DeepChannel: Robust Multimodal Outdoor Channel Model Prediction in LTE Networks Using Deep Learning
IEEE ACCESS(2022)
摘要
Accurate channel model predictions are crucial in mobile communication systems to identify the coverage area of cellular base stations. It also allows network operators to optimally choose the locations of the new sites, solve coverage gap problems and optimize the current network parameters. Current prediction models use ray tracing techniques that are too computationally expensive and depend on the 3D maps, which are costly and need to be regularly updated. This paper proposes a multi-modal channel model prediction algorithm using satellite images to extract the environmental features and other numerical features. For an accurate evaluation, experimental measurements in the 2100 MHz band are gathered and combined with 2D maps from two different LTE network areas with varying characteristics to practically reference our results and compare the results with the ray-tracing output. Using the well-known AlexNet architecture as a baseline for our model and introducing new numerical features, we achieve a mean absolute error (MAE) of 2.06 dB and 2.6 root-mean-square error (RMSE) with 4.8 dB enhancement compared to only using numerical features. Using transfer learning, we train the model in area one and test it in another area. We achieve 1.47 dB MAE and 1.99 RMSE with 77.34 % reduction in the training time.
更多查看译文
关键词
Predictive models, Computational modeling, Feature extraction, Channel models, Long Term Evolution, Ray tracing, Deep learning, Channel model prediction, coverage estimation, LTE network, deep learning
AI 理解论文
溯源树
样例
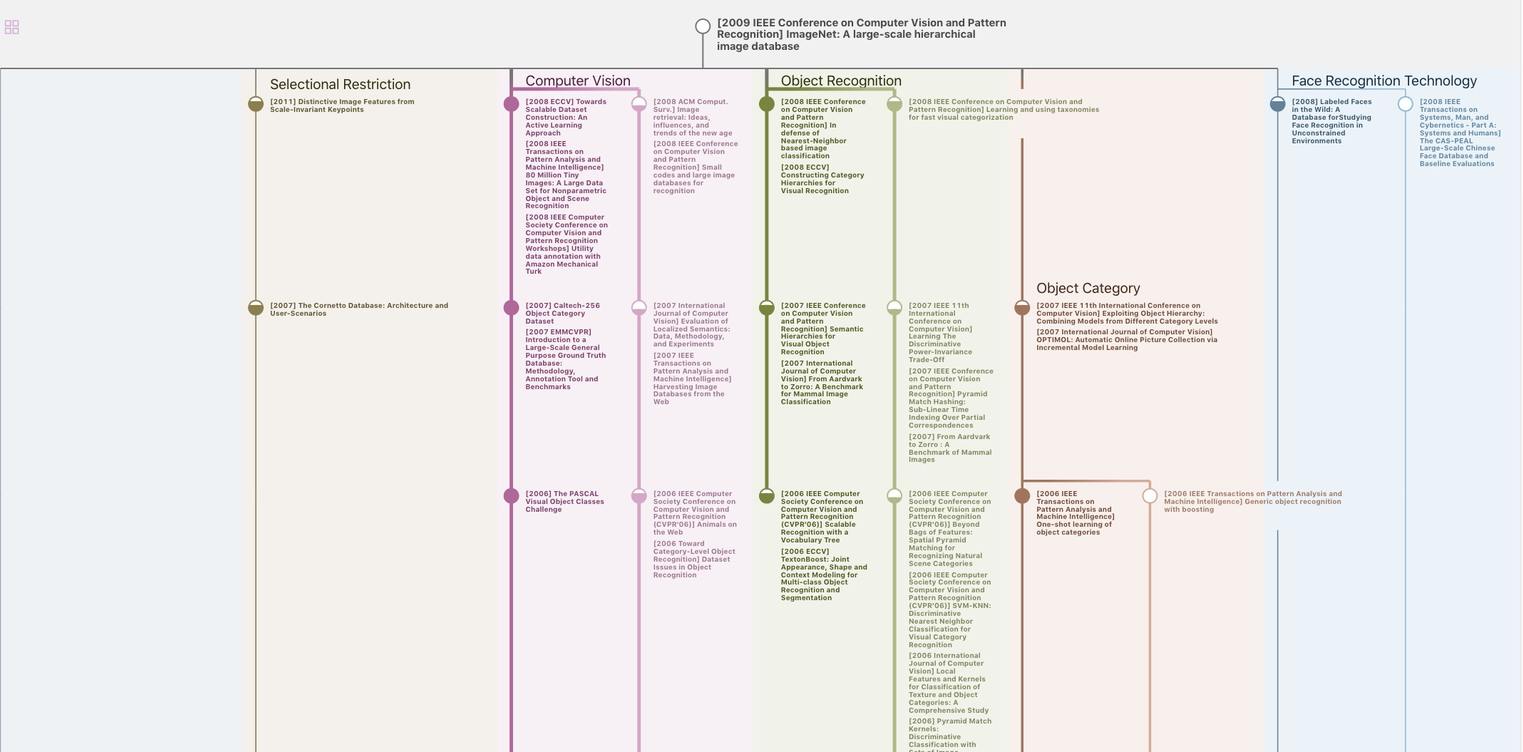
生成溯源树,研究论文发展脉络
Chat Paper
正在生成论文摘要