstruction (CADxDE) for polyp classification. Specifically, the above-reconstructed density images could generate a series of pseudo-single energy CT images multiplied with the corresponding mass attenuation coefficients at selected n energies. Given the augmented n-energy CT images, we proposed a convolution neural network (CNN) based CADx model to differentiate malignant from benign polyps by recognizing material features at different energies. The dataset consists of 63 polyp masses from fifty-nine patients were carried out to verify our CADxDE model. The classification results showed that the area under the receiver operating characteristic curve (AUC) score can be improved by 12.17% with CADxDE over the conventional single energy data only. This feasibility study indicates it is promising that the computer-aided diagnosis with DECT Bayesian reconstruction could be used to improve the clinical classification performance.
更多查看译文
关键词
DECT,CADx,Bayesian Reconstruction,Polyp differentiation
AI 理解论文
溯源树
样例
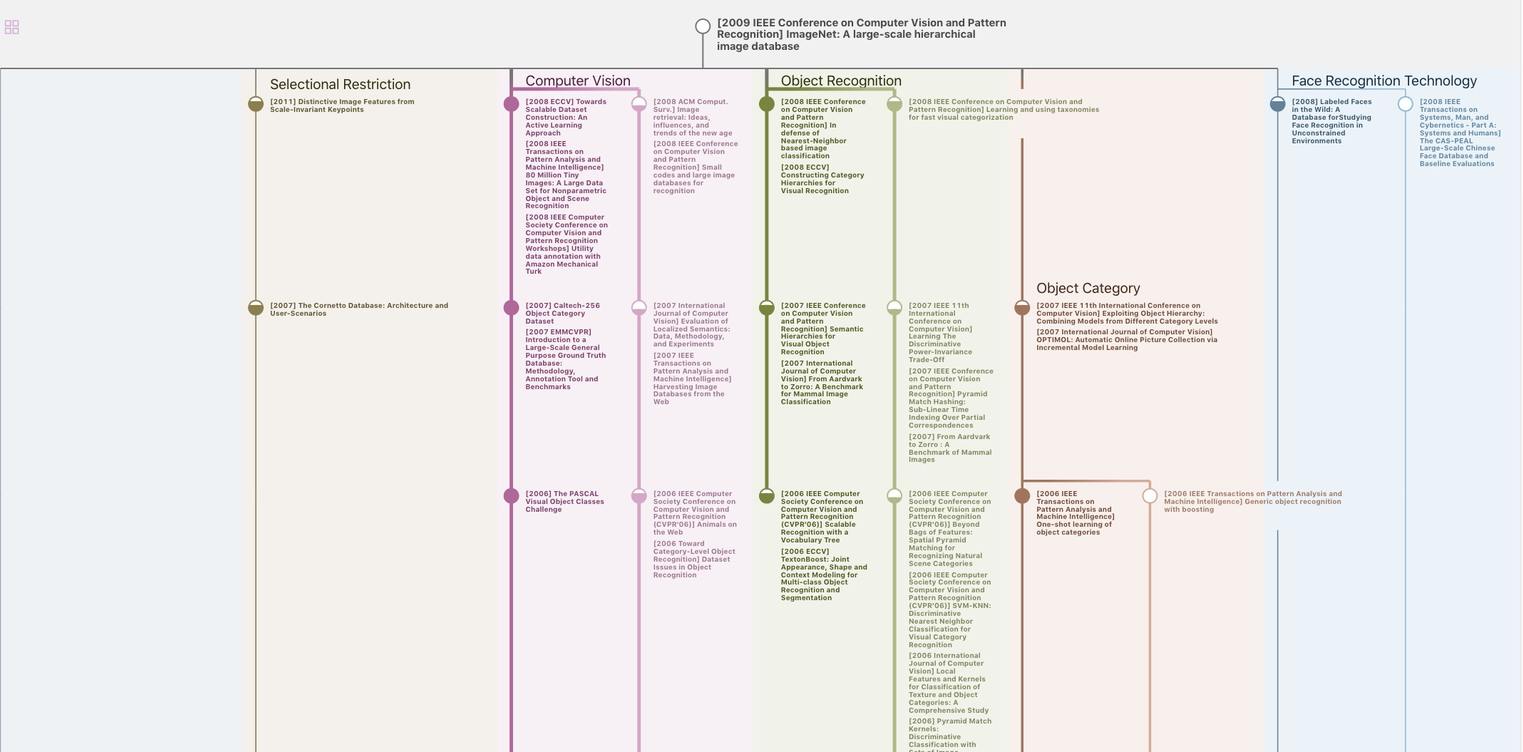
生成溯源树,研究论文发展脉络
Chat Paper
正在生成论文摘要